Blog
Discover how partnering with a software development agency can help maximise your R&D tax credits in the UK. Learn about upcoming legislative changes and tips for optimising your claim.
In the UK, the Research and Development (R&D) Tax Credit scheme is a valuable incentive for businesses innovating in science and technology. While many companies focus on their internal resources for R&D, leveraging a specialised software development agency can offer strategic advantages that maximise your claim. Here’s why partnering with an agency is often the smarter choice.
1. Expertise in Documenting R&D Activities
One of the most challenging aspects of claiming R&D tax credits is documenting qualifying activities. HMRC requires detailed technical narratives and precise cost breakdowns to validate your claim. A software development agency:
- Understands the R&D Criteria: Agencies often have experience in projects that inherently align with HMRC’s R&D guidelines, such as solving technological uncertainties or creating innovative software solutions.
- Provides Comprehensive Documentation: Agencies maintain meticulous records of time spent, technical challenges overcome, and methodologies employed, which can directly feed into your R&D claim.
This documentation not only ensures compliance but also reduces the risk of HMRC rejecting or questioning your claim.
2. Access to Specialised Skills and Resources
R&D often involves venturing into uncharted technical territory. A software development agency brings a diverse team of experts who specialise in cutting-edge technologies like artificial intelligence, blockchain, cloud computing, and more.
- Accelerated Innovation: Agencies have the infrastructure and expertise to quickly prototype, test, and iterate on ideas, ensuring faster progress on your R&D objectives.
- Cost Efficiency: Hiring and training in-house talent for a one-off R&D project can be costly and time-consuming. Agencies provide access to these skills on-demand, reducing overhead costs.
By outsourcing these efforts, you can maximise the technological challenges undertaken, directly enhancing your R&D tax credit claim.
3. Clearer Cost Segmentation
HMRC requires businesses to separate R&D-eligible expenses, such as employee salaries, materials, and software costs, from non-qualifying costs. This process can be intricate and time-consuming when handled internally.
A software development agency simplifies this by:
- Providing Transparent Billing: Agencies typically offer detailed invoices, categorising costs related to R&D activities.
- Streamlining Subcontractor Claims: If the agency is subcontracted, their costs can often be included in your R&D claim, subject to HMRC’s rules.
This clarity ensures that no eligible expenses are overlooked, maximising your claim.
4. Staying Up to Date with Legislative Changes
R&D tax relief legislation in the UK is constantly evolving, with significant changes being introduced in 2024. Staying informed and compliant with these updates can be a challenge for most businesses.
Software development agencies often have dedicated teams or partners specialising in R&D tax credits who:
- Monitor Regulatory Updates: Agencies stay ahead of the latest reforms, such as the new restrictions on overseas R&D and the merging of R&D schemes.
- Incorporate Changes into Processes: By aligning their operations with updated HMRC guidelines, agencies ensure that their clients’ claims remain compliant and optimised.
- Provide Strategic Advice: Agencies can guide businesses on how to adjust their R&D strategies to maximise benefits under the new rules.
This proactive approach ensures that your business is always leveraging the most up-to-date information and strategies.
5. Mitigating Risk and Uncertainty
Navigating the complexities of R&D tax credits can be daunting. Errors in your submission can lead to penalties, audits, or rejections. Agencies offer:
- Proven Processes: Agencies experienced in R&D projects understand how to align their workflows with HMRC’s criteria.
- Support During Audits: In the event of an HMRC inquiry, an agency can provide robust evidence and support to substantiate your claim.
By partnering with experts, you reduce the risk of non-compliance while ensuring that your claim is robust and defensible.
6. Focus on Your Core Business
Undertaking R&D while managing daily operations can stretch internal teams thin. By outsourcing to a software development agency, you can:
- Free Up Internal Resources: Allow your team to focus on core business objectives while the agency drives innovation.
- Benefit from Scalable Solutions: Agencies can scale resources up or down based on project requirements, giving you flexibility.
This collaboration ensures that your business remains agile and competitive without compromising on R&D ambitions.
7. How to Estimate Your Potential R&D Claim
If you’re wondering how much you might be able to claim, it’s wise to consult an experienced R&D tax credit advisor. Specialists like Walmer Group can help you assess your eligibility, estimate your claim amount, and guide you through the submission process. Working with an advisor ensures that your claim is maximised while remaining compliant with HMRC’s requirements.
Conclusion: A Strategic Advantage
Maximising R&D tax credits isn’t just about claiming back costs; it’s about leveraging those funds to drive further innovation and growth. A software development agency provides the expertise, resources, and transparency needed to optimise your claim while delivering cutting-edge solutions.
By partnering with a software development agency, you not only streamline the R&D process but also position your business for long-term success. It’s not just an investment in software—it’s an investment in your company’s future.
Looking for a partner to amplify your R&D efforts? At Blott Studio, we specialise in innovative software solutions that align with your business goals and help you maximise your R&D tax credits. Contact us today to explore how we can bring your ideas to life.
Learn the basics of cybersecurity and why they are critical in today's digital age. Explore how mastering the fundamentals supports compliance with DORA and strengthens your organisation's resilience against cyber threats.
As the digital world evolves, so do its challenges. Cybersecurity is no longer a mere technical consideration; it’s a strategic imperative for businesses. The introduction of frameworks like the Digital Operational Resilience Act (DORA) by the European Union has further emphasised the importance of building a strong cybersecurity foundation.
For individuals and organisations alike, especially those in sectors like fintech, securing digital ecosystems is essential to maintaining trust, protecting sensitive data, and ensuring compliance. This guide explores the basics of cybersecurity and why getting them right is more critical than ever.
What is DORA, and Why Does It Matter?
The Digital Operational Resilience Act (DORA) is a regulatory framework introduced by the EU to strengthen the operational resilience of financial entities. It requires firms to ensure they can withstand, respond to, and recover from cyberattacks and operational disruptions.
Key Pillars of DORA:
- Risk Management: Identifying and managing ICT (Information and Communication Technology) risks.
- Incident Reporting: Streamlining processes for reporting cyber incidents.
- Resilience Testing: Regularly testing systems to withstand cyber threats.
- Third-Party Oversight: Managing risks from outsourcing and ICT service providers.
- Information Sharing: Promoting the exchange of cybersecurity intelligence.
For fintech companies and related service providers, DORA underscores the importance of robust cybersecurity practices. Without the basics in place, complying with DORA's requirements becomes almost impossible.
The Basics of Cybersecurity: A Prerequisite for Resilience
Getting the basics right forms the foundation of digital operational resilience. Strong cybersecurity hygiene reduces vulnerabilities and lays the groundwork for more advanced resilience strategies.
Why the Basics Matter More Than Ever
The basics of cybersecurity, like password management and regular updates, might seem straightforward, but they are often neglected. According to studies, over 80% of breaches result from easily preventable issues, such as weak passwords or unpatched software. With DORA, these oversights can have regulatory consequences.
How the Basics Support DORA Compliance:
- Strong Password Policies and MFA: Essential for protecting access to sensitive systems, reducing the likelihood of breaches.
- Regular Software Updates: Prevent exploits targeting outdated systems, ensuring resilience against common vulnerabilities.
- Data Backups: Critical for quick recovery in the event of a ransomware attack or data corruption.
- Incident Awareness: Training staff to identify phishing attacks can significantly reduce the chances of incidents requiring DORA-mandated reporting.
- Network Security: A secure, encrypted network ensures that operational disruptions are minimised, supporting resilience.
Practical Steps to Strengthen Cybersecurity Basics
Here’s how organisations can align their cybersecurity practices with DORA’s requirements by focusing on the essentials:
1. Establish Strong Identity Controls
- Enforce multi-factor authentication (MFA) across all systems.
- Regularly audit access privileges to ensure only authorised personnel can access sensitive data.
2. Regular Updates and Patching
- Create a schedule for applying software and firmware updates.
- Use automated tools to identify and patch vulnerabilities in critical systems.
3. Build a Resilient Backup System
- Follow the 3-2-1 rule: Maintain three copies of data on two different media types, with one stored offsite.
- Test backups regularly to ensure they can be restored quickly in emergencies.
4. Train Employees Continuously
- Conduct regular cybersecurity training tailored to roles and responsibilities.
- Emphasise the importance of vigilance in spotting phishing attempts and other common threats.
5. Secure Your Supply Chain
- Vet third-party providers for adherence to security standards.
- Incorporate cybersecurity requirements into contracts with ICT vendors, aligning with DORA’s oversight provisions.
DORA: Raising the Stakes for Cybersecurity
Under DORA, failure to implement even basic cybersecurity measures could lead to:
- Operational Downtime: Unaddressed vulnerabilities can lead to disruptions, directly impacting business continuity.
- Regulatory Sanctions: Non-compliance with DORA can result in fines and reputational damage.
- Loss of Trust: Customers and stakeholders demand assurance that their data is safe and accessible.
The message is clear: if the basics aren’t done right, advanced resilience efforts, no matter how sophisticated, will crumble under pressure.
Looking Ahead: Cybersecurity as a Continuous Journey
Cybersecurity basics aren’t a one-time task—they are ongoing commitments. Frameworks like DORA aim to create a culture of continuous improvement and operational resilience, but that starts with mastering the fundamentals.
By focusing on strong passwords, regular updates, secure networks, and robust training, businesses can not only comply with regulations like DORA but also build trust with clients and partners.
At Blott Studio, we understand the importance of cybersecurity in the design and development of resilient digital solutions. If you’re looking to enhance your operational resilience or navigate frameworks like DORA, get in touch with our team today. Let’s build a future where innovation and security go hand in hand.
Discover the ultimate guide to fintech security. Explore key strategies to protect data, prevent fraud, and comply with regulations, ranked in order of importance to help your fintech thrive securely.
In the rapidly evolving fintech industry, where trust is the currency of success, security must be prioritised above all else. Handling sensitive financial data and facilitating transactions comes with significant responsibility, and fintech companies must establish strong safeguards to protect their users and operations.
Below, we outline the most important security considerations for fintechs, ranked in order of priority, to help ensure success in this high-stakes industry.
1. Regulatory Compliance: A Non-Negotiable Foundation
Fintechs must adhere to a complex framework of regulations. Compliance is essential not only to avoid penalties but also to build trust with customers. Laws such as GDPR, PCI DSS, and FCA regulations form the backbone of a secure and compliant fintech operation.
How to prioritise compliance:
- Conduct regular audits to ensure ongoing adherence to regulations.
- Stay updated on changes to industry laws, such as PSD2.
- Partner with compliance experts to navigate legal complexities.
2. Data Protection: Safeguarding Sensitive Information
Data breaches can have catastrophic consequences. Fintechs must secure all customer data at every stage, from storage to transit. By employing encryption, tokenisation, and data masking, sensitive information can be kept out of the wrong hands.
Best practices for data security:
- Use advanced encryption methods like AES-256.
- Replace sensitive data with tokens whenever possible.
- Mask customer data in logs, non-production environments, and UIs.
3. Identity and Access Management: Securing Access Points
Compromised credentials are one of the leading causes of breaches. A strong identity and access management (IAM) strategy ensures that only authorised users and systems can access sensitive data.
How to secure access:
- Implement multi-factor authentication (MFA) for all logins.
- Use role-based access controls to limit permissions.
- Adopt zero-trust principles, verifying all access requests.
4. Fraud Prevention: Staying Ahead of Threats
Fraud is a constant challenge for fintechs. With the help of artificial intelligence (AI) and real-time monitoring systems, companies can detect and prevent fraudulent activity before it escalates.
Steps to prevent fraud:
- Leverage AI to identify unusual patterns in transactions.
- Set up real-time alerts to flag suspicious activities.
- Strengthen user authentication with biometrics and behavioural analytics.
5. Secure Development Practices: Building Security from Day One
Security must be embedded in the development lifecycle. Following secure coding principles and addressing vulnerabilities early can significantly reduce risks.
Key development practices:
- Perform code reviews regularly to catch potential flaws.
- Use automated tools for static and dynamic code analysis.
- Follow the OWASP Top 10 recommendations to mitigate common threats.
6. API Security: Protecting the Digital Ecosystem
APIs form the backbone of fintech services. However, insecure APIs can expose sensitive data and open the door to cyberattacks. Fintechs must ensure all APIs are secure and resilient.
How to secure APIs:
- Authenticate API calls using OAuth 2.0 or JWT.
- Validate all inputs to prevent injection attacks.
- Implement rate limiting to control API usage and deter abuse.
7. Infrastructure Security: Fortifying the Foundation
Behind every fintech service is an infrastructure that needs to be safeguarded. Networks, servers, and cloud platforms must be hardened against external and internal threats.
Steps to protect infrastructure:
- Deploy firewalls and intrusion detection systems.
- Leverage cloud-native security tools from providers like AWS or Azure.
- Regularly test disaster recovery plans to ensure business continuity.
8. Third-Party Risk Management: Minimising External Vulnerabilities
Most fintechs rely on third-party vendors, which can introduce security risks. Proper due diligence and ongoing monitoring of these vendors can reduce exposure to vulnerabilities.
How to manage third-party risks:
- Evaluate vendors for compliance with industry standards.
- Clearly define data-sharing agreements.
- Regularly audit third-party systems and processes.
9. Incident Response: Planning for the Unexpected
Even the best defences can be breached. A robust incident response plan ensures fintechs can quickly recover and mitigate the impact of any security incidents.
Building an incident response plan:
- Develop a detailed, actionable plan for handling breaches.
- Monitor systems 24/7 for suspicious activities.
- Conduct post-incident reviews to identify lessons learned.
10. User Education: Strengthening the Human Firewall
Many security breaches occur due to human error. Educating users and employees about best practices can significantly reduce risks.
What to focus on:
- Teach users to identify phishing attempts and scams.
- Encourage strong passwords and secure account management.
- Design intuitive, user-friendly security features to reduce mistakes.
11. Penetration Testing: Identifying Weak Spots
Regular penetration testing helps uncover vulnerabilities before malicious actors can exploit them. Ethical hackers and security auditors can test fintech systems for weaknesses.
How to implement penetration testing:
- Schedule annual penetration tests with independent security experts.
- Launch a bug bounty programme to encourage vulnerability reporting.
- Use findings from tests to continually improve systems.
12. Privacy by Design: Building Trust Through Transparency
Privacy must be built into every system. This approach not only ensures compliance but also fosters user trust and loyalty.
How to prioritise privacy:
- Collect only the data you absolutely need for operations.
- Anonymise data wherever possible to protect user identities.
- Provide clear, accessible options for users to manage their privacy settings.
13. Emerging Threats: Preparing for Tomorrow’s Challenges
The threat landscape is constantly evolving. Fintechs must stay ahead by anticipating new risks, such as quantum computing and AI-driven attacks.
How to prepare:
- Begin transitioning to quantum-safe cryptography.
- Stay informed about AI tools used for cyberattacks.
- Train teams to recognise and respond to advanced social engineering attempts.
Conclusion
Security in fintech is not a destination but an ongoing journey. By addressing these considerations in order of importance, fintech companies can create secure systems that inspire trust and support long-term growth. In an industry where trust is everything, a robust security strategy isn’t just an advantage—it’s a necessity.
What’s that? Some call it the “eureka” moment. Some, the “wow” moment. But it’s all in the name; the “aha” moment is when a user thinks, “Aha! I get it.”
What’s that? Some call it the “eureka” moment. Some, the “wow” moment. But it’s all in the name; the “aha” moment is when a user thinks, “Aha! I get it.”
If you read my last blog post, you’ll know that first impressions matter. The first-time user experience is really important in long-term engagement. Why? Because users are exploring. They’re curious. And they probably have a problem to solve. With Saas competition on the rise, users might not stick around if they don’t find value quickly. So the sooner they feel that “aha,” the better.
Plus, a good first impression will keep your users coming back for more. Stats show that improvements in the first 5 minutes can drive a 50% increase in lifetime value.
How do we find it?
Your job is to find out which actions or behaviours led users to their “aha” moment. Then, adjust your product accordingly so that more users take that same path.

Step 1: Look For Patterns
Whether you have your own platform or use third-party tools, turn to your user data analytics. You want to look closely at what separates your “power users” from your visitors. Did they finish your onboarding experience? Did they continue using your app after the product tour? Did they engage with any of the core features? This will help you understand your product’s retention trends. Focus on those who stayed instead of those who didn’t.
For example, the behaviour exhibited by the most number of retained users is meaningless if it’s the same behaviour exhibited by the most number of churned users. But the behaviour exhibited by the most number of retained users, and only a few churned users? That’s something to look into.
Step 2: Ask Your Users
You’ve got your quantitative data. Now it’s time for qualitative data. Reach out to those “power users” for feedback. They know the ins and outs of your product. If the numbers have shown a correlation between certain behaviours and retention, these users can tell you why.
There’s no wrong way to get in touch. A survey, email or phone call will do. You’ll get information that the numbers can’t give. Why? Because people speak. They’re expressive. You’ll find out what features they used first, what other options they considered, and at what point your product became their go-to.
Don’t discount your churned users. You can learn from them too. If they didn’t find your product useful, you’d benefit from knowing why. Maybe they experienced some friction in the user experience. Maybe they prefer another platform (blasphemy!) So, ask them. Well, try. Chances are one-time users won’t be as giving as your loyal customers with their time, but a quick exit survey could do the trick.
For example… Asking a multiple choice and an open-ended question is a quick and easy way to get actionable feedback from churned customers.
Step 3: Experiment With New Users
You’ve identified patterns and you’ve listened to users. By now, you’ve got some idea what influences retention. This is called your hypotheses, and you’re going to test it. How? With new users.
You’ll reverse-engineer the journey of your “power users” and apply it to segmented newcomers. Yes, segmented. You need a “control group” who aren’t exposed to the changes. Use A/B testing to determine how variables impact retention.
If your hypothesis is proved? Great. You know where to drive your users. Once they get there, they’ll experience value and they’ll stay. So you’ve got a clear goal for your onboarding.
The goal?
There are many “aha” moments. And there are many factors that contribute to them. As your user-base grows, so will your understanding of what these factors are.
The best product leaders think about value from their user’s perspective. Make it easy for your users to find the value in your product. It isn’t always obvious. Experimentation, iteration and analysis is key to a better experience. For you, and your customers.
And remember, your users are the reason for your product. They have a problem. You have a solution. Make sure it solves their problem better than anybody else.
As much as we’d love to think that our users are a lot like us, they rarely are. That’s why it’s important that we understand who they are, what they need, and how they go about getting it. Our products are designed for them.
As much as we’d love to think that our users are a lot like us, they rarely are. That’s why it’s important that we understand who they are, what they need, and how they go about getting it. Our products are designed for them. So why not improve the experience?
User testing is a great way to do this. Gathering unbiased opinions will ultimately lead to a better user experience. Ask the right questions and you’ll get the right insights, ones that you can act on.
First things first…
You need to define your objectives. Figure out what it is you want to achieve, and why. Are you looking to prove a hypothesis? Find issues in your product? Understand how people interact with your competitors? A clear objective will help you create the right questions and get to where you need to be.
Essentially, your questions should produce a mix of qualitative and quantitative data. Think multiple choice, open-ended and the yes/no type. Throw in a practical exercise, and you’ve got yourself a recipe for success.
Screening Questions

Right. You know what you want. Now you’ve got to decide who to get it from. Who would be your ideal candidate; someone who’s just signed up? Or a power user? Get your demographic and experience questions out the way. They’ll help you find potential participants. Plus, you can use this information at a later stage in your analysis of the final results.
1. How old are you?
2. What’s your highest level of education?
3. How much time do you spend online?
4. What apps and websites do you use on a regular basis?
5. What’s your income level?
Pre-test Questions

Once you’ve selected your test subjects, you’ve got another opportunity to filter them. This will uncover whether or not participants know enough about your products to provide relevant feedback. Do this through a questionnaire, survey, face-to-face interview or any other way that works for you. Plus, knowing the backgrounds of your participants means you’ve got context for the way they interact with your product.
6. What made you choose this website/app?
7. How often do you use the website/app?
8. Which features do you use most?
9. What other websites/apps did you consider using?
Test Questions
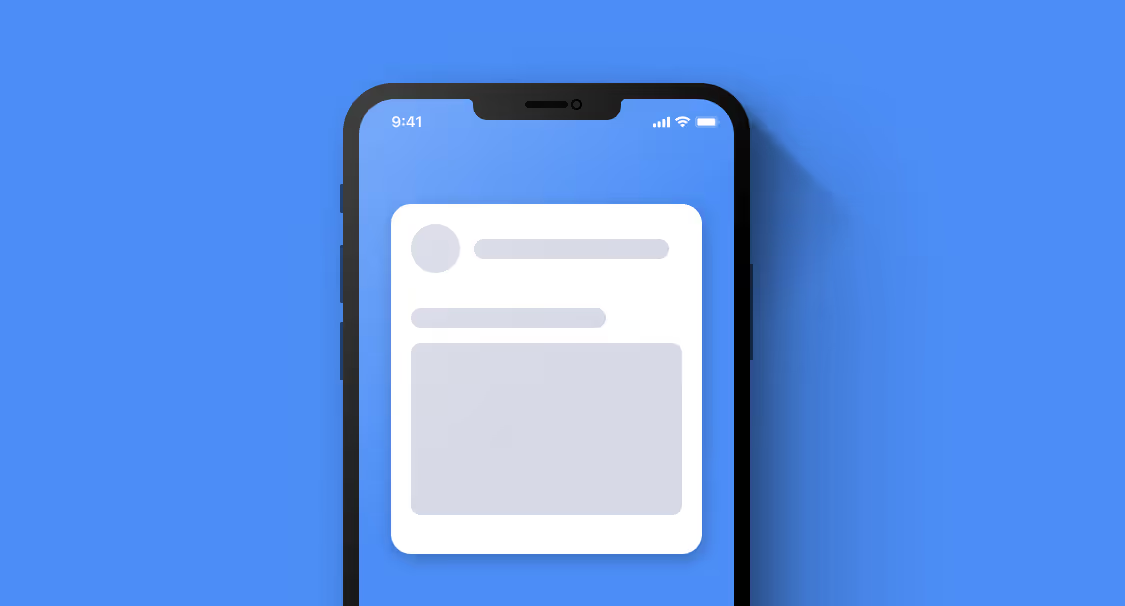
It’s time for the real deal - the test. During this stage of questions, your goal is to collect data that explains why users make certain choices while interacting with your product. It could be silent, with users completing tasks and then answering questions after. Or, it could be conversational, with users talking through their thought process at each step.
Our advice? Make it conversational. The more relaxed participants are, the more open they’ll be.
10. I noticed you did ___. Can you tell me why?
11. Is there another way to do that?
12. What stops you from completing a task?
13. How do you use the X feature?
14. What do you think of the interface?
15. What do you think of the design?
16. How do you feel about the way information is laid out?
17. What do you think of X page? How easy is it to find?
Post-test Questions

Missed anything? Not fully understanding certain answers? Now’s your chance. It’s also a great time to let your participants ask a few questions or offer feedback they believe is relevant. These questions can be more general to get an overall opinion on the user experience.
18. What’s your overall impression of X?
19. What’s the best and worst thing about X?
20. How would you change it?
21. How would you compare X to a competitor?
Note taking?

Forget about it. You won’t be able to focus on non-verbal cues if you’re furiously scribbling down minutes. It might also distract your participants or make them even more uncomfortable than they already are. Record your interview instead. Then, upload it to a transcription service. Like the speech-to-text software, Rev.
Constructive criticism?
It’s one of the necessary evils, especially in tech. The more usability tests you run, the more feedback you’ll get - good and bad. But that’s okay. Without different perspectives, you wouldn’t be able to see the bigger picture and then better your product. Plus, allowing your users to rip your work to shreds in the name of improvement often results in an even better user experience than anyone could have imagined.
Startups fail from time to time. Not because of a lack of good ideas, but because the wrong idea’s been chosen.
How to prioritize product features and improvements
Startups fail from time to time. Not because of a lack of good ideas, but because the wrong idea’s been chosen. Most product managers will agree that the hardest part is deciding which features deserve the team’s blood, sweat, and tears. Okay, we lied. There’s no blood.
.avif)
First things first.
Goal setting is a serious problem. Before you can even think about prioritising your features, you have to figure out what it is you’re working towards. Think, “shared vision.” Without it, you’ll go round in circles.
As Max McKeown, author of “The Innovation Book: How to Manage Ideas and Execution for Outstanding Results” writes:
“Strategy is not a solo sport, even if you’re the CEO.”
Prioritization? It’s not personal.
But… It can be. Humans are complex creatures. They have emotions, opinions and ideas. And when those ideas are shared with the team, they have to be acknowledged. Why? Because every idea is a reflection of someone’s hard work and experience. Fair enough. But it does make things complicated.
It’s important to remember that you’re not picking someone’s idea over someone else’s idea. You’re picking what’s right for the company. So, prioritization? It’s not personal. Fact.
Hippos and out-of-nowhere requests.
A bit confused? So is your team. The Highest Paid Person’s Opinion (HiPPO) has the power to veto certain ideas or insert personal choices without the necessary backing. Why? Because they’re usually in a senior position, so they’ve probably got good judgement.
This can be frustrating for the team though. Nobody likes it when high-level ideas are added to the list as “must-haves.” Decisions made or changed without evidence have the potential to derail even the most thought-out plans and leave team members feeling lost. Most leaders don’t want that. So, no evidence? No action.

What’s the opposite of subjective?
Objective. Correct. If personal bias can lead the team down the wrong road, then look at features through an impartial lens. Breaking them up into categories of criteria is a good way to do this.
- Feasibility. Can you do it? And by “you,” we actually mean the technical team. Speak to your front-end, back-end and UI designers to understand what can and can’t be done.
- Desirability. Do your customers need it? If you’re solving a pain point, then yes. That’s great. But if you’re not, then you’ll have to rethink your solution.
- Viability. Is your solution capable of succeeding long term? It needs to serve a bigger ecosystem. Will it keep up with the industrial times? Does it support your overall strategy? Hard to test, but worth it.
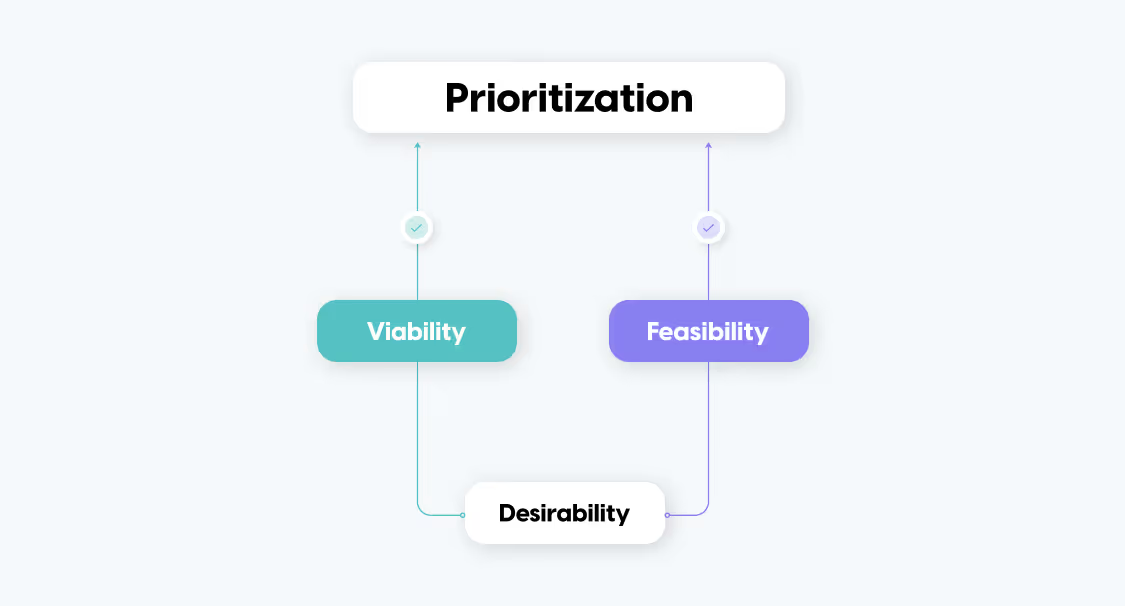
If you hear the word “gut instinct,” abort mission. This, along with customer requests and isolated economics are not ways to prioritise your strategic goals and activities.
Constraints are the new filters.
Time constraints. Money restrictions. Lack of expertise. They’re all important. If you don’t have the time, resources or skills for the job, you can’t get it done. Simple.
Constraints come down to two factors; people and processes. If you have the right people with the right skills to get the job done, then figure out which of those people will provide the best results. Someone who works well under pressure is better suited for time sensitive work. Are they more valuable than their peers? No. Not at all. The idea is to align certain personalities and working styles with certain outcomes.
And if you don’t have the right people with the right skills, outsource. Or hire. Conflicted? We touched on the topic in this article.
As for processes? Some teams use a home-grown approach, others a standard process. But a mix of the two is most common. The best processes are those that keep the team focused while allowing for the flexibility of everyday life.

Our advice?
Keep it real. There’s always a lot of hype around new features. They’re exciting! As a product manager, you need to be the voice of reason. Sorry.
Build a roadmap and add your priorities into it. This will keep the team on the same page and help you track your progress. Plus, we’re visual beings. We want to see what we’re working towards and how we’re going to get there. If you make data driven decisions, set aside time for regular check-ins and keep your roadmap front-of-mind, you’re in for a smooth ride.
Why are people using one feature three times more than another? Why are they falling off the wagon on the last step? Why are customers using your product less frequently than before?
Why are people using one feature three times more than another? Why are they falling off the wagon on the last step? Why are customers using your product less frequently than before?
Don’t you wish you could just… Ask them? Well, you can.
That’s what product feedback is all about.
Company lacking feedback?
You’re wrong.
It’s in your reviews, your support queries, your community forums... You just have to look for it. This is considered unsolicited feedback. It’s typically text-based and requires some effort to organise. It’s worth it though. And much like unsolicited advice, we don’t always like it. But in business, nothing is personal. Right?
There’s also solicited feedback. This means you ask your customers for it. How? You could send out a survey or hold interviews. You could host panel discussions and support groups. Or, you could even just have a rating option. There are many ways.
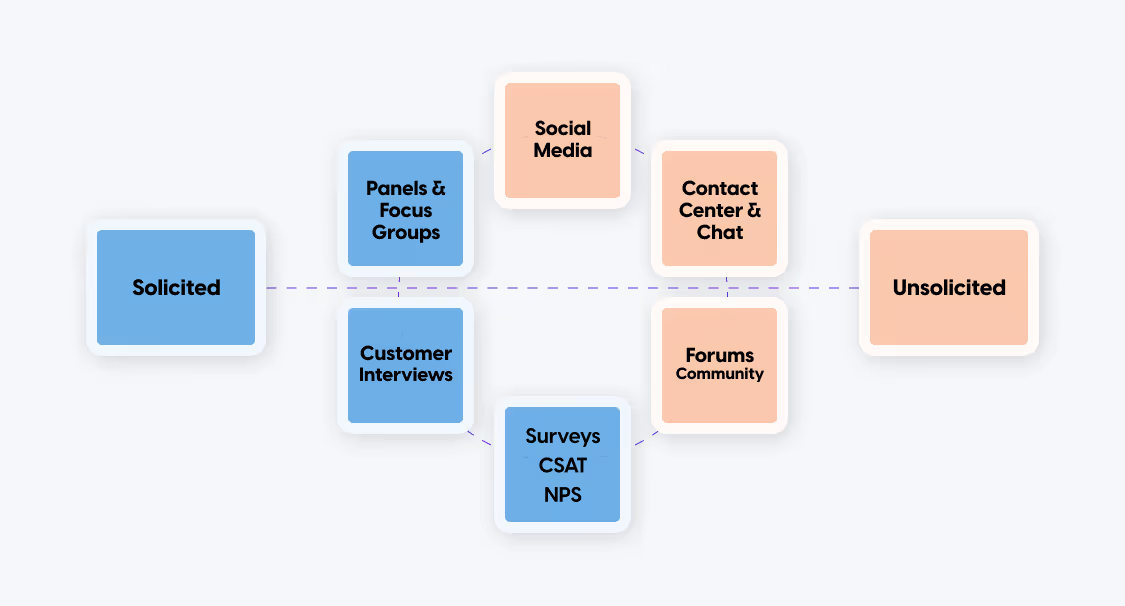
In this scenario, less is not more. You want to include both types of feedback from a variety of channels. This will help you get a well-rounded understanding of how your product is interpreted by users. Balance.
Feedback: Check. Now what?
Now, give it meaning. Few companies actually take action. Be one of them. By closing the loop, your customers know that they’ve been heard. Plus, you’ll set yourself apart from your competitors. Why? Because you’ve made it clear that you care.
Gather your feedback, turn it into insights, and send it off to your product team. They’re responsible for product decisions, right? So make sure your insights reach them. Get more feedback (and brownie points) by showing your users your new and improved product.

Need help with your feedback strategy? Here are 3 questions to ask yourself:
- Who needs to be involved in gathering feedback, and what role will they play?
- Think of churn, growth and other data. Which sets will you use to make decisions?
- What’s the best way to update your team about your progress, and your users about your improvements?
Analyze.
Easy? Nope. There are a lot of challenges when analyzing feedback. Seriously. If done manually, it can take a helluva long time. Also, each bit of feedback rarely fits into one category, if ever. Why? Because humans love to mention a bunch of different themes in their reports. Plus, visualizing this data is tricky.
Do we have advice? Of course.
Group your feedback into quantitative data.
Yes, even if it’s qualitative. If one user can’t find the checkout and another user can’t find the product, tag them both with an “improve search.” Take care not to overlap with your teammates though, a slight difference in linguistics could cause an unnecessary delay. And we don’t want that. So, no “poor search” and “improve search” tags. Pick one.
All feedback was (not) created equal.
What this means? You’ve got to link your feedback to demographic and behavioural customer data. Is this a new or old customer? How often do they use your product? How did you acquire them? Consider all aspects of who your customers are.
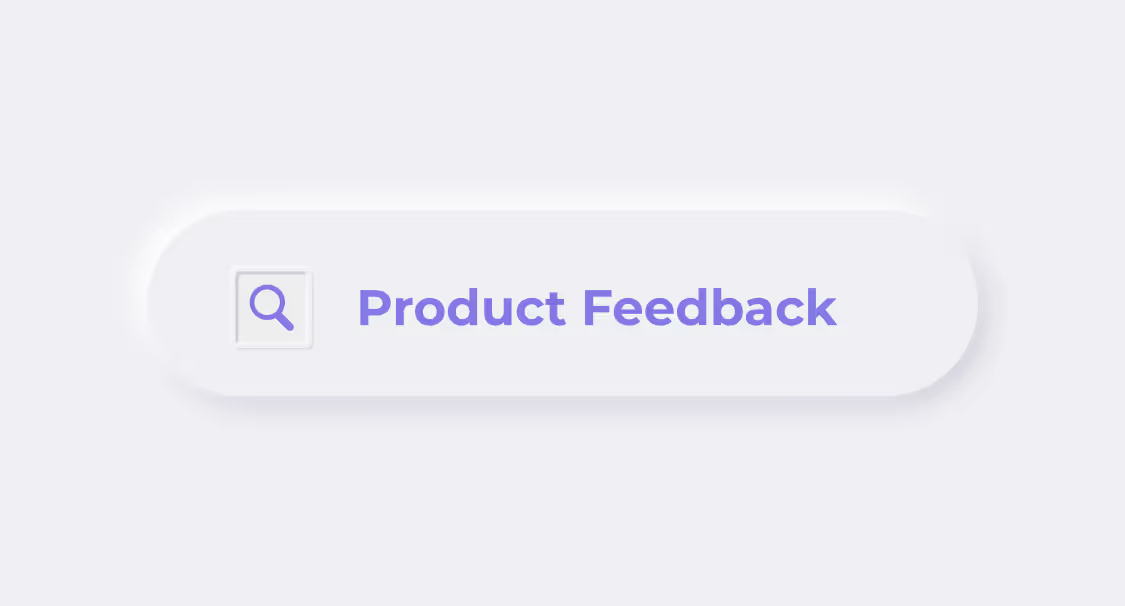
Who cares about feedback?
Honestly? Everyone. Feedback is a two-way street. You get feedback, then you return it... In the form of an update. It’s really valuable. Just as valuable as data. Why? Because it’s a form of data. When feedback is gathered, analyzed and actioned correctly, it works in the exact same way as data. True story. Testing hypotheses, discovering insights and aligning teams becomes easier with feedback. Sound familiar?
Give the people what they want.
You’ve got access to so much information about your users. Seriously. So there’s no excuse for not knowing what they like and dislike. It’s at your virtual fingertips. Take advantage of the data available and do something with it. Building a great product and marketing it well is only half the job. The other half? Committing to a customer-centric culture. Without your users, your company would be pointless. Be sure to find, analyze, share and implement feedback across your brand. Internal communication is key during your feedback journey, which should be a consistent and ongoing one.
Fun fact? Your support team always understands more about your customers’ needs than your product team. Always.
Brainstorming session, discovery group, team meeting; whatever. Every project should start with a group discussion- client included.
Brainstorming session, discovery group, team meeting; whatever. Every project should start with a group discussion- client included. Why? Because it’s important for your team to have an accurate idea of your client’s business, its goals, and a whole lot of other (related) insights that help with execution.
Usually, a discovery session takes place after a contract is signed, but it’s not uncommon for it to occur beforehand. That’s because not all services that software developers offer are suitable for those who enquire about them, and there’s no time to waste in the Saas world. Back and forth emails? Nope. No thank you.
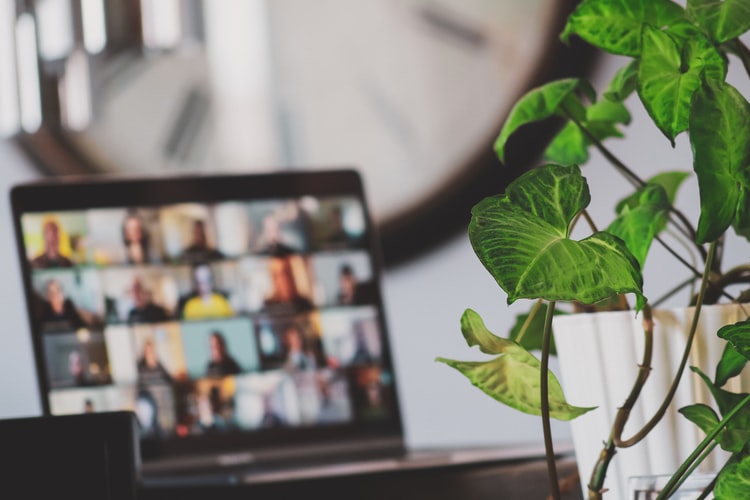
The point?
From identifying bottlenecks to creating a project roadmap, there are many outcomes of a successful discovery session. You can’t put a price tag on a project if you don’t know what services you’ll be exercising, so evaluating the state of your prospective client’s product is key.
Do this beforehand- it’s homework. Check out their company profile as well as every page of their website and app. Look at their services, competitors and ratings. Don’t forget to take note of your own first impressions, too. Looks promising? Great. Set a time.
Who’s involved?
If you’ve got a big and busy team, you probably don’t want to halt the progress on every project to meet with a maybe-client. Instead, have representatives from each department join the discussion. Your developer, UX designer, and project manager is important, but so is your strategist and facilitator.
Let the teammate with the highest level of understanding about your client’s business run the meeting. Why? Because they’ll encourage a deeper conversation. It’s generally a good idea to have this person translate the information into a strategic outline too- like a project plan.

The questions?
Need better answers? Easy. Ask better questions. Your clients may not know exactly what they want, so ask questions. A lot of them. Focusing on the questions, not the answers, will help with breakthrough insights and ultimately better align the both of you.
Start with constraint-related questions.
- Cost. What’s the budget?
- Scope. What’s the outcome? (New processes? Software? An upgrade?)
- Time. What’s the deadline?
Find out who the key stakeholders are.
- Sponsor. Who (or what) is providing support and resources?
- Customers. Who are the people who will pay for this?
- End-users. This one’s a no-brainer. Who’s going to use the product?
Set your intentions.
- Sprint duration. How long will you spend on each sprint?
- Tools. What software are you going to use to help you?
- Communication. How are you going to reach each other?

The interviews?
You’ve done your homework. Your maybe-client is now a yes-client. And you’re ready to talk business. So, chat (remotely) to the specialists on your client’s side. Why remotely? Because it’s easier, and you’ll have a far greater reach. If the specialists are too busy, an online form is a great compromise. This way, the specialists give you information in their own time. And you can focus on your other projects in the meanwhile. Win-win, right?
Need insights from the other side? Of course you do. Reach out to the end-users. How? With questionnaires or surveys. Don’t have end-users yet? Steal the target audience from your competitor. Go on, we won’t tell. Find out what the pain points are. A healthy combination of qualitative and quantitative is important, so A/B tests probably aren't the best way to go about this one.
Got your answers?
Great. Structure all of them, and move on to the following:
- What are the user’s pain points? Is there more than 1 type of user?
- Prioritize every issue. You can use the Decision Matrix to do this. It’s built on axes; “urgent” to “not urgent” and “important” to “not important.” The space with “urgent” and “important” gets first priority.
- Brainstorm a solution to every problem or opportunity. Your software developers should be involved in this one.
- Create a MVP. This is a low-fidelity prototype for a quick and easy representation of your product. Use it to improve the next version by getting quick feedback.
- Compare how the current product works versus how the future product will work.
- Create a roadmap.
What does this achieve? Your client gets a glimpse of the future product. And once you’ve got the go-ahead, you can start working towards it.

Our tips?
Communicate via Slack. It’s great for business-related instant messaging. Plus, it’s faster, better organised and more secure than email.
Delegate tasks with Trello. You can also manage, track and share your progress with your team.
Transcribe meetings with Otter. You get 600 minutes free per month, and it’s pretty accurate.
Record your meetings with QuickTime- video or audio.
The dictionary (well, Google) describes the term “Agile” as “able to move quickly and easily.” That’s true, even in the context of tech. But it’s also more than that.
The dictionary (well, Google) describes the term “Agile” as “able to move quickly and easily.” That’s true, even in the context of tech. But it’s also more than that. In the world of a software developer, “Agile” refers to a project management approach, and it’s a pretty common one.
The idea behind “Agile” development is that you build a framework nimble enough (agile enough) to adjust to changing demands. It’s probably one of the simplest yet most effective ways to turn a vision into something real. Plus, it’s quick. And there’s less risk. Instead of spending precious time and valuable money in the development phase of a product you have yet to find a market for, it gets broken up into the smallest, most basic version of your idea- the “minimum viable product.”
.avif)
This flexi-methodology emerged as a solution to the shortcomings of waterfall- a different (more expensive) way of finding a product-market fit. There’s only so many times you can afford to scrap and rebuild your product, and the time it takes to go through another product iteration in waterfall puts you at a disadvantage. Competition is rife in the Saas side of town.
It’s a continuous process, but don’t be fooled- Agile is anything but boring. The iterations are done in sprints, typically 4-6 weeks with a presentation to the client at the end. Then, repeat. Doing it this way replaces high-level design with frequent redesign. Plus, it allows you to test the application in real time, learn from your mistakes, and adjust the development strategy accordingly.
.avif)
The pros?
- There’s transparency between all parties, and at all points- users and clients included. Why? Because of the ongoing iterations. This makes having a shared vision easy, even in the face of unforeseen changes.
- Quality? Check. Breaking your project up into iterations means you’re able to focus on specifics. And because you’re constantly testing and iterating, your product gets updated and upgraded all the time.
- Early release. Remember that “minimum viable product?” It goes to market before the final product is ready. So identifying your product’s weaknesses and strengths becomes easy, and correcting them? Even easier.
- Costs are predictable. Because of the fixed sprints, the project can be well budgeted for. That’s the dream, right? And, because of the sprints, the project can also be frozen if necessary- something that’s not possible with waterfall.
- Changes can be made, and made often. Because your product is constantly evolving, you have ample opportunity to tweak things.
The cons?
- When do you stop improving your product? With Agile development, you don’t. Okay, you do. But it’s hard to. The desire to make endless changes can lead to a deadlock.
- Working in short-term iterations can hamper your long-term progress. And since it’s hard to tell what the end product is going to look like, it becomes difficult to predict the time, cost and effort it’s going to take to get there.
- Hard to maintain. Why? Because the level of collaboration is pretty high.
Even though the pros outweigh the cons, Agile isn’t for everyone. And if it isn’t for you, no worries. There are loads of different alternatives. Like waterfall, scrum or DevOps.

Waterfall
The Waterfall model is the earliest approach ever used in software development. And honestly? It makes sense. It’s the most logical. Seriously, the name is even sensible- progress flows towards the end goal, like a waterfall. So if you’re someone who likes structure, order and tick boxes, this traditional methodology’s for you.
Scrum
Not a fan of Waterfall? Take a look at Scrum. It’s similar to Agile in terms of the sprints and MVPs, but it’s not the same. Everyday you attend a brief meeting chaired by the “Scrum Master.” Fun, right? This is essentially to touch-base with your team. There’s also a product backlog, so a complete list of functionality yet to be added to the product. The best way to create a product backlog? Populate it with short descriptions from the perspective of your users. It helps if you do this on the first day of your sprints.
DevOps
Option 3? Merge two fields together- software development and information technology. Using this approach gets you a fast release. How? Through the use of automation, constant interaction, and a continuous workflow- also known as a DevOps process; a 7-part operation. Plus, efforts are made to get everyone familiar with each step. Not pros, but familiar.
Us? We’re Agile fans.
It’s a win-win. An Agile approach provides better opportunities for both the client and the agency. Deliberately learning from your mistakes allows you to tighten your feedback loop. And when you tighten your feedback loop, you speed up your product. The best part? Because of this to-and-fro cycle, you’re not just getting a flexible product, but a durable one too.
Need help with Agile Software Development? Check out this manifesto.
The internet has evolved from Web2.0 to Web3.0 - and this is not just a change in naming conventions. The underlying technology that powers the internet is changing, with blockchain being one of the key components of Web3.0's infrastructure.
Web3.0: The Next Era of the Internet
The internet has evolved from Web2.0 to Web3.0 - and this is not just a change in naming conventions. The underlying technology that powers the internet is changing, with blockchain being one of the key components of Web3.0's infrastructure. It will be decentralised, secure, self-sovereign, transparent, and scalable - all while being more cost-effective than its predecessor! Read on to find out how blockchain, Crypto and NFTs will be involved in Web 3.0 and what we can expect for the future.
So what are blockchain, crypto, and NFT's then?
Blockchain is a decentralised ledger that records all transactions of crypto - which includes but is not limited to cryptocurrencies like Bitcoin, Ethereum, and Litecoin. Crypto is essentially the fuel for blockchain as it requires an incentivising system for users to contribute their computing power towards maintaining the network (and thus decentralising it). The transaction fee paid in cryptocurrency also ensures security on the blockchain by making attacks costly.
Web technologies are powering Web2.0 with Javascript being one example - think about how many web pages you've seen spammed with ads or pop-ups! But what if there was another way? Well, this will be possible through the utilisation of new technology powered by NFTs, otherwise known as non fungible tokens. These differ from fungible tokens in the sense that one cannot alter the ownership signatures of one token to match another. This makes NFTs unique and thus valuable because they can not be copied or duplicated.
NFTs are being used in the creation of decentralized applications (dapps) which will power Web3.0. All dapps have their own cryptocurrencies powering them, these are known as utility tokens - think about how many times you've seen something advertised with 'Buy Now! Use Cryptocurrency!' The use of crypto techniques like smart contracts is also becoming increasingly popular in order to facilitate decentralised exchanges (DEXes). These enable people to buy/sell tokens without an intermediary; this has led some DEXes to gain huge amounts of volume.
.jpeg)
How does Web3.0 impact our everyday lives?
Firstly, by using a decentralised internet we can ensure that our personal data is not being used to make money from big businesses. We have seen this in recent times with Facebook and Google who have been criticised for collecting data on users without their consent. There are also implications for voting - an area where blockchain has already begun seeing success. In Estonia, anonymous votes were cast using a distributed ledger which ensured transparency - no more elections fraud! The coming years will see many people move towards web browser ‘add-ons’ which enhance privacy & security further while having little impact on performance e.g Brave Browser. Blockchain technology means it's possible to be your own bank i.e control your own funds directly rather than have them held in a regular bank account.
NFTs on the other hand will allow artists, musicians, and even regular users to license their digital assets as they see fit e.g artists can sell licenses for use of a photo - think Spotify but with photos, NFTs & Blockchain technology will allow people to monetise these digital assets and even own them! But how does ownership transfer work, how do we buy and sell NFTs? This is where exchanges come in, think of them as a marketplace for NFTs to be bought and sold. But what happens when you want to buy an item on the exchange? How do we know who owns it or how many tokens exist? This will all be tracked using blockchain technology e.g Cryptokitties uses Ethereum Blockchain.

What can we do to prepare for Web3.0?
We can start using cryptocurrencies like Bitcoin, Ethereum & Litecoin to buy NFTs. We need to be ready for when they become mainstream and want to spend them in the real world e.g paying with cryptokitties at Starbucks! There are already some places that accept these currencies as payment but expect this number to grow exponentially over the next few years (e.g CVS is now accepting it)
There's also a lot we can do on an individual level: I'm currently learning how to code so I will feel more confident about buying/selling items via exchanges or creating my own blockchain / dApp projects etc. If you're unsure of where and how to get started, youtube is always a great place to start - or if you're feeling really brave, buy just 10$ of crypto and have a foot in the game; by being invested, you'll naturally start to search for more info and resources. Get stuck in!
I think one of the most exciting things about Web3.0 is that it could be completely open-source - anyone will be able to contribute ideas or code which could really lead us into a new age of technology! I'm excited already just thinking about what we can do with this!
Cloud computing is continuing to take the digital world by storm. Businesses of all sizes are making the switch to Cloud-based systems, and for good reason.
Cloud computing is continuing to take the digital world by storm. Businesses of all sizes are making the switch to Cloud-based systems, and for good reason. Cloud computing offers a number of benefits, including resource scalability, resilience, and security. In this blog post, we'll take a closer look at why Cloud computing is the future of digital business, and how your business can make the switch, saving your business money.
AWS is the leading provider of Cloud computing services, and for good reason. AWS offers a comprehensive set of tools and services that can be used to build, deploy and scale applications in the Cloud. AWS also offers a variety of pricing options, making it easy to find a plan that fits your budget.
The first stage in the migration process is adoption, but it's also critical to consider how businesses may avoid AWS Cloud costs from escalating uncontrollably. Here are some essential tips on how to enhance your financial efficiency when it comes to the Cloud.

Top Tips for Reducing AWS Cloud Costs:
1. Use Reserved Instances: One of the best ways to reduce your AWS Cloud costs is to use Reserved Instances. With Reserved Instances, you can purchase compute capacity in advance, and save up to 75% on the cost of running those instances.
2. Budget AWS Cloud Services Carefully: Another way to keep AWS Cloud costs under control is to budget for AWS services carefully. When you know how much you need to spend on AWS each month, it’s easier to stay within your budget.
3. Remove Unused Resources: One of the biggest causes of AWS Cloud cost overruns is unused resources. Make sure you delete any resources that your business no longer needs, in order to avoid unnecessary expenses.
4. Identify and Consolidate Idle Resources: AWS Cloud resources may frequently be idle, resulting in unnecessary expenditure. In order to enhance financial efficiency, identify and consolidate inactive resources. The cloud provides autoscaling, load balancing, and on-demand capabilities that allow you to expand your computing power as needed.
5. Lower your data transfer costs: AWS offers a number of ways to lower your data transfer costs. For example, you can use AWS Snowball Edge devices to move large amounts of data into and out of AWS for free.
6. Use the Right AWS Services: Not all AWS services are created equal when it comes to cost. Right-sizing a workload involves re-assessing the true amount of storage and compute power that it needs. To determine this, you need to monitor workload demand over a period of time to determine the average and peak compute resource consumption.
7. Use Spot Instances: AWS also offers a Spot Instance pricing model, which allows you to purchase compute power at a discount. However, there is no guarantee that your desired instance will be available when you need it. Opportunities to buy Spot Instances can also disappear quickly. That means they are best suited for particular computing cases such as batch jobs and jobs that can be terminated quickly.
8. Choose The Right Worker Nodes: AWS offers a variety of worker nodes, each with its own cost. For example, AWS Fargate is a serverless compute engine for containers that removes the need to provision and manage servers. AWS Lambda is another serverless option that is suited for event-driven applications.
9. Use tools for infrastructure cost visualization: AWS provides a number of tools that can help you visualize your AWS infrastructure costs. These tools can help you identify areas where you may be able to save money.

In conclusion, the Cloud is a great way to reduce your business costs and improve financial efficiency. AWS provides a comprehensive set of tools and services that can be used to build, deploy and scale applications in the Cloud. AWS also offers a variety of pricing options, making it easy to find a plan that fits your budget. By following these tips, you can ensure that business is making use of AWS efficiently so that you can focus on what’s important - your business goals.
What are your thoughts on Cloud Computing? Have you already made the switch to AWS? Let us know in the comments below!
When designing new products, it's important to strike the right balance between conducting customer research and starting product development.
When designing new products, it's important to strike the right balance between conducting customer research and starting product development. Spending too little time on research can hamper your ability to discover real customer needs around which to design a successful business. In contrast, spending too much time on research can delay your product development iterations and deprive your team of precious learnings that could be gained from usability testing.
Signs that show you're doing too little research:
There are a few easy sense-checks you can do to determine if investing a few extra days on customer research is worthwhile. If one or more of these symptoms appears, go back through your previous interview notes or maybe schedule a few more customer interviews to drive that last bit of knowledge you need before moving forward. Here are some possible learning gaps that you should think about:
- Not knowing your target clients' journey from beginning to end: The first step in learning about your customer is to appreciate their entire trip. If you're still unsure about all of the stages your consumers should complete, how their feelings vary, who they're interacting with, and what technology they're currently using along the path, keep learning until you've drawn up a clear - and ideally visual - customer journey map. This may assist you in better defining where your solution should fit in the overall process.
- Not knowing how your target consumers are broken down: Personas can assist you in differentiating key aspects among diverse user types. You may better define exactly what target users you want to win by describing their activities, needs, behaviors, and desires. Make sure you make distinctions and similarities between subgroups of customers to properly identify whose needs your product is truly meeting.
- Not having a specific customer's voice in mind: You must capture the experience of a single, identifiable customer who you think epitomizes your target user and use that customer to rally your team. The power of specific user stories is immense and can be highly effective in pitching your venture to others. Building a new venture is tricky because the path is full of distractions and possibilities. The voice of the consumer can be your compass. Revisit your research until you’re able to pull out enough customer verbatims to ensure user-centricity among your team.
- Not knowing how customers would characterize and describe the problem: Consumers tend to have different mental models and concepts that they use to explain the same problem. To ensure that you optimize all your copy and marketing for customer conversions, you must speak consumers’ language. So avoid using jargon and complex constructions. Use consumers' own words wherever possible.
- Not understanding how your consumers view your competition: Before you design and build anything for your audience, there’s so much you can learn from what they think about products that already exist in the marketplace. So make sure you’ve had ample opportunity to ask consumers about what products they’ve seen, heard of, or used before - the competitors playing in the same arena. Ask consumers for their thoughts about those solutions - and why they did or did not enjoy using them. If they’ve never tried those products before, why not? What would change their mind about a product?
- Not being clear on how the problem you're solving compares to other pain areas in your customers' lives: It’s crucial that you grasp how the pain point you’re focusing on solving stacks up against your audience’s other relevant circumstances and issues. Do they care enough about this pain point to give you the time and money to solve it? Or is there a more important problem on which you could and should be focusing?
Signs that show you're doing too much research:
The more you research and learn about your customer base, the better it is said to help product design. However, in reality, spending too much time on customer research may lead to significant problems for your business. Delaying product-development timelines, allowing your competitors to gain a lead, losing focus, and becoming trapped in analysis paralysis are all possible consequences. The following are indications that you're ready to advance to the next stage of product development:
- Getting too caught up in user personas and customer journeys: the purpose of research is to advance clarity, not cause confusion. If you’re finding yourself knee-deep in data, trying to account for every permutation of your personas and their nuanced journeys, it’s time to stop and simplify. Your goal should be to capture just the right amount of complexity, so keep your personas simple and avoid getting too creative with your customer journeys. Generalize where possible and try to create models that your team can easily reference - models that can guide you onward.
- Hearing the same feedback continuously: If you're getting the same inputs from different, unconnected consumers over and over again, you've already found a pattern. It's probably time to move on if the next few customer conversations produce little new information.
- Taking too long to prototype: It is hard to put time limits on when you should move on to such work - mainly because the right answer depends on how much day-to-day capacity you have for pushing your ideas forward. Maybe you’re exploring ideas in your free time outside of work. But, if you’re working on your product full time, months have already passed, and you haven’t yet gotten around to building it, you should probably reevaluate your progress. Explore some ways to move forward with prototyping and testing your learnings and assumptions so far.
When prototyping and testing, you would be better off going for quick sprints that target particular assumptions or problems. Avoid getting stuck in analysis paralysis. Remember, customer research is a means to an end: building a minimum viable product (MVP) that you can test. It’s okay if you don’t know or fully understand everything about your customer base. In fact, your learning only deepens once you have something you can put in front of your customers.
In conclusion, customer identification should be as fine-grained as possible. Identify the various sorts of consumers that may be in a product category and what their pathways might look like. Understanding customers' decisions, inquiries, and low points allow you to make better judgments about who to develop your product or service for and what issues you want to address during their journeys. You should begin by striving for complexity so that you may notice little details.
However, you should then attempt to generalize until you can make broad-based decisions. There is no such thing as a single client journey, not even for one persona. Your goal should be to gather enough information to determine what critical, important learnings are required in order for you to establish a functioning model that allows you to take action.
There is a sweet spot for research in customer-insight development that balances complexity with simplicity. Being conscious of how much time and effort you should devote to research might help you achieve this objective and, as a result, produce better goods faster.
The pandemic has significantly accelerated the move to digital, forcing certain businesses to adjust. At the same time, buyers still want businesses to be simple to order from, engage with, and get service from.
The pandemic has significantly accelerated the move to digital, forcing certain businesses to adjust. At the same time, buyers still want businesses to be simple to order from, engage with, and get service from.
What is the difference between an exceptional customer experience and a good one? What are the steps you can take to ensure you provide your customers with both an excellent and pleasant encounter? Customer Experience (CX) needs redefining to be seen as more than simply a business practice. And in order to do so, businesses must change their perspective on CX through a comprehensive customer relationship management (CRM) strategy integrated into a modern CMS platform, with buy-in from all team members.
How have customer expectations changed, and which of these new behaviors are likely to endure?
Customers are curious as to why a business that provided a fantastic digital experience during the lockdown would not be able to maintain it in a post-pandemic world.
For today's customers, being happy is no longer an added value to their experience; it's the fundamental foundation on which your connection is founded. Consumers will pay more attention to a moment of friction than to a moment of flawless performance. Buyers are now more independent, expecting more intuitive experiences, and are stronger in their ability to change service if the experience does not fulfill their expectations.
Only those businesses that recognize their customers' requirements, wants and most importantly needs, focus on them first, and provide seamless, contextual experiences across all touch points will survive in today's extremely competitive market.
What's the best way for a business to offer a seamless customer experience in 2022?
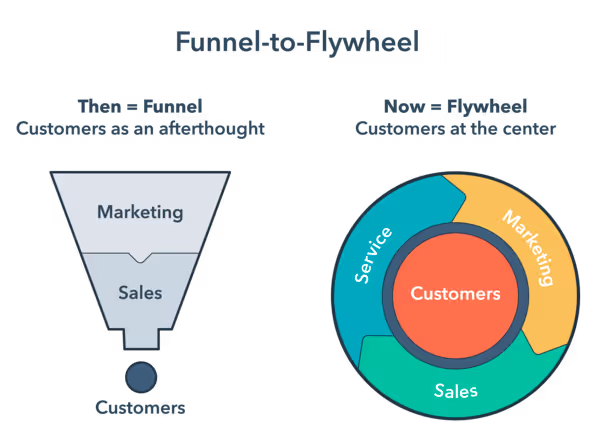
It's critical to unify marketing, sales, and customer service under one department, then create a decision-making group that owns the end-to-end client experience and has a winning aspiration centered on consumers rather than functions. This will allow any business to operate quicker while staying focused on its customers.
The funnel approach has previously dominated CX. Different functions focus only on fulfilling their part of the funnel before passing the customer on to be someone else’s problem once the sale is made. Instead, making use of an approach that puts the customer at the heart of a continuous process to attract, engage and delight customers will result in all functions having an ongoing responsibility to support one another in fulfilling goals for the overall business.
What is the role of technology and what obstacles should businesses overcome?
The 2020 survival mentality resulted in procedures and operations that were ill-suited for scale. As CX has become more complicated, most businesses have employed a patchwork of disparate technologies from various vendors, each with its own distinct underlying technology stack: a CRM to handle consumer data, a customer management system (CMS) to develop their website, and marketing automation to increase their activity.
When two completely distinct platforms are assembled together, the burden of employing them successfully is placed on the customer. This method is preventing businesses from flourishing, slowing them down, and depriving them of a complete perspective on their clients. It's also difficult to reconcile these differences without generating friction for customers.
Today's businesses need a strong and easy-to-use CRM solution that enables them to establish a "single point of consumer truth" that customer-facing staff can consume, allowing them to eliminate friction in client interactions and deliver delightful digital experiences in 2022.
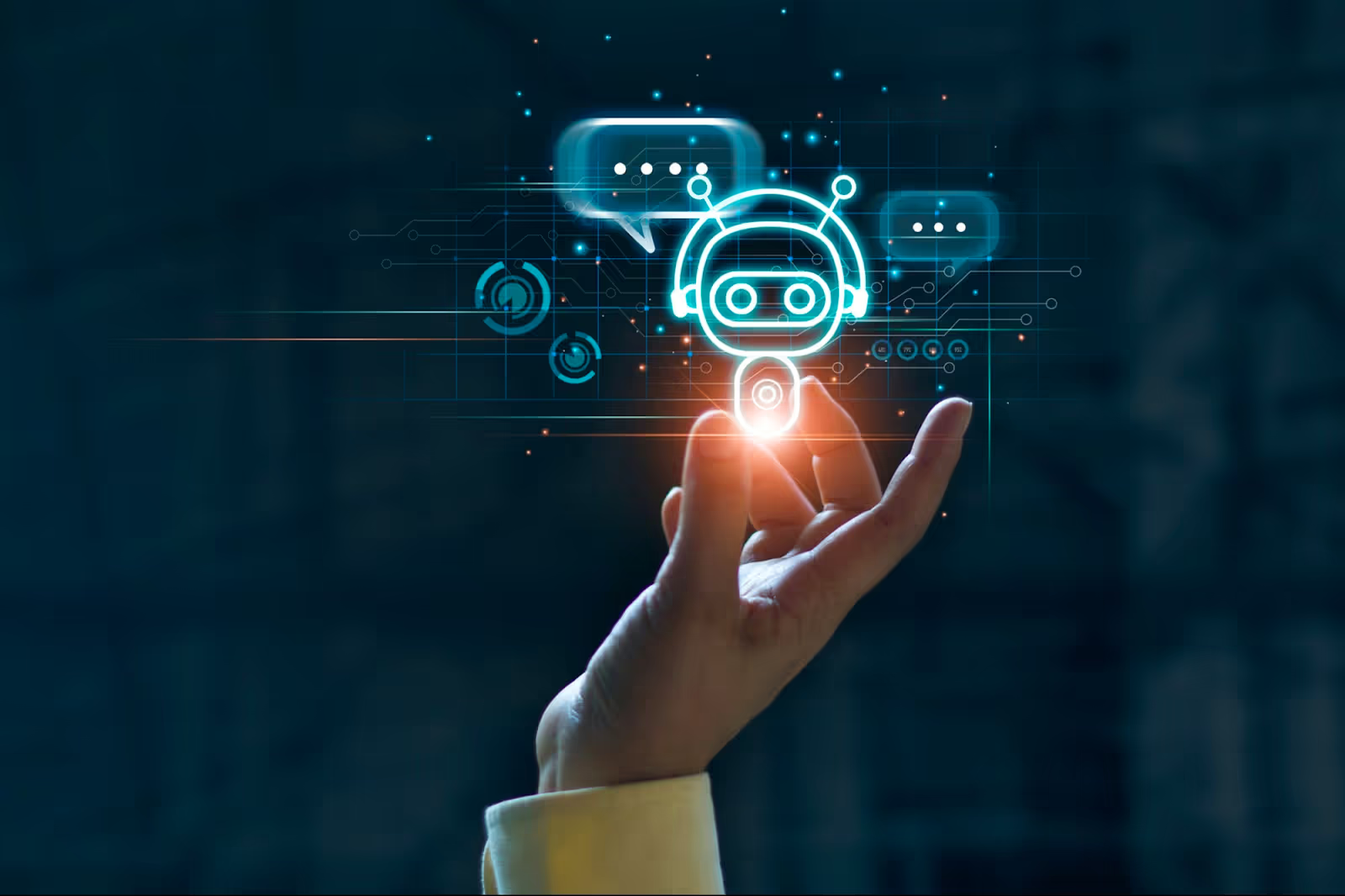
Where should businesses be concentrating their resources?
To get the digital experience right, good data is required: dependable, organized, and actionable data with insights into each customer's experience. ‘Who are your customers?’ isn't a useful question anymore. Instead, you need to be able to see the whole picture of this and other individuals in order for it to make sense. What does a customer's digital path look like? When, where, and how have they engaged with your business? What do they require and desire from you now, as well as what will they anticipate from you in the future?

It's also critical to guarantee that businesses provide all parts of the digital journey online. We've seen greater website traffic than ever before since the epidemic, and consumers will expect the same accessibility and convenience that they have come to expect from businesses over the last year.
Today, connecting what's going on on your website, the front door of your business, to the rest of the customer experience is a must. This may be a significant issue for businesses that don't use CRM systems. There will be businesses who have reached the maximum capacity of their present set-up and believe they are stuck. It's time for businesses to consider CMS as part of CRM in light of how closely linked customer experience is to websites today. The bar for customer experience has been raised; similarly, yours should be too.
It’s no secret that we all need to do our bit in reducing our energy consumption if we want to protect our planet, but the concept of "energy saving" is rather abstract.
It’s no secret that we all need to do our bit in reducing our energy consumption if we want to protect our planet, but the concept of "energy saving" is rather abstract. Knowing your exact carbon footprint and then tracking how much energy you have saved can give you a better idea of your contribution to a greener environment.
This is true for our personal lives, as well as business, and technology developments overlap in both. As the tech industry continues to develop and grow, so too, does this development impact our environment. The importance of energy-efficiency in all aspects of life is paramount, but how does this translate to mobile application development? What is best practice to paint your mobile app “green”, so-to-speak? Blott Studio along with Zevero, one of our clients for whom we have built a carbon footprinting management tool, takes a closer look.
Whilst software development isn’t the first thing that comes to mind when talking about carbon-intensive business operations, it is indeed responsible for a considerable amount of carbon emissions and it is therefore worth considering building a strategy to reduce those emissions. These emissions are attributed to building the operational app through its cloud providers (measured as emissions per gigabyte stored).
However, Zevero noted that the small amount of emissions produced through the creation of their platform was completely overshadowed by the tens of thousands of emissions their platform currently manages and the opportunities they have to scale reductions across entire industries. This is the impact of developing one “green” application.
With this in mind we have identified eight ways in which ourselves, and you, can reduce the carbon footprint associated with the development of applications:
One: Choosing the right hosting partner
By the year 2025 it is believed that data centres will account for 3.2% of all global carbon emissions (this is more than all airline emissions combined). It is therefore important to choose an eco-friendly hosting provider; one that makes use of renewable energy sources and/or has efficient data centres. A wide network of hosting partners is available for hosting services, so selecting a green partner is a great way to receive the technical support your application deserves, while still accounting for energy efficiency.
Two: Implement an efficient cache policy
It’s no stretch of the imagination to make the connection that caches use a lot of data, and thus a lot of energy resources. There are two ways to optimise your cache policy to ensure it operates efficiently. First, add support to all apps and APIs of If-Modified-Since header field with HTTP 304 Not Modified. Second, compress the data by making use of content delivery network services (CDN services). The new rule ensures that data will not be sent unnecessarily, while data compression reduces data usage.
Three: Optimise all support media
Re-evaluate all of your app’s resources to further minimise your carbon footprint. Start by clearing out unused metadata, media and images. Next, ensure all existing media and images are in vector or jpeg form. Alternatively, if media elements are non-vector, resize all items to the minimum-recommended size. Making these changes will get rid of excess weight and ensure that download times are optimised.
Four: Reduce data transferal
This tip is two-fold as it not only aids in your mission to reduce the carbon emissions of your application, but also improves the overall UX of your app by improving speed and reactivity. As previously mentioned, data is energy-intensive, so it is imperative to find ways to minimise data usage without compromising on UX. “Lazy loading” is the action of prioritising resources so that only those that are needed are downloaded for any given scenario.
Five: Declutter your app
Now, we’ve addressed the back-end by way of decluttering your app, but what about the front-end? Every feature added to an application adds more weight and increases the carbon emissions of the app. As per a report conducted by Standish Group, 45% of app features are used very little or not at all. Take the time to evaluate which features aren’t being used and remove them. The general rule of thumb is to reconsider keeping all features that are used by less than 5% of your users.
Six: Enforce energy efficiency
Mobile apps are known to drain a device’s battery, but these energy-intensive tools are also a burden on our carbon footprint. A good policy to implement is adapting the app’s behaviour based on the device’s power mode; effectively limiting background activity when the app is in low power mode. Examples of “background activity” could include location updates, Bluetooth and audio, amongst others. Battery life, speed, responsiveness and an “element of cool” all contribute to a great UX, as per the iOS App Energy Efficiency Guide, so your users will be sure to thank you for heeding this advice!
Seven: Optimise the use of location services
This rule is simple: don’t make use of location services when you don’t need them; location updates should only occur when absolutely necessary. It is completely understandable that developers require location information from time-to-time and to a certain degree of accuracy, but keep in mind that the more often this information is requested, and the more accurate the readings need to be, the higher the impact on your carbon emissions. Consider how regularly you truly require this information and to what degree of accuracy (will national rather than local suffice for your needs?).
Eight: Optimise the use of timers and notifications
Timers that run in the background and notifications that wake a device are all energy-intensive. Timers that are abandoned but not disabled further impact on energy usage. Consider if timers are the best solution for your mobile app, or if something like an application programming interface could work better. Our recommendation, on the topic of push notifications, is to make use of local and remote notifications. Local notifications are great for time-specific requirements, while the deferred delivery method for remote notifications ensures that the notifications are sent at a time that is most energy-efficient.
Our belief is that all mobile app developers should aim to produce green applications to the best degree possible. We’ve highlighted our guide to creating better-for-the-environment platforms that will not only help you to reduce your carbon footprint but optimise the UX at the same time. Selecting the right partners, implementing a few changes to the operational side of things and looking at simpler, cleaner displays all play a major role in mitigating the effects of carbon emissions on the planet. Blott Studio invites you to paint your mobile apps “green”!
Reach out to the Blott Studio team to further discuss best practices regarding energy-efficient mobile app development or find out how we can implement our tips throughout the design process of your mobile application.
The IT industry is surging as the innovation of technology continues to reach new heights.
The IT industry is surging as the innovation of technology continues to reach new heights. While this is great for those of us eager to download the latest app or communicate with our home appliances, the short straw in this industry boom is the lack of web developers looking for work. U.S. Labor Statistics found that, in December 2020, there was a shortfall in developers worldwide amassing 40 million roles and, worse than that, their forecast is set to reach double that number by the year 2030. Many companies are thus facing a similar conundrum: how to recruit a programmer.
The problem is that, in many cases, recruiters are bombarded with applications, only to find that many are underqualified. As a result of this, our instinct is to default to bias. Filtering applications according to schooling is a typical way we sift through CVs, but could we be overlooking quality programmers by doing this? How do you suss out the best talent and secure a candidate for your programming role? The answer lies in both the functional fit, or technical skills, as well as the cultural fit, or soft skills, of your candidates.
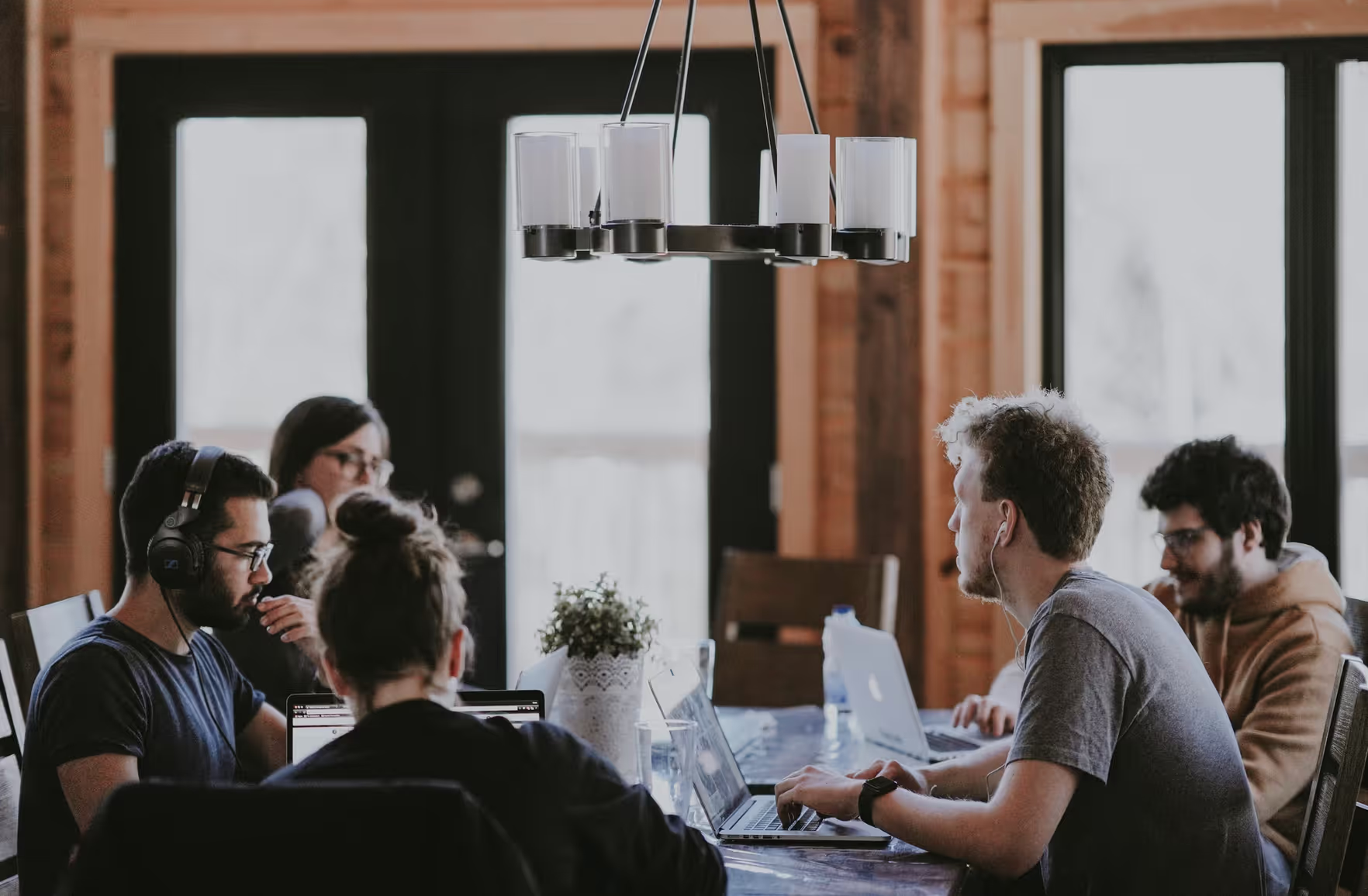
Functional Fit
Considering the functional fit of any applicant boils down to their hard skills and how those skills meet the requirements of the role of the programmer at your company. First and foremost, would be determining their qualifications. Now, there is debate regarding if programmers should have a degree or not. While the theory behind technical skills is important, some say that obtaining these skills via coding boot camps, rather than traditional degrees, is sufficient. A 2017 study by Indeed, where they collected survey answers from 1000 HR managers, found that 72% of employers felt that coding boot camp graduates were “just as prepared” as computer science degree holders, while 14% felt that their qualifications were lacking; leaving 12% thinking that coding boot camps provided more in the way of technical skills than traditional degrees. This same study found that 80% of tech managers had hired coding boot camp graduates, and 99.8% of these managers claimed that they would hire graduates from similar programmes, again. The decision then, on the debate circling “degree or no degree”, rests with you as the recruiter.
Regardless of the form of theory studies supporting your applicants, it is important to nail down their technical suitability throughout the interview process. Here are some ways to do just that:
1. Coding Project
This approach to evaluating a candidate’s skill set is used across several industries and is a great way to receive more than just their CV at the start of the interview process. Consider providing interested applicants with homework, which they would then be required to complete as part of their application submission. Examples of tasks could range from editing existing code to creating an application with a solutions stack (you may decide to provide parameters or allow the candidate free rein on the project). The coding project you prescribe should relate to the day-to-day work conducted within the company itself, so that you may get an idea as to how the applicant will solve your real-world problems, should their application prove successful. The only drawback of this technique is that these projects require manual review, which makes for a time-consuming process.
2. Coding Interview
A coding interview is a process of using a series of questions to test the applicant’s technical know-how. Programmer recruitment agencies typically make use of two platforms to do this: Hackerrank and Codility. The great thing about these platforms is that the evaluation of the applicants’ answers is conducted via an automated system, so you do not need to spend time manually scoring work. These questionnaires typically include a combination of open or closed questions, in addition to algorithmic tasks. The algorithmic tasks are formulated as problem statements, which the applicants need to solve. While the upside to coding interviews is the ease of processing, it is important to bear in mind the downside: the tasks may not align with the type of work your company aims to fulfil with this role, and you may need to spend additional time developing this interview format to meet your needs.
3. “Whiteboard” Interview
During the 1980s computers were expensive and difficult to transport due to their size, thus impractical for assessing a computer sciences candidate. Instead, pen and paper were used, and an interviewer would dictate problems or tasks for the applicant to answer. When the whiteboard was later introduced in the 1990s, it became all the rage and so they replaced the pen and paper for these interviews. Whiteboards made it possible for the interviewer to view the problem-solving techniques in real-time and introduced a way for them to engage the interviewee to better gauge their soft skills, too. Still termed “whiteboard” interviews, the whiteboard has since been replaced with virtual alternatives. While this approach offers a great insight into the technical and soft skill set of an individual, it is seen as outdated due to its disconnect from Stack Overflow and other tools currently used “on the job”.

Cultural Fit
A company’s culture can be described as those behaviours and morals which underlie the operations within an organisation and define how those operations are conducted within the company’s work environment. With that in mind, it is important to evaluate if an applicant’s behaviours and morals match those of the company and this can be done by looking at soft skills. While we won’t go into the details of each of the 15 examples of soft skills employees should possess, it is important to note how the person who takes on the role within your company should engage and interact with other members of your team. Some applicants may be experts at coding, but this doesn’t necessarily mean that they will get along with others or have a good balance of soft skills. Take the time to analyse how the programmer: communicates, works within a team setting, solves problems at hand, and manages stress in the work environment. These soft skills are very important to ensure your company finds a programmer who mimics the company’s culture. The process of analysing an applicant’s soft skills is best done during the screening phase and again after the technical aspect interviews, by Management or Human Resources.
It is important to remember that your company will most likely need to combine several of the above-mentioned strategies to properly assess the top candidates for the available programming role. While technical skills get the work done, soft skills ensure that your chosen candidate gels well with the team. Both are integral to the hiring process and, with the correct interview tools, will be able to highlight how the top candidates think and act in a workplace simulation.
Chat to the Blott Studio team today to connect with developers or discuss how your company can go about finding the right addition to your internal team.
POV: You’re planning to create your very own fintech app and have spent hours poring over articles relating to the start-up space.
POV: You’re planning to create your very own fintech app and have spent hours poring over articles relating to the start-up space. You’re aware that approximately 90% of apps fail (it’s the figure echoed across multiple industry-related blogs, after all) and you’re simply planning the best possible strategy to prevent a failure. But what is the secret recipe to ensuring a successful fintech product? How can you guarantee brand loyalty for an untested (and more than likely, undeveloped) fintech app? Here’s how the Blott Studio team believes you can formulate your fintech product for the win.
To understand the potential of fintech apps, we first recommend taking a glimpse into the social media powerhouse app of the hour: TikTok. As of September of 2021, TikTok reported 1 billion active users – a 45% growth over the previous year. They’ve also claimed titles such as the most downloaded, and most engaging, app. Parent company “by dance”, however, has even bigger plans for their app as they aim to build on the success of TikTok by turning it into a super app. That is an application that offers a range of services and allows integrations for mini-programs with select partners. An evolution such as this would mean a greater chance of success for the new feature programs (due to an already captive audience), as well as limit the user’s desire to use multiple apps rather than simply use the multiple services available on the existing product. By way of example, there have been whisperings that TikTok may be planning to launch a fintech product to their users in the near future.
But how can a social media application, such as TikTok, expand into the fintech space (amongst other potential industries)? It’s quite simple really: the key lies in the formula: CX = ML + UX. TikTok is already in the business of collecting data; they have a plethora of data points available on any given consumer of their product. These data points range from demographics used to maintain a user’s profile through to a user’s interests, which are tracked to recommend content. Now that they have this data, they simply need to employ machine learning to catalogue the information and then connect the organised algorithms to a top-quality user experience. This organised data can now be deployed across a range of products, even to the point of personalisation for each unique consumer. And, as the studies suggest, consumers are more interested in these “super” apps, even in the fintech space! So, let’s delve into the elements of that formula, shall we?
Part 01: Customer Experience
The bottom line in a successful app is customer experience; if consumers don’t have a need for the product, or find the product difficult to use, they’ll either not download the app in the first place or delete the app in frustration. It’s up to your team to balance the technical complexity with its useability; that is, determining the best combination of features to satiate the user while ensuring that navigating and engaging with the app remains easy and rewarding. Customer experience is their impression of every phase of the journey they undertake when purchasing your product. The first thing to consider on your climb to success is the need your fintech product serves consumers. Now, many companies get this wrong, because they tend to focus on which customers fit into their available product portfolio, rather than viewing the needs of the consumer (irrespective of that portfolio). Consumers want to feel valued, and they’re more likely to make use of fintech apps that have taken a beat to listen to their hearts’ desires. Once that need has been determined, you’ll want to turn your attention to the aforementioned formula, which states that customer experience is equal to machine learning plus user experience. Let’s take a look at “the other side” of formulating your fintech product for the win.
Part 02: Machine Learning
Now, we’ve touched on the importance of changing the focus from your product to the consumer’s needs. The idea is that your product should pivot to serving this identified need. The second phase of discovery lies in developing a machine learning algorithm that will analyse and organise the raw data you have access to so that you can step in and evaluate your consumer base with ease. (Think predictive banking analytics and propensity models.) The third, and final phase of discovery, is next-best-action systems. Monique Duquette of SAS Canada defines NBA’s as, “a customer-centric marketing technique that considers the alternative actions during a customer interaction and recommends the best one.” Combining this approach with machine learning algorithms allows you to target unique consumers with personalised offers. It is this level of personalisation that your consumers will appreciate, and that will ultimately turn them into loyal users.
Next-best-action systems are an important part of the algorithms your app employs for the following reasons:
- They analyse individual consumers’ spending and buying behaviour, record location, and even keep track of how this consumer responded to previous campaigns. This information provides you with the opportunity to push personalised offers to individual consumers, which leads to higher conversion probabilities.
- Further to point one, these predictive analysis tools can make recommendations on which products individual consumers will most likely need next. This aids in customer experience.
- These systems also allow for in-depth tracking, so that your team can keep abreast of previous campaigns offered to individual consumers to avoid marketing the same product repeatedly.
Fintech start-ups can maximise the impact of machine learning (and how it influences customer experience) by making use of conversion AI interfaces to summarise those personalisation’s to meet the needs of the individual efficiently.
Part 03: User Experience
It has most recently been purported that millennials are getting better at saving and managing their money habits. In fact, Bank of America suggests that there has been a 16% increase in saving by millennials from 2018 to 2019. They’ve also noted, however, that approximately 75% of millennials in the USA have debt. With these statistics in mind, let’s take a look at the three elements that will assist you in developing a quality, user experience focused, fintech product:
- Educating the user. We’re specifically speaking to intuitive and progressive learning metrics in developing your fintech product. It’s important to aid the user in discovering the abilities of your app, in a way that is easy to follow. Think of creating a funnel that follows the user’s journey from beginner to expert.
- Security. Fintech carries the burden of offering a secure product. There is also a fine balance between ensuring that consumers can use your app safely and making sure that the security of said app is sufficient to protect that user, without creating frustration for that user. Consumers need to be able to trust that their information and finances are protected while having relatively easy access to manage their app. Biometrics and two-factor authentication are great security tools that can be used at different levels of the app.
- Authorisation. Just as you wish to create a smooth, secure experience for your existing users, so too should the onboarding process of new users be relatively easy. Authorising new accounts is the first glimpse users have at understanding the intricacies of your fintech product and, if the process is over-complicated, they’ll more likely be scared off. Consider keeping the process simple for access to the basic features of your product; additional security measures can always be utilised for features of a more sensitive nature.
- Friction. Points two and three discuss security systems for your app and, while your aim should be to offer a smooth user experience, you also want to ensure that mistakes are non-existent. This is where the term “friction” comes in. In essence, friction refers to protecting the user from making mistakes and will involve pop-up confirmations, two-factor authentication, and review steps to avoid users accidentally sending their earnings to the wrong place and the like.
- Display. Finances are stressful, and a large majority of mobile app users don’t have degrees in financial services or related fields. So, keep the front-end of your app easy to navigate and understand. For example, if your fintech app helps users track their spending, ensure that the key indicators are displayed at a glance. Navigation to in-depth metrics can always be included, but could overwhelm the average user and should thus be incorporated as a separate dashboard. The interface should be seamless and effortless.
Bringing the Parts Together to Create the “Whole”
As you work on your fintech product, keep the formula CX = ML + UX, in mind. While it presents as a three-part formula, there are actually six layers to those three parts that need to be considered to take your app to the next level. Raw data and analytics work hand-in-hand as the first two layers. They involve collecting and organising consumer data to understand the individual and prepare the most successful strategy to deploy for each. This is then where marketing operations take place, as the marketing function makes use of the analytical information to target individual consumers with a personalised experience. On acceptance of this personalised offer, the user is then digitally onboarded. The fifth layer is interaction and involves input from your analytical layer that monitors the individual so that you can set push notifications that are of value to that specific user. The final layer is that of customer service. This function involves resources available to the user, a potential chatbot for self-help, as well as your support team.
In summation
This modus operandi is the blueprint for formulating your fintech product for the win. A quality customer experience is what sets Fintech apart from traditional banks and employing the elements of machine learning and an excellent user experience is what will get you there. The key lies in maintaining an intuitive product that is perceived as simple in the eye of the consumer, irrespective of how many features your fintech product expands on to include. If your users feel valued, and believe that their app is personalised to their needs, your product will remain their primary financial product.
Loved this blog? Read next: Top Tips for a Market-Ready Fintech Product
Ready to bring your fintech product to fruition? Schedule a chat with the Blott Studio team.
Facebook, Instagram, Shopify, and Uber are among the many businesses that have developed their applications using React Native.
Facebook, Instagram, Shopify, and Uber are among the many businesses that have developed their applications using React Native. In fact, it’s become one of the top frameworks used by developers across a range of industries. But is it the right fit for your product? Do you know enough about the benefits as well as the drawbacks of React Native? Have you connected with a developer that can facilitate your React Native journey? In this guide, we’ll take a look at everything you need to know about React Native to make the best decision for your app.
So, to start, what exactly is React Native?
React Native is a framework based on JavaScript that is specifically used to build natively rendered mobile applications on both Android as well as iOS. It combines JavaScript coding with native platform capabilities to empower developers to craft products efficiently and effectively. The great thing about React Native is that the same codebase can be applied to various platforms. It is due to this capability that the framework has quickly become a favourite amongst developers. React Native saves valuable time and energy with its “one size fits all” approach, is based in React (a popular JavaScript library), and provides developers with the ability to create fully-fledged mobile applications; all contribute to its success and favourability.
React Native’s origin story
In 2012 Facebook decided to shift their focus from web-first to mobile-first. The problem, that stuck out like a sore thumb in the face of this company shift, was that the majority of their development team were web trained. This resulted in a few clashes, attempting to use HTML5 and WebView, and the Facebook team soon realised that none of these options were resulting in a mobile product they loved. Their aim was to provide the best possible user experience and the natural choice would have been a native application, though this presented its own set of challenges. With this in mind, Christopher Chedeau teamed up with Jordan Walke, Ashwin Bharambe and Lin He to create a prototype that enabled them to generate native UI elements from JavaScript threads from the user’s device directly. Armed with this breakthrough, Facebook organised an internal hackathon which resulted in a prototype that the team felt could form the basis of a mobile application framework. The original team took the discoveries from the hackathon and built out the React Native technology, which was open-sourced at the React.js Conference in January of 2015. Initially, the framework could only be used for iOS application development, but in September of the same year, the Android compatibility update was released. The Facebook team didn’t stop there and announced Windows and Tizen platform support in 2016. Fast forward to 2022 and React Native is as popular as ever with approximately 50 000 contributors on Stack Overflow.
React.js vs React Native
Now, you’ve heard us use the terms “React Native” and “React.js”, so what is the difference? The React framework was also created by Facebook, but it was created to enable their developers to craft front-end user interfaces based on UI components for their website. It is an open-source JavaScript library that gives developers the ability to write sophisticated front-ends for an enhanced user experience on websites. React Native was developed later to solve the crisis of mobile use for Facebook by “mirroring” the UI qualities developers love about React.js. Furthermore, React Native is powered by the React framework.
How does React Native work?
React Native makes use of JavaScript and JXL as its write-up programming languages, which means that it can communicate with both JavaScript-based, as well as native app threads. This communication feature is written into existence thanks to React Native’s “bridge” function that forms a link between the two unique languages (much like a bridge connects two land masses). The great advantage of this functionality is that developers can make use of components within existing iOS or Android mobile applications or make the switch to React Native. This effectively makes React Native a cross-platform development solution.
The advantages of React Native
One of the biggest reasons for React Native’s success is that it operates on actual native views and components rather than rendering in WebView like many other cross-platform development frameworks. This fact aside, there are a several advantages to using React Native for mobile application development:
- Cross-platform development. We’ve touched on this already, but the value of code reusability cannot be understated! React Native saves developers time and makes it easier to implement codebases for multiple platforms. Further to this, if your website was developed using React Native that same code can be reused for your mobile app.
- Cost efficiency. Further to its cross-platform capability, React Native is a cost-effective solution for mobile application development. Because the same code can be reused between Android and iOS, there’s no need to hire separate development teams; one effective team can build out your product.
- Community-backed. Open-source frameworks benefit from developer advancement and React Native is no exception. This community of developers provide support to one another when solving coding issues and offer a wealth of knowledge when it comes to using the JavaScript-backed framework.
- Speed. React native has a fast refresh rate and offers fast applications. Its refresh function allows developers to modify UI and implement updates to the app in the background, while the app still functions for users, saving time and improving productivity. While native code does operate faster than JavaScript, this difference is widely believed to be inconceivable to the human eye and thus does not affect the user’s experience.
- Simplicity. JavaScript, the foundation of React Native, is the basis for building out the user interface for a more responsive app with a reduced load time and improved user experience, lending to both simple and complex design needs.
The disadvantages of React Native
While there are many benefits to making use of React Native for your mobile application development, there are some considerations to be made to ensure that this framework is the best fit for your project:
- Compatibility. Through all its success and climb to stardom, React Native is still in the beta phase, which means your developers may encounter debugging or package compatibility issues. This requires troubleshooting which will be particularly challenging for developers with less experience using this framework.
- Scalability. While many companies have seen great success scaling their mobile apps with the use of React Native, such as Facebook and Skype, others, such as Airbnb, found that the framework did not meet their scalability needs and so switched to native applications. React Native, however, continues to make great strides in advancement, so this issue is preventable with support from software architecture.
- Custom module shortfalls. In certain, specialised cases, React Native does not provide the support in terms of customised modules you may need. Either the existing codebase falls short of expectations, or they are missing in their entirety, meaning developers are required to use multiple codebases to achieve fully functioning apps across multiple platforms. While this concern exists, it is also deemed an unlikely event (though it always pays to be prepared for anything).
- Native support. React Native bridges JavaScript with native mobile code, which means that your developer needs to be proficient in native coding to make a smooth transition when building out your mobile application. Alternatively, you’ll need some native development support that could add to your mobile app development cost. You can get around this disadvantage by bringing in some Blott.studio specialist support.
Comparing React Native for Android vs iOS
We’ve discussed all the ways in which React Native is compatible with a range of platforms, but are there some ways in which the programming language differs between them? The short answer is yes. The long answer has three considerations:
- Operating system. Testing your mobile app on both Android and iOS is imperative to ensuring the best possible experience and smooth functionality for your user. The difference between Android and iOS then comes in with regards to the fact that Windows cannot run Xcode, meaning you will need to use a macOS device to test your application, rather than Windows.
- Native elements. While you’ll be making use of the React Native library for the native components of your application, it’s important to keep in mind that they each look and function differently so anything you implement for Android may present with a slight difference on iOS.
- Styling. UI elements differ between Android and iOS, which means that manual configuration will come into play when working on the visual elements of your mobile app. Each platform has its own unique display manner, so developers need to account for this.
When shouldn’t you use React Native?
React Native is particularly a great option for companies looking to get to market quicker or those with a smaller budget but would not be considered a suitable framework for projects that require native functions, a specifically complex user interface, or for an app only required for one platform.
Naturally, there are a few considerations to take into account when determining if React Native is the right fit for you. With this being said, the value of React Native outweighs any small niggles. It is a well-loved programming language in the developer community for its cost efficiency, quicker development process, and time-saving aspects, while offering a broader audience for your product with its cross-platform capabilities. Community-backed by Facebook, Uber, and Shopify, amongst others, React Native further cements its worth as a framework worth considering for your mobile application development.
The team over at Blott Studio would be happy to answer any of your questions regarding React Native as a solution for your mobile application; get in touch!
The value of having a market-ready product cannot be understated; this is true for every industry but almost more so within the fintech space.
The value of having a market-ready product cannot be understated; this is true for every industry but almost more so within the fintech space. Many apps fall flat (approximately 9 out of 10 for those who are keeping track) and are unsuccessful due to a lack of vision, clear purpose, and understanding of their target audience. The fintech industry is ever-evolving and is seeing a surge in recent times, so now’s the time to have a crystal-clear strategy in place so that your fintech product is foolproof and ready to succeed in the market. The Blott Studio team shares top tips for a market-ready fintech product to set start-ups on the path to success.
The Game Plan
As with any business model, your fintech product should have a clear vision and mission. Consider what specific problem in the market your start-up aims to solve. There are several types of fintech products readily available: online banking, payment services, cryptocurrency – the list goes on. While these apps make for great inspiration, one can easily get swept up in the beauty and brilliance of many apps and end up with a list of functionalities to incorporate into your own design, which will only lead to a confuzzled fintech app. Take the time to jot your idea down onto paper and define the value proposition, the core features of your app, how you plan to acquire customers and your revenue model. Having a clear aim in mind will help to declutter the interface, provide clear direction to your development team and result in a seamless UX. Your game plan should make it very clear what the core functionality of your product will be to give you a competitive edge, as well as highlight a few features that help address your customers’ concerns in a unique way.
The Value of Research
Market research is crucial to ensuring your fintech product is market-ready. Market research dives deep to give you as many insights as possible to best understand your audience and how your product will best serve their interests. Without it, your fintech product won’t have a clear goal and is more likely to struggle in the market.
First, market research identifies, A. if there is a need for your fintech product and B. who your target audience would be. Understanding your target audience and their needs helps to best know how to serve your niche with your fintech product’s capabilities. (This research may even show that the specific version of your fintech product won’t be well-received, but we’re a “cup half full” type of group at Blott Studio, so keep reading for our solution to this hypothetical.)
Complying with financial service regulations in your area of operation is of utmost importance and the next important step in your research. Any number of restrictions and licensing could be required; we recommend partnering with a development agency that has experience operating in the area you wish to launch and who can give you valuable insight and assistance.
As with any market, trends and challenges exist within the fintech space. Trends could potentially help your fintech product, while challenges may hinder it, so it’s important to monitor and be aware of how the macroenvironment could influence your product. Artificial intelligence systems, blockchain, and cybersecurity are just a few of the trends your start-up should be tuned in to.
Customer Discovery
Once you have a handle on market research, and you’ve done your initial homework, you’ll want to switch gears and zone in on your ideal customer, and how your fintech product is going to solve a pain point they have. Start by making estimations about your market and spend time working down to a niche audience. The aim of customer discovery is to determine your niche, outline the problems they have, see how your product can solve that problem and if your solution is something they would be happy to purchase. For this reason, and building on the research you’ve already undertaken, you’ll want to conduct surveys and host focus groups and/or one-on-one interviews. This is a learning curve for you to ensure that the fintech product you’ve outlined will be successful in the market, so take the time to ask in-depth questions that are open-ended, start discussions, and gather as much feedback as possible.
Learning to be Flexible
An important lesson to be carried is that you may need to be flexible. (Ah, yes. Here it is – the hypothetical we mentioned earlier.) In some scenarios, fintech start-ups come up with their prototype, conduct theoretical research, and launch their MVP to early adopters and realise that what they initially had in mind for their app, is actually not the solution their niche needs. Oftentimes, what does happen is that they learn that a part of what they initially had in mind, rather than the sum of all parts, is what their target audience is looking for right now. Enter, the “pivot”. By way of example, when we say, “a part” what we mean is that you may have planned a two-part functionality, such as “Save” and “Invest”, or “Buy” and “Exchange”, only to realise that to best serve your niche market, you should be sticking to “Save”, or “Exchange”, only. By identifying this change through user feedback, your start-up can pivot and double down on serving exactly what it is your customers are looking for in a fintech app. When we say, “right now”, what we mean is that you won’t necessarily have to give up the remaining capabilities you had hoped to incorporate, only that these capabilities should be tabled and considered as future additions. Being flexible does not only mean that you should be pivoting but listening to your early adopters and turning their feedback requests into deliverables wherever possible, as well. Working with and aiming to serve your niche grants you a higher probability of generating loyalty and longevity.
A Strong Foundation
“A Jack of all trades” means “master of none” and the last thing you want to do is jeopardise your start-up, especially when you’ve laid all the groundwork and spent valuable time tinkering with your fintech solution. So, don’t overlook the impact of quality development when it comes time to. At Blott Studio, our specialty is with fintech start-ups. Our gurus and wizards have years of experience in the fintech space, meaning they can add valuable input to the development of your fintech product and create an incredible user interface and experience. The ideation and market research conducted up to this stage should all weigh into the creation of your fintech app and it’s important that you can trust the team you’ve enlisted to bring this vision of yours to life.
Working to fulfil that product-market fit is no easy feat, and is by no means a small task, so it’s important for any fintech start-up looking to launch to market to take the time to fully develop the strategy, from ideation to launch. As we’ve discussed, some tips to keep at the forefront of strategic planning have to do with: developing a clear goal, centralised on meeting the needs of a target market, conducting thorough research in support of this goal (or highlighting any shortfalls or opportunities), to connect with a quality fintech development team and to remain flexible throughout the process. With these tips in-hand, your fintech product will be well on its way to being market-ready and geared towards success.
Connect with the Blott Studio team to find out how our expertise can best help your start-up develop a market-ready fintech product.
The Blott Studio family includes not only our own team, but the teams that make up our client base.
The Blott Studio family includes not only our own team, but the teams that make up our client base. Our main mission is to see our clients succeed and thrive and, naturally, we’re their in-house cheerleaders. So, our excitement for the Uplinq team comes as no surprise when hearing of their successful pre-seed funding round that raised USD $3.5 million recently!
UPDATE: Uplinq has announced a $1.25 million investment from ATX Venture Partners and become part of the ATX community.
Uplinq’s journey began with recognising a pain point in the market: traditional credit indicators do not offer valuable insights when it comes to small- and medium-sized businesses looking to secure a loan. Founders Ron Benegbi and Patrick Reily immediately set to work in crafting an incredible technology that better provides data signals to SMB lenders so they can make informed decisions.
Venture capital investors, N49P and Surface Ventures, led the charge shoulder-to-shoulder with a collection of Fintech investors, such as: MoVi Partners, Impulsum Ventures, Magic Fund, Assure Syndicates, and the DVC Syndicate. Other participants included the former President of Experian Consumer Services, co-founder at SoFi, former CIO and Treasurer at JPMorgan Chase, former Head of Risk at Scotiabank, and the former CTO at FIS. With an impressive USD $1.4 Trillion in underwritten loans approved since its inception at the start of 2022, it comes as no surprise to see Uplinq being triumphant during pre-seed funding rounds and securing USD $3.5 million to support their ongoing mission.
The funds raised will be dedicated towards refining their product, so SMB lenders will continue to receive improved and precise indicators that will add value to their decision-making process, and better serve their customer base of small- and medium-businesses needing loan support. In addition to these improvements, the investment will further aim to scale Uplinq’s operations, so SMB lenders across the globe may have access to their cutting-edge technology.
Patrick Reily, co-founder at Uplinq, shared his comments, “SMBs create jobs and are at the bedrock of intellectual property creation around the world. These companies drive the global economy, yet as borrowers they remain perhaps the most underserved segment on the planet. At Uplinq, we can change that. That’s why I am humbled and honoured to now partner with so many brilliant and purposeful people who share our vision.”
Uplinq co-founder and CEO, Ron Benegbi, added, “Now, it’s great to have so many amazing people who want to be part of our journey. Through this raise, we have connected with people like ourselves, who truly care about others and who want to lead with empathy, humanity, and purpose. Together, we all share a collective vision of making an impact on the lives of millions of families worldwide.”
Working alongside Uplinq to deliver a first-of-its-kind global credit assessment platform for SMB lenders has been nothing short of an incredible experience. The team at Blott Studio are honoured to have been involved in this project and to continue working with such Fintech innovators to Improve the Uplinq product and experience for its users. Read all about the Uplinq project on our case study, here. Be sure to keep up-to-date with Uplinq on LinkedIn, here, and give us a follow while you’re at it, here.
Established in 2018 in the heart of London, Blott Studio is a hard-working digital agency from and for fintech.
Established in 2018 in the heart of London, Blott Studio is a hard-working digital agency from and for fintech. Our team is filled with the best professionals that are ready to work on different fintech design and development projects. We are passionate about helping promising startups and brilliant enterprises that want to conquer the complex market.
The incredible trust of our beloved clients recently helped us unlock a new award from a trusted industry resource. On The Manifest’s very first company awards, Blott Studio is among the best recommended and reviewed B2B leaders from the United Kingdom! According to their latest report, our team is a highly reliable partner for Google app engine, product design, UX strategy, and many more this 2022!
This is a huge distinction for us. It’s one thing to receive an award for a project but it's a bigger feat to earn it with your clients’ stamp of approval. Since our inception, we’ve gone above and beyond to deliver what our clients need. It’s a huge privilege for us to work with brilliant clients like Zevero, G&G Productions, Shepherd, and Howler.
To give you a better understanding of this honour, The Manifest is an independent business news resource designed to publish insightful content like agency shortlists, how-to guides, and market reports. The awardees for their inaugural company awards were chosen based on the testimonials and referrals they’ve earned over the past year.
Needless to say, our clients’ made this moment possible. From the entire Blott Studio team, thank you so much for your wonderful trust and confidence! We owe this incredible moment to all of you. We can’t wait to see what lies ahead for us all next year.
At Blott Studio, we believe that the user comes first when designing and developing. Connect with us today and let’s work on an exciting project together.
Let’s talk about digital currency. There are varying reports on the fintech industry's growth trajectory, but one thing is clear: it’s on an incline and is certainly here to stay.
Let’s talk about digital currency. There are varying reports on the fintech industry's growth trajectory, but one thing is clear: it’s on an incline and is certainly here to stay. This industry boom has resulted in a number of financial services, digital currencies, and other products coming to light within the space. Digital currencies, such as cryptocurrency, and the newly “minted” central bank digital currency, are among those taking the world by storm. But what are digital currencies and why or how do they hold value? We delve into digital currency and take a look at how they’re shaping the future of payments.
Types of digital currency:
Cryptocurrency.
Cryptocurrency, in case you need a refresher, is a digital currency that is secured by cryptography while having its transactions verified and records maintained via a decentralised system, rather than relying on any central authority. You’ve undoubtedly heard of Bitcoin, the most popular cryptocurrency to date which reached an all-time high value of $68,000 in November 2021. (At the time of writing this, Bitcoin’s value was sitting pretty at $23,484.) Cryptocurrency has somewhat disrupted the payments space, and it was this disruption that caused central banks around the world to take a pause and consider joining in on the action.
Central Bank Digital Currency.
CBDCs are similar to cryptocurrencies in that they are also considered to be a digital currency, however, these digital tokens are issued by the central bank and their value is underpinned by the relevant country’s fiat currency. Other differences include CBDCs use of private permissioned blockchain networks versus cryptocurrency’s open networks that do not require permissions. Cryptocurrency holders are pseudonymous, offering some sense of anonymity, whereas CBDC’s are linked to a person’s bank account and therefore identity.
Stablecoins.
Stablecoins are a variation of “traditional” cryptocurrencies, and as the name suggests, they were invented to be less volatile and, thus, more lucrative for widespread use. The value of stablecoins is pegged to a country’s fiat currency (much like that of central bank digital currency), financial instrument, or other commodities (such as gold), which is why it maintains stability comparative to cryptocurrencies. While stablecoins are a variation of cryptocurrency, there isn’t a limited amount available; stablecoins are issued based on economic conditions and the value of the assets that back them.
The value of digital currency:
The main advantage of digital currency is that it minimises the time it takes for transactions to be actioned and it cuts down on the costs associated with fiat currency. It is also more secure; blockchain technology ensures that counterfeiting, fraud, and duplication cannot occur. Another advocate for the shift to digital currency use is decentralisation. This means removing governing bodies from the mix, so you have full access and control over your funds when you need them, rather than having to complete paperwork or jump through hoops to gain approval to access or move your money. Decentralising further means that transactions occur between peers, without the interference of third parties (who charge transactional fees and slow the process). Confidentiality has also driven the rise of digital currency as they track only the transaction history and do not collect information on individuals, unlike traditional financial services.
One crucial disadvantage of digital currency to consider, however, is its susceptibility to being hacked. This not only poses a threat to individuals investing but to economies on a larger scale. The importance of security should never be downplayed when it comes to the Fintech industry and digital currencies are no exception.
Why digital currency matters:
Digital currencies are shaping the future of finance by solving several problems associated with cash. The first step toward digital currency has existed since 1950, in the form of credit cards with the introduction of electronic transfers occurring in 1970. The problem, however, is that these methods are expensive and take time to process. Digital currencies aim to remove the time it takes to transfer money and minimise (and in some cases eliminate) transaction costs. Being peer-to-peer, digital currencies also eliminate the need for central databases for record-keeping and offer transparency. In addition, digital currencies solve the concern of “double-spending”. The production and distribution set-up employs a system of serial numbers to ensure that each “note” is unique. Paired with cryptography, blind signatures, and encryption, this ensures that digital currencies cannot be tampered with, further protecting users’ transactions.
While central bank digital currency might still be just an idea with a few kinks to work out, cryptocurrency, CBDCs, and other digital means of payment are certainly disrupting the financial services industry and changing the way the global population manages their money. Any way you look at it, fintech is an exciting space filled with possibility that is shaping our future.
When we think of our mobile devices, we see a lifeline or “our lives” in our pockets, and with good reason.
When we think of our mobile devices, we see a lifeline or “our lives” in our pockets, and with good reason. The world wide web was initially introduced in 1989, to serve universities and scientists with an information-sharing tool, but the introduction of the internet has since spiralled outward and being “online” has drastically affected our day-to-day and stimulated innovation for online living. Nowadays, we rise to the tune of an app, check in with friends and families through social media, and manage our finances, our careers and our schedules – so many facets that are intertwined with our existence can be accessed at the touch of a button. So, it’s no wonder then that we feel a sense of connection and a deep-rooted need to protect this device and the access it grants to our online lives.
This is something that developers are all too aware of, and need to dedicate resources to, to ensure that every website or mobile application that launches to market offers a secure and safe interactive environment. This translates to security protocols. And not just any standard of security; security that will withstand any form of cybercrime and ensure that users find the platform they are using trustworthy. Unfortunately, passwords simply aren’t enough anymore, so what is?
Here’s all you need to know about FIDO Authentication; the new gold standard in multi-factor authentication.
What is FIDO?
Fido Authentication is an open-source library of protocols and standards that assist web and app developers with creating safe and secure platforms that do not require password logins. The types of FIDO used are security keys, facial recognition, fingerprint and voice activation. FIDO Alliance, an NPO that was founded in 2012, discovered that approximately 80% of data breaches occur because of password logins. Armed with this, and other research, the FIDO Alliance group decided to address the insecurities surrounding password use and established a series of protocols to implement instead of password authentication when requiring login information from internet users.
What are FIDO Protocols?
Approximately 200 companies make up the membership group of FIDO Alliance, and together this group determined a set of standardised protocols for passwordless protection. These protocols offer several security improvements, including user authentication using digital signatures, hardware that generates and stores cryptographic keys, and biometrics. In this way, FIDO protocols preserve internet users’ privacy and offer increased security over traditional password methods.
There are three protocols, namely:
Universal 2nd Factor (U2F)
This simple protocol is a two-factor authentication model that combines a second-factor authentication scheme with a password. It works by enabling internet users to access online services with a single security key that does not require drivers or client software.
Universal Authentication Framework (UAF)
This passwordless protocol is only applicable to mobile applications and works by registering a user’s device to online services through a local authentication mechanism. These mechanisms can be biometric or PINs (numerical, alphanumeric and patterns are examples of PINs.) UAFs can also be a two-step process, much like U2Fs.
FIDO2 or WebAuthn
U2F and UAF were the first protocols drafted by FIDO Alliance, and the group soon realised the necessity of a third protocol, one that would combine the two original models. Commonly referred to as FIDO2.0, this protocol incorporates JavaScript to offer accessibility for web applications.
How does FIDO work?
FIDO protocols make use of standard public key cryptography techniques for stronger, more secure authentication services. There are two phases to initiating these protocols, namely Registration and Authentication. Registration is the first phase and involves a user activating a security key per website using an authenticator. Authentication is then the process of validating that user each time they want access to said site. We’ve simplified the processes below:
Steps for Registration:
This process only happens once per website
- User identification occurs through a unique username on the website in question.
- The FIDO server then sends a challenge to the user.
- This challenge validates the user, and the authenticator then generates a pair of corresponding cryptographic keys; one public and one private.
- The public key is shared with the website along with digitally signed metadata and any other relevant content. The private key never leaves the user’s device.
Steps for Authentication:
This process happens each time the user returns to the website
- User identification occurs through a unique username on the website in question.
- The FIDO server then sends a challenge to the user.
- This challenge validates the user, and the authenticator then digitally signs the challenge and accompanying metadata.
- This response is shared with the website.
- The website verifies this digital signature against the public key supplied during Registration and authenticates the user.
Why should you use FIDO?
While there are many authentication options on the market, FIDO Authentication is considered the first strong authentication technology that addresses several problems that extend beyond security to ease of use and affordability. FIDO also contemplates what may be deemed “commonplace” when using the internet. The protocols take into account elements of internet use like JavaScript in browsers, USB ports and usage, Bluetooth Low Energy (BLE) and Near Field Communications (NFC) on mobile applications, to mention a few. FIDO also understands that most users make use of their smartphones for internet access and that these encryption-capable devices are likely to be their authenticators, so purchasing specific authenticator technologies is not necessary. Another advantage of FIDO is that it is compatible with other authentication technologies for a transition period, provided the web application is programmed to route the user through the correct authentication process.
Not only is FIDO an affordable solution that is easy to use and integrates well with our daily-use devices, but it is also backed by the well-known platform manufacturers of Microsoft Windows and Google Android, and the browser creators of Mozilla Firefox, Google Chrome, and Microsoft Edge.
FIDO Alliance has worked to protect users’ privacy and to prioritise shared secret authentication. While the world continues to shift further toward digitalisation, the priority of our security and privacy becomes more prevalent. Each protocol drafted works to offer security solutions for a range of risks, devices and technologies to mitigate cybercrimes and provide developers with the solutions needed to build out the best possible websites and mobile applications that users can trust.
Have any other questions about FIDO Authentication, or want to learn how these protocols can be incorporated into your product design? Chat to our development team, here.
At Blott Studio, our aim is to continue to serve our clients with the best possible service, and that means continually offering cutting-edge design and development solutions.
At Blott Studio, our aim is to continue to serve our clients with the best possible service, and that means continually offering cutting-edge design and development solutions. Our team is always monitoring design trends, new development tools, and conducting further research to ensure our team is equipped with the best possible skills and capabilities to serve your needs. We’re proud to announce that Blott Studio has officially become an accredited Webflow Partner. We delve into what that means and why we decided to join the community of developers who have come to love Webflow’s platform.
So, what does it mean that we’ve become a Webflow Partner? Essentially, Blott Studio has been vetted by Webflow and is an accredited and certified Webflow service provider. Webflow Partners are any individuals or agencies who are trusted and deemed professional experts at Webflow by Webflow themselves!
What is Webflow?
Webflow is a SaaS company that offers designers website building and hosting services. The Webflow platform offers web design and development, a content management system (CMS), and hosting services so that designers may build out visually beautiful, seamless websites that are completely customisable. Webflow is considered the gold standard of web design platforms as it does not require any coding; the platform processes the designer’s selections within the editor into the appropriate HTML, CSS or JavaScript. This makes it one of the best available tools on the market for web app creation.
How does Webflow compare to other website builders?
Webflow is considered the best website building tool available on the market at present. It is easy to use, requires no coding and saves valuable time for web design teams to deliver modern, stunning websites for clients. Naturally, other website building tools each have their strengths and weaknesses, though none quite match up to Webflow’s level of customisation. Here’s why:
WordPress
While WordPress is a great web-building tool for beginners, its coding layout is considered cluttered due to the high volume of plugins. WordPress also does not offer options when it comes to coding solutions, whereas Webflow allows users to employ their custom coding or make use of Webflow’s provided coding. On the topic of user interface, WordPress offers limited features compared to Webflow’s extensive design functionality, including animations and illustrations.
Wix
When it comes to template-based web-building, Wix isn’t a bad option, though Webflow also offers a selection of templates that are more extensive. It also offers customisation of these templates. The Webflow editor visualises HTML and CSS code for a better building experience, while also offering a library of code snippets to integrate into the design.
Squarespace
Squarespace offers web-building services to get a simple website operational within a few hours. While it may take more time to develop a site, Webflow offers a more extensive, highly advanced service and incorporates integrations with Shopify and Google Analytics for a more holistic approach to web development. Further hereto, Webflow’s hosting capabilities mean that these integrations operate efficiently and that updates are instantaneous.
Shopify
Shopify is a great solution for e-commerce-centric businesses, though this makes it a very niche tool. Webflow offers integrations with Shopify, so your clients do not have to sacrifice on high-end design. Webflow also offers plugins to assist with sales tracking metrics to further support e-commerce initiatives.
What are the benefits of enlisting a Webflow Partner?
Certification
Webflow Partners are specifically required to have at least one accredited and certified Webflow designer or developer on their team to be deemed certified. Blott Studio has 5 as of 16 Nov 2022. The certification process involves a series of exams that must be passed with an average score of 88% or higher (per). Passing these exams provides certification to the individual for 12 months only, so every year our Team of Webflow experts is required to retake the exams to remain certified and ahead of the trend. This ensures that the team you work with when designing and developing your website, has the relevant expertise and understanding of Webflow to deliver the best possible outcome.
Trust
Becoming a Webflow Partner means that Webflow trusts Blott Studio to deliver high-quality website design and development through advertising their platform and offering Webflow solutions. Blott Studio’s Webflow design and developers can provide our clients with cutting-edge web technology solutions that best serve their interests and goals. As we’ve mentioned before, Webflow only accredits designers who pass their rigorous testing programme with distinction, on an annual basis. You can rest easy knowing that our Webflow designers maintain these standards to be considered Webflow-worthy.
Experience
The Blott Studio team has worked on over 50 Webflow projects over the past 3 years. These projects have scaled from fintech startups to enterprise, across several time zones, spanning a few countries. Our expertise has been nurtured with every completed project and we’ve thoroughly enjoyed resolving the challenges our clients have come to us with, to guarantee their satisfaction. This experience further ensures efficiency when working on your project; our team adheres to deadlines and offers sound advice and pricing structures from the onset.
Which Webflow Services does Blott Studio offer?
At Blott Studio, we innovate. Our designers and developers work ahead of the curve to deliver high-quality, seamless websites that are future-proof. We continually aim to improve our skills and capabilities so that our clients receive holistic support for their brand. As a full-service Webflow studio, Blott Studio offers a range of Webflow services, including:
- Webflow Ecommerce Websites
- Webflow No Code App Creation
- Webflow Design System Setup
- Webflow Platform Migrations
- Webflow Interactions & Animations
- Webflow Custom Code & Integrations
- Webflow CMS Setup
- Webflow Marketing & SEO Guidance
- Webflow Rebrands & Redesign
- Webflow Third-Party Integrations
- Webflow Accessibility
- Webflow Training
As an official Webflow Partner, Blott Studio can assist in a range of services to support your business, from web or app design and development to branding, strategy and marketing. Having a full-scale team of Webflow accredited experts means that we can tailor a Webflow solution to you, drawing on our team members’ relevant experience and skills, for the best possible results. Find out more about our Webflow services and schedule a chat with us here.
Fintech’s have reshaped customer expectations, setting new and higher bars for user experience.
“Fintech’s have reshaped customer expectations, setting new and higher bars for user experience. Through innovations like rapid loan adjudication, fintech’s have shown that the customer experience bar set by large technology firms, such as Apple and Google, can be met in financial services.” - Deloitte, 2020. These large technology firms have shown that user experience is any business’ salvation, and it’s no different for fintech solutions that already face the challenge of trust straight out of the gate. This blog takes a look at how user experience can transform your fintech product into a long-term success.
Defining User Experience
User experience (or UX) is a broad concept that explains how any person interacts with a product or service. Within the digital industry, UX design often means said person’s overall satisfaction with a digital product that provides value, function, usability and a positive impression. The purpose of UX design is to provide a series of conditions that best facilitate the user to ensure gratification. The priority when aiming for quality user experience is meeting the needs of your users, while the second focus is doing so in a way that seamlessly integrates all the functions of your business into a cleverly crafted application.
Five Things Fintech Start-ups Should Know About User Experience
- UX is more than just usability
While users appreciate apps that are easy to use and navigate most, it isn’t the only element that they anticipate from a great website or application. Ease of use is just one of the things users expect from their digital product, and having usability only may not guarantee that individuals will use your fintech app.
- UX and UI are two different things
User experience (UX) and user interface (UI) are ofttimes used interchangeably, but user interface is only one part of the whole user experience. User interface refers to the visual representation of your app, while the user experience incorporates user interface design along with other processes to create a high-functioning and visually appealing experience.
- UX is customer-centric
Successful fintech apps are apps that put the user first. Quality UX design is all about understanding your customers, meeting their needs and addressing the problems they have through your product. Part of the process to achieve the best possible user experience involves researching your customers’ behaviours, aspirations and pain points.
- UX continually evolves
Delivering on user experience is a never-ending cycle that ultimately forms part of your service delivery. Once the product launches, it becomes imperative to translate feedback from users into actionable requests. Technology advancements also influence and improve the user experience, while regulations within the fintech space evolve, and need to be accounted for, too.
- UX should support your business
Just as users have needs that should be met through the development process of your application, so too does your business. The design process of your application should aim to find a balance between providing a quality user experience and offering a technical solution that supports your business needs.
Understanding user experience as part of your fintech solution design and development process assists in laying foundations for building out a quality app that has a higher chance of success in the market. But what exactly are the benefits of prioritising user experience?
Five Benefits of User Experience for Fintech Start-ups
- Minimises development costs
UX designers begin their whole process of development by first identifying the customers’ needs through rigorous research to best understand how to serve them the ideal product solution. This makes them more productive. It also means that there is less time spent on reworking any element or function of the application once it has launched, based on user feedback, as the groundwork has already been done. Some studies have highlighted that 50% of programmers’ time (not to mention budget) is wasted on work that could have otherwise been avoided.
- Attracts new users
Word-of-mouth marketing is considered the best advertising tool to-date. If you deliver on a quality user experience and high-end fintech app, chances are your loyal users will love it and recommend it to their friends and family. This results in more customers for you, so working to ensure your product is user-friendly and enjoyable is time well spent.
- Increases conversion
Global digitalisation has meant that more individuals and generations are growing up with technology in-hand, which means their expectations of websites and mobile applications are high. Providing a fintech app that is easy to navigate, has clear flow and offers call-to-actions at the right time drives conversions. 75% of users judge websites on how they look and feel, and it is this quality user interface that will convert.
- Builds brand loyalty
Long-term growth and success for any business is driven by positive brand experience, which happens at every customer touch point. Delivering an inefficient app or non-functional website are ways to guarantee customer losses. UX designers are best equipped to not only deliver a high-functioning fintech app, but also encourage trust in your product; something that is a challenge when it comes to financial services.
- Reduces operational costs
A quality fintech app that prioritises user experience produces a product that is easy to use. This means that users will require minimal additional customer support to navigate and make use of your product, saving time and resources that can be better dedicated elsewhere in the business.
It’s not just enough to understand user experience and know how it can affect your start-up, you need the right developers working alongside you to implement UX tools effectively. Peter Morville, a leading expert and author on the topic of user experience, classified his seven-trait model that developers should follow in order to achieve quality UX in their designs:
The Seven-Trait Honeycomb Experience Model
- Usable. Any web or app-based product should be easy to use. If a user cannot navigate your website or complete the tasks your app was designed to fulfil, they will not use your product. Iterating and user testing help to ensure that your product is user-friendly.
- Useful. While usability speaks to the ability of the user to make use of your product, usefulness refers to what need your product fulfils in their lives. Being useful is a necessity. If your product does not serve a purpose, you will not retain customers. Thorough research and sticking to core functionality help keep your goal clear and your app useful.
- Desirable. How desirable your product is to the consumer is influenced by social trends or culture, emotional connotations and advertising. The appearance of your product should attract and retain customers. Thoughtful user interface design and marketing strategy influence desirability.
- Findable. The creation of any website or application involves troubleshooting, and it is important to ensure that the solutions to any foreseeable problems you can identify throughout the development process are made available to users. If a user cannot find the information they need, or resolve a problem they have, they will not keep using your product. Organising information, providing context, and search features all form part of a findable product.
- Accessible. Ensuring your website or mobile app can be accessed by all your users is of paramount importance. Products that are difficult to use or inaccessible are not easily adopted in the market. There are a number of ways to make your app more accessible, from iconography to functionality.
- Credible. Credibility is crucial in the digital age, and even more so when it comes to financial services. Any individual or scammer can create a website or mobile application, so users are naturally more sceptical of products they do not know. It’s important to gain trust and build brand loyalty. This can be achieved through BJ Foggs four types of internet credibility:
- Presumed: being supported by a well-known brand.
- Reputed: through word-of-mouth marketing or customer reviews.
- Surface: your brand’s perceived professionalism through its web design and tone of voice.
- Earned: based on a user’s previous experience using your product.
- Valuable. All the other six traits go a long way in establishing value, but it is important that your product provides value for its users, as well as for your business. Value is generally reflected in financial gain or time spared.
Quality user experience is the difference between effective, high-converting websites and applications, and failed startups. Partnering with a development and design studio that prioritises UX and UI will grant your fintech startup a competitive advantage, build brand loyalty and drive sustainability for your business. Get in touch with our UX designers today, click here.
Security measures were not always front-of-mind when application development first hit the scene, but with the industry expected to reach over $935 billion in revenue by 2023.
Security measures were not always front-of-mind when application development first hit the scene, but with the industry expected to reach over $935 billion in revenue by 2023, and with over 143 billion apps and games downloaded in 2021 alone, this space surely has skyrocketed and welcomed many opportunists ready to assess applications for any vulnerabilities they could potentially exploit. It is for this reason that application security is now a high priority when it comes time to develop and design a product, and for fintech this need is even more pressing. So, here’s an introduction to application security and why your fintech should care about it.
Defining Application Security
Application security is defined as the steps taken by a development team to create, test, add or manage the relevant security measures of applications and platforms to mitigate threats, such as attacks or unauthorised access, and strengthen vulnerabilities the application may have. Application security measures may be any combination of hardware, software, protocols, and best practises to protect against vulnerabilities. Examples may include proxy servers, firewalls, or encryption, though the overarching goal of application security is always the same: to prevent security breaches.
Reasons Application Security is Important
The main reason applications are considered vulnerable to threats is that they are connected to the cloud and made available through numerous networks. In recent times, hackers have shifted focus to infiltrating applications and it’s easy to understand why. According to Think with Google, the average person has approximately 35 applications on their mobile device. Companies are under pressure to guarantee their customers’ security, not only on a network-level but within the application itself. Other reasons why application security matters are:
- Reduces a company’s attack surface. An attack surface is the number of entry points any application has where unauthorised access could potentially be gained. Finding and eliminating entry points reduces the attack surface and ultimately, a security risk.
- Proactivity minimises risk. A company that is proactive with its security measures is better protected against orchestrated attacks and thus minimises potential losses that otherwise could have materialised, had the company been reactive instead.
- Fosters trust with customers. Any individual that makes use of a website or application wants to know that their online activities, personal information, and assets are protected and secure. Consumers are more likely to trust your product, and use it, if they know that privacy and security are a top priority.
Types of Application Security:
Authentication
Authentication security is built into the application itself by software developers. The purpose of authentication procedures is to ensure that only the sanctioned person may gain access to the app by incorporating “login” features such as a password, security questions or biometrics. The use of multiple authentication procedures is commonly referred to as two-factor authentication and simply takes this security measure one step further by combining two protocols. For example, logging into a mobile app with a password in addition to scanning a fingerprint.
Authorisation
Authorisation is the second step, after authentication, whereby the application needs to vet the person who has been authenticated against a list of authorised user accounts. While authentication takes place within the application itself using software programming, authorisation is stored and managed on a server, to feed the relevant data back to the application. Once authorised, the sanctioned person may access and use the application in question.
Encryption
Consideration needs to be made for data or information that is shared between the application in use and the web server or cloud-based application. This information is also considered sensitive and thus should be protected. Encryption protocols transform the data being shared so that anyone who does not have authorisation cannot interpret the data. Examples of encryption include Secure Shell (SSH) and Socket Layer (SSL) protocols.
Logging
Logging is a type of application security tool that offers feedback to the development team. Application log files continually track who is accessing the application and how they achieved access. This tool becomes particularly important in the instance of a security breach as it provides further information as to who gained access to the application and in what way, so that vulnerabilities can be identified and fixed.
Testing and Control
It is important to continually administer system checks and testing protocols to ensure your application security measures are functioning optimally. There’s no point in investing resources into security measures if they aren’t maintained, and/or become ineffective! Developers may wish to conduct security audits wherein the application is reviewed to ensure it meets a set standard of security criteria. Penetration testing is also common and entails developers acting as cybercriminals to search for application weaknesses. Fuzzing is another measure of testing and control. Developers purposely use unexpected inputs to review how the application reacts and assess these reactions for vulnerabilities.
Now that you have a better idea regarding the types of application security, what are some examples of how these might look in real-world applications?
Application Security for Web
Web applications are apps or services that are accessed via a browser interface on the internet. The application data is stored on remote servers which is why the information must be transmitted to the user via the internet; it is for this reason that application security for web applications is considered a high priority. The most common way to protect a web app’s network is by way of a web application firewall. These work by managing data packets. The firewall software reviews these data packets and blocks any that may pose a threat to the web application.
Application Security for Mobile
As with web applications, mobile applications are considered vulnerable because they too transmit information via the internet and not a private network. Companies may wish to provide a private network for their internal staff by incorporating a virtual private network (VPN) into their application security measures. Vetting mobile applications used on company devices by staff is another way to protect against threats. When it comes to providing efficient application security for your fintech mobile apps, several tools can be utilised, including conducting regular scans for malware and protecting against unauthorised logins with sufficient authentication steps.
Application Security for Cloud Apps
Cloud-based applications are considered a bit trickier than web or mobile. Not only do cloud-based applications operate online, but cloud environments share resources. Due diligence must be taken to ensure that only authorised users have access to the relevant data on the cloud.
We’ve looked at what application security means for your mobile or web-based application, in what ways it is important and can benefit your product development, as well as the different ways to achieve a secure environment for your customers. Feel that it’s time to start caring about your fintech application’s security? Connect with our developers and find out how we can help you create an app with a Fort Knox likeness.
A guide to web application development could be summarised into three words: planning, framework, and architecture. But it shouldn’t be. In all fairness, it is much more complicated than that.
A guide to web application development could be summarised into three words: planning, framework, and architecture. But it shouldn’t be. In all fairness, it is much more complicated than that. Web applications have become highly specialised, advanced products that combine the best of mobile applications and web browsers to offer users quality solutions. Several businesses have chosen web applications over mobile applications (we highlight a few in this article), due to their capability of being used without needing to be installed and not requiring updates that interrupt usability. Web applications simply require sufficient planning and thought to result in seamless creations that users will not only adopt but recommend to others. This guide to web application development delves into what the development of web apps entails, the types thereof, and which frameworks to consider for your web application.
What is a web application?
Web applications are computer software programs that are accessed via a browser, which users can interact with. Web applications are typically made up of two parts, the server-side, that processes input data through HTML, AJAX, or API requests, and a client-side, that interprets the data and displays it for the user in the interface.
Web applications differ from other applications in that they combine mobile applications and websites. While a mobile application relies on mobile devices and a native environment, and a website only displays information via web pages that are not interactive, a web application offers the interactivity of mobile, and the same amount of (or more) information compared to a website.
Some examples of web applications include:
- Google Docs Editors
- Canva
- Netflix
- META
- Twitter
The benefits of web applications
It’s commonplace to find web applications more popular than websites in today’s digital world; often, companies opt to include some form of web app functionality in their sites, whether that be in the form of chatbots, ecommerce or completing forms online. It’s rare to come across a “pure” website, and that probably has to do with the benefits of choosing web application development over websites:
- Web applications are cross-platform compatible, which means they can be accessed and used across multiple devices or browsers without impacting the functionality of the app.
- Web application development occurs on the server-side, or “back-end”, and the client-side, or “front-end”. This makes the web app more manageable, as the development team can implement updates and make changes on the server-side without compromising usability.
- Web applications are more secure because the server-side and client-side operate independently of one another. Specific access is required to manage the data and back-end.
- Web applications are more cost-effective than websites and mobile applications as they require less support and maintenance. Updates can also be made instantaneously.
- Because web apps are more manageable and are cross-platform compatible, they are also more readily available to users who may have limited bandwidth.
The different types of web applications
Selecting the right web application fit for your product comes down to functionality. Each type of web application differs in terms of its structure, or “architecture”, and how it communicates with the server.
Type 01: Progressive Web Apps (PWAs)
Progressive web applications operate and display similarly to that of mobile applications. The difference between PWAs and mobile apps, however, is that the user simply visits their browser to view and interact with the progressive web app, rather than first having to download and install the application on their mobile device. Another advantage of a PWA is that it combines all the benefits of native mobile apps with web browser functionality, which combats the need to develop separate native apps for different operating systems (this is especially beneficial to ecommerce merchants). Further to this, progressive web apps incorporate a “service worker”. The “service worker” adds value to the user as it uses caching to allow for offline features. What this means for users is that they can quickly access stored information from their last interaction with the web app, even when they do not have internet access.
Type 02: Single Page Applications (SPAs)
As the name suggests, a single page application consists of one page that is browser-based and combines static information with elements of display that can change. Email apps are typically SPAs and display a navigation bar that is static (remains on your screen), even when you scroll through your inbox messages. The server-side communicates with the client-side of the SPA through its tiered architecture to render specific subsets of information, rather than entire web pages. So, when a user requests specific information from the web app, an API request is triggered and instructs the business layer to execute the request, also known as the “logic”. The information is exchanged through the data layer of the tiered architecture and a JSON file (the response from the server-side) is then submitted to the browser to display on the presentation layer. The great thing about this functionality is that the load time for users is drastically reduced, though initial load time may be a bit slow. SPAs are also considered cost effective web application solutions.
Type 03: Multi-Page Applications (MPAs)
Naturally, if SPAs exist then MPAs exist as well. Multi-page applications are web applications that consist of multiple web pages that are all linked to one another. This type of web application is considered more traditional as the app display needs to reload each time a new data request is made. With multi-page applications a request is made by the user, which then needs to be transferred to the server-side and returned to the browser to action. Each page not only has its own functionality to load or reload information, but also requires its own design layer. Multi-page applications are a top consideration due to search engine optimisation. Indexing for search engines is made easy due to the multiple-page hierarchy and use of internal links. Further to SEO, MPAs are also beneficial to businesses who may wish to scale up in the future, as these products or services can easily be integrated as new web pages.
Type 04: Static Web Applications (SWAs)
Developed using jQuery, HTML, AJAX, and CSS, static web applications are web apps that are not interactive, though they do offer compatibility for animations and video content to enhance the user interface. Static web apps process requests by sending that request to the server which then identifies the correct HTML file and sends it back to the browser. The browser then processes the file and displays it to the user. Static web apps make use of JavaScript to transform the information request into a display output. Static web applications are particularly useful to launch to market quickly, as they incorporate “building-block” coding that every web developer is familiar with (HTML, JavaScript, and CSS). They are also scalable and flexible.
Type 05: Dynamic Web Applications (DWAs)
While static web applications are rather simple and straightforward in their construct, dynamic web applications are more complicated. DWAs can be single page websites or have multiple web pages, but what truly distinguishes them is their level of interactivity. A dynamic web apps’ functionality, appearance and content changes at every user request or input. Three components work together to provide this level of interactivity, namely: the backend component, the client-side component, and the front-end component. Users input a request on the frontend, which is then processed through the central admin panel to collect the data from the backend and share it to the browser which then actions the appropriate response to update the information, display or visuals of the DWA for the user. The benefit of a dynamic web application is that server access is not required and thus implementing features is quick and easy. Moreover, updates happen each time the user accesses the DWA. Dynamic web applications are also loved amongst developers because they are easily, and quickly, updated to keep up with the evolving demands of users.
Type 06: eCommerce Web Applications
At their core, eCommerce web applications are multi-page applications that have added features and functionalities incorporated into their design. Examples include online payments, shopping carts and delivery tracking metrics that are all considered dynamic. eCommerce web applications are truly beneficial to businesses looking to provide an uncompromised user experience because they offer personalisation to a degree that websites cannot.
Type 07: CMS Web Applications
Content Management System web applications are a mouthful, but this type of web application is simply a CMS that offers tools to better manage the web application itself. Examples of CMS web applications are WordPress and Webflow. These Content Management Systems offer the building blocks for the web application that can be managed by web developers, as well as those who do not have the technical capability to create, curate, modify and manage their own web apps. They must simply follow the template guidelines. The main benefit of CMS web applications is that more users can have access to the content and be able to make changes to or update that content, resulting in faster updates.
We’ve discussed the different types of web applications for you to consider, but now you need to understand the different frameworks available to you, to develop the web application that will meet your needs.
Web application development frameworks you should know
Web application development frameworks offer valuable tools and features that form the foundation of building out the right web application for your business. Frameworks also provide libraries and scripts of ready-to-implement coding for specific functions and features that streamline the development process.
Ruby on Rails (RoR)
Created by David Heinemeier Hansson in the early 2000’s, Ruby on Rails is a web application development framework that is founded on the Ruby programming language that combines HTML, CSS, and Javascript for improved interactivity. Commonly referred to as Rails, RoR is an open-sourced web application development framework for the server-side, with a Model-View-Controller architectural core. Airbnb, Dribbble and Shopify are examples of companies who have incorporated RoR into their web applications. Ruby on Rails is considered a quality web app development framework that can efficiently manage high levels of traffic, though some developers feel it has begun losing the popularity contest due to newer frameworks that offer improved functionality and expanded feature capabilities. Special mention needs to be made for RoR’s Do Not Repeat Yourself approach (or DRY approach) that minimises bugs as the code is never repetitive, always simplified and easily managed.
PHP
Sometimes referred to as a hypertext pre-processor, PHP is an HTML-embedded scripting language that creates dynamic web applications by melding Common Gateway Interface (CGI) with HTML. Due to PHP being based in HTML, this framework is compatible with MySQL, Oracle and more. Facebook, MailChimp and Wikipedia all make use of PHP web application frameworks. PHP offers developers a way to build dynamic web apps that are flexible, simple, and quick. Developers also love this framework as it has template scripts that are ready-to-use, can be supported across most major operating systems, and, like Ruby on Rails, has a Model-View-Controller architectural core.
Node.js
Node.js has quickly gained accolades as a preferred framework by developers since its first introduction in 2009 by Ryan Dahl. Node.js is an open-sourced JavaScript-based platform that offers server-side capabilities, which can also be supported on Chrome’s V8 Javascript engine. Express, Socket.io, and Meteor.js are all Node.js-founded frameworks that enhance the back-end of web applications. Node.js is unrestricted and allows developers to code their hearts’ desires. Other benefits of this framework include its scalability and improved performance thanks to the framework having non-blocking I/O compatibility. Developers also love Node.js because the back-end can be coded in JavaScript, one of the most commonly used coding languages. LinkedIn, Uber and PayPal are all examples of businesses who used Node.js to develop their web applications.
Angular
Angular was borne from Angular.js, which was a project undertaken by Miško Hevery during his time at Google. The framework was later open-sourced as developers at Google felt that, because it was based in typescript (out of JavaScript), it could be widely used and incorporated for front-end web application development. Angular is a fast-to-market framework as it offers a collection of tools and libraries that make building out the web app much faster and more efficient. The coding snippets are also cross-platform compatible for further efficiency. Examples of companies who used Angular are Gmail, Forbes and Upwork. We’ve mentioned Angular’s biggest benefit is how quick it is to develop, but that isn’t its only benefit. Angular also minimises coding error thanks to its foundation in typescript, allows for more integrations and is easily customisable.
React
React.js is an open-sourced framework based in JavaScript that offers developers the tools and building blocks needed to create feature-rich web apps without having to spend ample time coding new lines or changing existing code. These ready-to-use components simplify the development process without compromising on a quality user interface. Instagram, Netflix, and WhatsApp are examples of React.js web applications. These, and other companies, have opted for the React.js web application framework as it is supported by a large community of developers, offers code readability, and does not require the front-end of the web app to reload to update.
The stages of web application development
While you may have an idea of the type of web application that will suit your needs, and better understand the frameworks available to you, something we would be remiss not to mention is the process that should be followed when undertaking your development project. There are a set series of steps to be followed to ensure the best possible web application outcome:
Step 01: Define a problem
To create a web application that users will adopt and recommend to others, you first need to determine what problem you can solve for them. Thus, the first stage of the process of web application development involves in-depth research and data analysis to determine the problem the target audience is facing. It is then pertinent to formulate a solution to that problem, which will be outlined in step two of the process.
Step 02: Build a wireframe
The wireframe refers to the different aspects that need to be formulated, crafted, and then tested to result in the best possible web application outcome. Building a wireframe means creating the blueprint for the web app by taking into consideration resources, tools, frameworks, and iterations or testing phase, as well as determining the best development process.
Step 03: Determine the architecture
We’ve outlined our recommended frameworks for web application development, and this stage of the process is all about determining which of those frameworks will provide the best support for the problem you’re aiming to solve. Each architecture has multiple layers for consideration:
- Presentation; acts as the bridge between the browser and user interface
- Business; processes the browser requests
- Data access; transfers data with the servers for the business layer
- Data service; focusses on data security
Step 04: Design and develop the web app
Once the layers of the architecture have been outlined, it is time to shift focus to the user interface and highlight all the important touchpoints that users will mainly interact with on the web application. This process directly impacts the development stage and is thus very important. The other consideration to be made is regarding the navigations, as this too will impact the development decisions made. Once the architecture has been selected, the key features determined, and the user interface interactivity outlined, it is time to develop the web application.
Step 05: Test, iterate and deploy
The development process itself will involve coding, building, and integrating, until the web application has reached a usable state, by which time it should be tested. Many development teams choose to enact the agile approach to the development process which involves segregating the activities into sprints to streamline the process and maximise resources at each iteration stage. The goal is to develop a quality web application of the highest possible standard.
The important thing to remember is that the process does not end after step five of the development process. Once deployed, the web application needs to be continually monitored and maintained to stay up-to-date, relevant and keep its quality assurance promise.
The cost of web application development
There are several considerations that need to be made when determining the cost of web application development. Some of the factors that influence the cost include the complexity of the features you aim to have for your web app, as well as the level of experience you wish for your team of developers to have. Another consideration to make is where you wish to hire your developers from, as some countries offer more expensive development services than others. Determining the cost of web application development is so complex and unique to each client’s specific requirements and needs, that we’ve covered this topic in a whole separate blog. Read it here.
The future trend of web technology is clear: more users are heading online for solutions to their pain points, and more people are making use of smartphones and smart devices. While the adoption of web technologies is evident, it is simply not enough to have just any web application. With a growing market of web application products, it is pertinent to have a web application that offers high engagement and interactivity with a seamless design or else users may opt for a competitor’s product. The easiest way to avoid this is to partner with a design and development studio that has the experience and insight you need for a high-quality web application, so why not reach out to the Blott Studio team? Get in touch here.
Web application development has quickly risen to fame due to its benefits over mobile applications.
Web application development has quickly risen to fame due to its benefits over mobile applications. Web applications are compatible with all operating systems, allow for instantaneous updates without disrupting the user and offer a high level of engagement for a quality user experience. But something we’re often asked at Blott Studio is: what does web application development cost? It’s difficult to offer a set sum without knowing the full scope of the project and requirements you have for your specific web application development, though we can offer some insight into how different factors influence the cost thereof. This guide reveals the complexities of web application development and how different considerations can impact the cost of your final product.
When to consider a web application
Put simply, web applications combine the best of mobile applications with websites. They provide interactivity and functions similar to that of mobile apps while providing large quantities of information for the user in an easy-to-digest manner. Web apps are often considered to be a combination of dynamic websites and server-side programming that result in a high-quality user experience. Examples of web applications could be online banking, social media platforms such as Facebook, as well as eCommerce sites.
The best time to consider a web application for any business is when you aim to prioritise security and user experience, particularly in the form of customer support. Web applications are designed to provide higher levels of privacy and security for users. They also provide an increased level of customer support and accessibility to users, both of which drive brand loyalty.
For more benefits in considering a web application over alternatives, read Part 01 of our blog: A Guide to Web Application Development
The different types of web applications
We’ve reviewed the different types of web applications in-depth in our previous blog and, as mentioned, the main difference between the various types boils down to functionality and how you would prefer your web application to behave. We’ve summarised the five most common types of web applications, and how they could potentially impact the cost of development, below:
Type 01: Static Web Applications (SWAs)
Static web applications are as the name implies; they do not offer interactions for users and display information on a simple, clean interface that is easy to navigate. Their simplicity, and the fact that they use coding languages that are known to most developers, means that static web applications are one of the most cost-effective development options on this list.
Type 02: Dynamic Web Applications (DWAs)
If static web applications are on the cheaper end of the cost spectrum when it comes to web application development, then dynamic web applications are on the other end of the same spectrum. Dynamic web applications offer users a high level of interactivity on one or multiple web pages, that are processed at each user’s input request. This level of development is thus more complex and costly, though it is important to bear in mind that updating dynamic web applications is quick and effortless. The process of updating this type of web application is made easy due to the nature of the DWA not requiring server access, so updates happen instantaneously.
Type 03: Single Page Applications (SPAs)
Much like static web applications, single-page applications are a more cost-effective development solution. Single page applications are, as the name suggests, one web page that displays all the information, though it does incorporate some level of interactivity with elements on the web page that have changing displays. The most common examples of single-page applications are Gmail, Pinterest, and Netflix.
Type 04: Multi-Page Applications (MPAs)
Multi-page applications are web applications that mimic traditional websites in that they consist of multiple web pages that are all interconnected. When it comes to costing the different types of web applications, multi-page applications are considered more expensive due to the amount of development that needs to occur for each web page. These web pages not only have to display unique information and offer unique functionality but must also allow for interactivity between the various pages, which is naturally more time-consuming and labour-intensive compared to single-page applications.
Type 05: Progressive Web Apps (PWAs)
Progressive web applications can be likened to mobile applications, the main difference being that progressive web applications are accessed via browsers, while a mobile application must be installed on the mobile device. Progressive web applications can be more cost-effective than developing a mobile application as the development team does not need to develop separate native apps for different operating systems. It is however important to remember that progressive web applications can become expensive to develop as they offer the benefits of a website, as well as a mobile application; the complexity of the progressive web application simply comes down to the size of the app, as well as the level of interactivity you need.
The different levels of web applications
- Simple. Simple applications, sometimes referred to as basic applications, are web applications that are made up of any combination of landing pages, single web pages and/or simple eCommerce. Some examples of simple web applications may include calculators, digital catalogues, or widgets. These are all considered the “entry-level” of web application development.
- Professional. Professional web applications are considered “mid-level” web applications and are those that have a higher volume of content and require more interactivity. An example of professional web applications is online stores that provide more information about the products and allow functionality to make online purchases.
- Complex. Complex web applications, or large-scale web apps, are the top level of web application development, not only because they are made up of the largest amount of content and more functionality than simple or professional web apps, but because they can manage high volumes of users at a time without compromising consistency or functionality.
Factors that affect web application cost
Now that we’ve reviewed the different types and levels of web application development, and how they might impact cost, we need to consider the various factors that can also influence the cost of your web application development.
The project scope
A good starting point to estimate the cost of web application development for any given project is to outline the scope of work (SOW). It’s important to determine the functionalities or features of your ideal web application, your target audience size, and the purpose this web application will aim to serve. You may want to give thought to the type of web application you feel best suits your needs, the level of interactivity you require if the scope should extend to include mobile application development, and potential deadlines. As part of this process, you should also review the stages of the software development lifecycle:
- Discovery. The discovery phase refers to the research you conduct to determine the business strategy, requirements, and cost estimations of the project. It is also during this time that a prototype can be created to understand the technical input required for success. Partnering with the right development studio that has qualified, experienced project managers to assist you through this process ensures that you receive all the information you need to make a well-informed decision on the next steps.
- Design. The next phase is to spec the prototype and this happens with the assistance of UX and UI designers. Their role is to design the web application user interface, determine your target audience’s expectations and incorporate the business goals you have previously outlined to create the blueprint for the best possible web application.
- Development. During this phase, your development team sets to work. It is their responsibility to create the front-end and back-end of the web application through strategic software engineering that meets the business requirements. It is also during this phase that iteration takes place to ensure that all the web app’s features are operational.
The developer’s location and skillset
One of the main factors that will influence the cost of web application development most is the development team you employ to deliver the final product. Two important considerations to be made are the geographical location of the developer, as well as their level of expertise. Naturally, certain countries’ hourly rates are higher than others, and a developer with more experience and a broader skillset will be more expensive than someone without that level of expertise. The second consideration to be made regarding your development team is how many experts you will need to work on the project. It’s important to take the time to research developers’ rates and compile the skillset you will need fulfilled in your development team or outsource this function to a development studio that can propose the best team for you.
The UI/UX design complexity
The complexity of your web app design is directly proportional to the cost thereof. On the one hand, if you opt for a single-page web application that mimics an existing design with no customisation, then the exercise will be relatively inexpensive. On the other hand, a progressive web application with high customisation and many additional features will be a costly exercise. Regardless of if you choose simple or complex, your web application should always prioritise user experience. A web application that is difficult to navigate and isn’t optimised will mean a failed launch, regardless of the design path you’ve chosen.
Maintaining the web application
Every web application requires ongoing maintenance and support. As technologies change and businesses scale up, web applications will require updates and additional development to support that company's growth. Debugging, systems updates, new integrations, additional features and security checks and updates (amongst others) are all part of ongoing maintenance, so be sure to include the relevant agency costs associated with your web application development.
Potential hidden costs
Though not always the case, hidden costs may arise throughout the web application development process, or during the annual maintenance of your product. Two such hidden costs could be potential cyber threats or subscription costs for elements of your design. Unfortunately, cyber threats are a very real reality in the digital realm and protecting your users’ data is of paramount importance. In some instances, you may be required to consult security professionals or enlist additional security support to secure your web app. In the case of subscription costs, some design and development tools that offer templates or plug-ins may involve subscription costs that could be adjusted further down the line or implemented for certain customisations. One way to avoid subscription costs is to partner with a high-quality developer who can develop functionalities rather than employ plugins. Either way, be sure to discuss these potential hidden costs with your development studio for further clarity.
Selecting the right support team for your web app
The next step to determining the cost of web application development revolves around the type of support team you will employ. Businesses can opt for an in-house team, a freelancer or outsource the function. Each type of support team has its benefits and only you can decide which of these will best support your goals. We’ve summarised a few of the advantages and disadvantages of each below:
In-house
The first option is to employ a dedicated team of developers to form part of your company operations. They would be permanent employees of your organisation, adhere to your code of conduct and have a clear understanding of business operations, your goals, and the company vision from an internal viewpoint.
- Pros. The biggest advantage of an in-house development team is that you would have full control over their activities. An in-house team also allows for face-to-face engagements as they would operate from the same workplace.
- Cons. The process of hiring developers is time-consuming and will be costly as the resultant employees would be permanent staff. Another drawback is that your company would be entirely dependent on the in-house development team and, as a result, may risk skillset gaps or extended deadlines on projects.
Freelancers
Hiring freelancers is considered the cheapest option for your design and development needs. Freelancers are thus a good consideration for businesses with a limited budget. They are also easy to find due to the wealth of online resources for hiring freelancer web app developers. Choosing a freelancing team also opens the opportunity to hire remote-working individuals that may be based in a different country than yourself, to assist in offsetting costs.
- Pros. The advantage of hiring freelancers is that, as with an in-house team, you would still have control over their activities, they would simply no longer be under your employ once the project has been completed. This is another reason why hiring freelancers is more cost-effective than hiring in-house.
- Cons. The disadvantage of hiring freelancers however lies with the quality of work deliverables, which is entirely dependent on the individuals hired, but also in that managing a freelancing team is a time-consuming role that someone within the organisation would need to fulfil.
Out-source
Out-sourcing software development is a booming market and it’s easy to understand why. Out-sourcing guarantees a high level of work output as design and development agencies are experts in their field and hire the most qualified individuals to form part of their support teams.
- Pros. Out-sourcing may prove to be more cost-effective when enlisting services from a different country (if these same services are considered expensive in your region). You are also granted access to web app developers who are highly skilled and experienced and are managed by development professionals who understand the intricacies of software development. Out-sourcing thus provides the best probability for high-quality final products.
- Cons. Out-sourcing can be a more expensive option based on the skillset and experience of the design and development studio you choose to work with.
In-house teams are especially valuable to large organisations that require ongoing software development support, while freelancers are typically recommended for smaller tasks and projects that have a quick lead time. The drawback of both, however, is that you would not be guaranteed a full skill set for all your development requirements and these teams would need to be managed by the company itself which leaves room for a gap in an expert opinion that is vital for a high-end web app. Considering outsourcing for new web application development ensures that you have the best combination of experience, skillset and expert opinion.
Selecting the right tech stack for your web app
Now that you’ve determined your support team and have an idea of how your web application should look and function, you need to turn your attention to the tech stack. A tech stack refers to the technologies incorporated into the design and development of your application. This would include the front-end, back-end, data management, design of the app, and the like. While our recommendation is to chat with your development agency concerning the best solutions, here are three tips to help you decide on your powerhouse combination:
- First and foremost, start with research. Delve into your target audience and what their pain points are, discover applications that would be similar to your offering, and outline any additional features you want your web app to possess for a competitive advantage.
- Next, spend some time researching different tech stacks’ reviews and communities, as well as what level of support they offer developers.
- Once you have a better idea of the above you can begin selecting your tech stack and determine if its capabilities and processing power will support your web app needs.
Incorporating an understanding of software development and the factors that influence the cost of development is an integral part of the web application planning process. In this guide, we’ve covered a range of options when it comes to the type and level of web applications that may have helped clarify the direction you wish to take for your web app. We’ve also highlighted the various factors that form part of the cost consideration. Now you can recognise the elements that make up the cost of web application development and approach the development of your web app with a better strategy. The next step is to partner with the right design and development studio that can assist you in the creation of a high-end product that prioritises user experience. Chat with us, here.
Artificial Intelligence (AI) is the creation of machine intelligence that replicates human thought and behavior. In layman’s terms, AI is essentially the ability of machines to think for themselves and act independently.
Artificial Intelligence (AI) is the creation of machine intelligence that replicates human thought and behavior. In layman’s terms, AI is essentially the ability of machines to think for themselves and act independently. Technologies have been evolving at a breakneck pace ever since Alan Turing first mentioned the concept in 1950. The emergence of AI has been one of the most dynamic developments in modern technology. And, with its increasing relevance in a range of fields from healthcare to finance to retail and beyond, it’s only a matter of time before artificial intelligence becomes as ubiquitous as electricity or mobile phones.
What is Artificial Intelligence?
Artificial intelligence is the science of computer systems that can perform tasks that we associate with human intelligence, such as learning from data, problem-solving and understanding language. AI research focuses on creating machines that can simulate the cognitive functions that we associate with human beings. Rather than replicating human thought, AI tries to understand the underlying principles that make up human cognition. For example, AI can help us to understand the human desire for predictability and plan for the future or analyse data and draw conclusions.
How does Artificial Intelligence Work?
Artificial intelligence can make sense of data. That’s why AI is often compared with a ‘computer trying to understand human language’. To understand data, AI uses a process called ‘learning’ to decipher patterns and create its own outcomes. As it learns, AI creates outcomes that mimic human thought. For example, if you train an AI model to recognise cats, it will figure out that ‘red and white cat’ means ‘cato’ and ‘black cat’ means ‘ruffus’. AI uses algorithms to analyse data and model outcomes. Some of the most common algorithms used in AI include: - Probability-based model: In this model, the AI predicts with a certain percentage of certainty. - Machine learning model: This model involves training the AI with large amounts of data and then using the feedback to refine its prediction. - Predictive model: In this model, AI learns to predict future outcomes based on past data.
Types of Artificial Intelligence
- Decision AI: Artificial intelligence that helps us to make decisions.
- Collaborative AI: This is a type of AI that allows humans and machines to work in unison. It could be used for the automation of tasks or for human-machine interaction.
- Conceptual AI: This is an AI that can understand the abstract concepts of language.
- Emotion AI: This simulates human emotions like happiness, sadness, anger and so on.
- Planning AI: AI that aims to help us plan our future actions.
- Language AI: This is about AI that can understand human language.
- Natural Language AI: This is about AI that can understand human language.
- Numerical AI: This is about AI that can understand numbers.
- Social AI: This is about AI that can understand human social behaviour.
- General AI: This is about AI that can be used to create a ‘superhuman’.
- Procedural AI: This is about AI that uses algorithms to accomplish tasks.
Machine Learning vs Deep Learning
Machine learning and deep learning are two major approaches to artificial intelligence (AI) development. Machine learning is a subset of AI, which is based on the premise that computers can learn from data without being explicitly programmed. Deep learning is a more advanced form of machine learning that is based on the principle that a neural network can learn complex tasks by “deeply” understanding the input data. Both machine learning and deep learning have their own advantages and disadvantages. Machine learning is often faster and easier to use than deep learning, but deep learning can be more accurate and efficient at tackling certain tasks. Machine learning is also better suited for tasks that are simple in nature, such as recognizing text or images, while deep learning is better suited for tasks that are more complex, such as recognizing objects in images or speech.
Overall, machine learning and deep learning are two powerful AI tools that can be used to improve the accuracy and performance of various applications. It’s important to choose the right approach for the task at hand, so be sure to evaluate both machine learning and deep learning before making a decision.
Uses of AI in day to day life
AI can be used to solve a variety of issues in our daily life. It can help to automate tasks, create customer-friendly experiences and make sense of huge data sets. AI can help in decision making by providing insights into customer behaviour and behaviour. It can also help to predict outcomes and manage risks, thus making complex business operations more efficient. Some of the common uses of AI in day to day life are mentioned below -
- Customer behaviour analysis: AI can help us to understand customers better. The data sets of customer behaviour can be used for identifying customer needs and designing better products.
- Automated decision making: Decision making can also be automated with the help of AI. It can help to make complex operations more efficient like managing large data sets, understanding trends, predicting outcomes and managing risks.
- Customer service: AI can also be used to make customer service more efficient. It can help to automate the process and provide personalized experiences.
- Fraud detection: AI can play a crucial role in detecting frauds. It can help to analyse large data sets and detect patterns to detect frauds.
- Regulatory compliance: AI helps to manage regulatory compliance. It can be used to manage large data sets, understand reasons behind compliance issues and make operations more efficient.
- Predictive maintenance: Predictive maintenance is one of the most important uses of AI. Predticitive maintenance helps to schedule maintenance activities accordingly. It also helps to forecast failure of machines and manage risks.
- Data science: Data science is one of the most important uses of AI. Data scientists work on analysing huge data sets, making sense of data, creating algorithms and managing risks.
- Speech to text: Natural language processing is one of the key components of speech to text AI. It is used to convert human language to machine language. Speech to text can be classified into two types - Continuous speech recognition and keyword recognition. Keyword recognition is used to understand words like ‘cat’, ‘dog’, ‘motor’ and so on. Continuous speech recognition is used to understand continuous sounds like ‘ma’, ‘ga’, ‘ta’ and so on.
Benefits of AI in Business
AI can help to make operations more efficient. It can help to automate tasks, manage risks, predict outcomes and make sense of data. AI can also help to make decision making more accurate. It can help to reduce costs, increase efficiency and increase revenue. AI can help to make operations more customer-friendly by understanding customer behaviour and delivering personalized experiences. It can help to make operations more sustainable by managing risks, predicting failures and scheduling maintenance activities. AI can be used to make marketing more effective. It can help to make better use of data, create innovative products and make sense of huge data sets. It can be used to automate business processes. AI can help to make processes more efficient, make operations more reliable and help to make work forces more productive.
Role of Artificial Intelligence in future?
AI will be a key driver of change in future. It will play a crucial role in processes like decision making, risk management and operations. AI can be used to analyse huge data sets, make sense of data, manage risks and make operations more reliable. It can help to make operations more sustainable, create new business models and automate processes. AI can help to make operations more efficient, make sense of data, manage risks and make decision making more accurate. It can help to make operations more customer-friendly and can also help to make workforces more productive. It can also be used to make marketing more effective and can help to create innovative products.
Artificial intelligence is likely the biggest contributor to the disruption of the fintech space in recent times. Traditional financial services have been buying up AI-powered tools and technologies to help them move into fintech as they aim to launch their own fintech ap
Artificial intelligence is likely the biggest contributor to the disruption of the fintech space in recent times. Traditional financial services have been buying up AI-powered tools and technologies to help them move into fintech as they aim to launch their own fintech apps in the industry to remain relevant. Incorporating AI into their fintech products helps their customers manage their finances more efficiently, in a convenient and safe environment. These AI-powered fintech products also provide valuable insights that help financial institutions innovate and provide improved services to their clients.
It’s no wonder then that artificial intelligence is taking the industry by storm. So, what are the different ways these financial institutions are using AI, and how can you leverage this emerging technology that is undoubtedly here to stay?
Read on for 10 ways to incorporate AI into your fintech product.
1. Security
Artificial intelligence offers improved methodologies for better security measures, which are invaluable for businesses operating in the financial services space. The financial industry is particularly plagued by cyber risk, and AI and machine learning tools offer fintech the best opportunities to protect their user’s information and wealth.
One such opportunity is the use of AI models for chatbot integration that assists fintech users in recovering lost passwords and providing additional access. Identification via facial, speech and fingerprint are other security measures that present security opportunities to fintechs. Traditional passcode methods are more susceptible to cyber threats by hackers than personalised identification tools, which make your fintech product more secure and trustworthy.
2. Fraud Detection
Cyber risk isn’t the only threat to financial services. Credit card and loan scams, and fraudulent insurance claims are some of the other risks posed to fintech. Financial businesses are also required to balance customer experiences and maintain a positive reputation, as well.
Artificial intelligence is a great tool for enhanced cyber security to combat fraudulent activity. Specialised systems and algorithms can be implemented to monitor transactions and flag any suspicious or abnormal behaviour. A process that would otherwise require a substantial workforce as it is time-consuming and labour-intensive. AI makes fraud detection a simplified, streamlined process, allowing fintechs to react quickly and efficiently to correct fraudulent activity.
3. Algorithmic Trading
Algorithmic trading conducts stock trades by relying on pre-programmed instructions that help the system identify trading decisions and report on that data. This machine learning tool has been around since the 70s but has seen some recent upgrades since the introduction of AI.
Compared to older models, the artificial intelligence model has the capability to analyse millions of data points and provide keen insight to traders, all in real-time. This enhanced version of algorithmic trading is faster and more precise than older statistical models, thanks to its ability to identify further insights than previously possible.
What’s more, AI-enhanced algorithmic trading models are still progressing, and we’ll likely see more efficient iterations in future that are able to identify trading trends, react with relevant market trading, and pinpoint anomalies to take corrective action. All made possible by AI in fintech.
4. Robotic Process Automation
We’ve touched on chatbots as a front-end security measure for fintechs, but businesses operating in the financial services sector will be all too familiar with the back-end operational demands, and that’s also where chatbots, specifically robotic process automation (or RPA) can assist.
RPAs can automate a range of tasks, such as customer onboarding, inbound calls for general queries, as well as security checks, all tasks that are more client-facing. They are also able to process routine operations, such as loan applications and account information pertaining to credit cards or the closure of accounts. This integration helps fintechs save time and valuable resources to focus on the parts of the business that need their attention.
5. Predictions
In the past, if financial services businesses wanted to analyse their historical data and report their findings, they would have to deploy teams to dedicate extensive amounts of time and resources to interpret the information. Artificial intelligence and machine learning solutions have revolutionised decision-making for fintechs, as they are capable of processing large amounts of data in a short period of time to produce accurate insights that are actionable.
Credit scoring and loan predictions are some of the top financial technology developments in recent times, thanks to the capabilities of AI. Financial institutions are better able to analyse clients’ credit scores to determine their risk and approve loans faster. They’re also able to make improved decisions regarding market trends.
6. Data Automation
Credit scoring is a valuable tool for understanding whether a client is capable and/or willing to repay loans. Artificial intelligence integrations for data automation, particularly in the field of credit scoring, are considered one of the most useful fintech product incorporations.
Credit scores are based on a statistical model that interprets multiple data points to provide insights. AI enhances this function by combining those data points, as well as further information such as income, credit history, and transactions, to relay individual credit assessments that are based on real-time factors. This provides financial institutions with accurate data analysis to make less risky decisions when it comes to providing loans. Not to mention that the accuracy of AI data automation makes providing financing to a broader audience safer and more accessible.
7. Analysis of User Behaviour
Artificial Intelligence Application Programming Interfaces (or AI APIs) are just another product integration that is powering the future of fintech. AI uses predictive analysis to determine user behaviour and respond accordingly by providing the information the user will likely request next.
AI achieves this by combining artificial intelligence and machine learning models so that users can better understand their data with detailed insights. Data automation not only processes the user’s initial requests but pre-empts the next request and provides that data at the same time, making the fintech product more efficient while minimising the number of input requests.
8. Customer Service
The digitalisation of services and artificial intelligence have made customers accustomed to fast, 24-hour service. The threat this poses to financial services is the possibility of losing those customers when instant gratification cannot be provided.
Artificial intelligence techniques like chatbots and virtual assistants can provide support to customer service (human) teams by responding to commonly asked questions and handling inquiries that can easily be automated. This allows customer service centres to focus on resolving concerns customers may have or tend to specialised queries.
Advanced sentiment analysis is another AI tool that seeks to understand the customer and solve their pain points, leading to retained users, referrals, and increased profits for the fintech.
9. Personalisation
Personalised services are a great advocate for brand loyalty, but the problem financial institutions face is the large number of customers they serve. Providing services that are tailored to the individual would be all-consuming, and in many instances impossible, if not for artificial intelligence.
Artificial intelligence processes user data to provide insights, make predictions and analyse behaviour, and it can use that same data to make suggestions to the user on how to tailor their fintech app to suit their needs, as well. This could take the form of financial advice, tracking metrics, or recommending product features.
10. Cost Savings
Artificial intelligence not only streamlines procedures and makes them efficient, but it also helps fintechs save capital, labour-related costs, and valuable resources. AI solutions take over routine operations that would otherwise be managed by human counterparts, so they can shift their focus to other areas of business. Artificial intelligence models are also more effective, quicker, and accurate, making AI a valuable resource for cost-reducing activities.
AI technology can be deployed across a range of business activities, as we’ve already seen throughout this article, and may take care of tasks such as providing accurate data and insights, recognising and reporting fraud or laundering, and underwriting credit, to name a few. This support means that financial institutions can offer improved services to their clients, saving costs but increasing profits.
One of the more recent use cases of AI in fintech that has caught our attention, is that of Goldman Sachs acquiring Clarity Money, a personal finance app that uses AI and machine learning algorithms to help users manage their finances, in 2018. This acquisition led to the development of Goldman Sachs own product: Marcus by Goldman Sachs. The very same digital banking platform that powers Apple Card, who have been in the news recently for offering a 4.15% return on cash in their savings account. Most banks offer a return of 0.01%, so this is big news and a very clear clue to how the incorporation of AI into fintechs is driving innovation and growth for the industry.
Artificial intelligence has progressed the fintech space leaps and bounds in a short period of time, offering startups and established financial service businesses alike opportunities to save costs, become more efficient and deliver an improved customer experience. The overarching goals of AI in fintech are to improve productivity and drive profitability, something that is readily achievable thanks to AI’s features like automation and predictive models.
Identifying key opportunities to incorporate AI into your fintech is a winning strategy that can only be supported by a team that understands, and can implement, AI-powered tools and techniques for your product. Reach out to Blott Studio’s team of developers to chat about artificial intelligence for your business.
Artificial intelligence is driving innovation in the fintech industry by making financial services more efficient, faster, and more reliable. AI-powered apps are spearheading business growth and improved user experience within the fintech industry, and these creations are
Artificial intelligence is driving innovation in the fintech industry by making financial services more efficient, faster, and more reliable. AI-powered apps are spearheading business growth and improved user experience within the fintech industry, and these creations are paving the way for the future of fintech. We’ve seen startups flourish with the aid of AI and machine learning capabilities in data analysis, watched apps develop new payment solutions, and seen innovative personalization integrations accelerate brand loyalty.
We take a look at artificial intelligence in the financial services industry and the future of fintech, thanks to AI.
How AI drives innovation for the fintech industry
Predicting the future is a tough ask, but there are a few trends to take note of that are already emerging, and that we feel will drive innovation for the fintech industry in the coming years. It is however important to keep in mind that the fintech industry is an ever-evolving space, and we’ll likely see new developments being brought to light as the financial services sector and artificial intelligence technologies continue to merge. Nevertheless, here are a few ways AI is driving innovation for the fintech industry:
Transfers and Payments
Fintech, as the contraction for “financial technology”, has changed the way traditional financial institutions offer transfer and payment solutions to consumers. Incorporating AI into fintech products will undoubtedly give rise to new and improved ways for users to employ mobile banking services, action payments, and conduct peer-to-peer transfers and/or payments.
Cryptocurrencies
Blockchain and crypto have the potential to change the way the world manages its finances and has opened the door to new forms of currency and ways to transact. Cryptocurrencies have already begun to revolutionise the financial industry, and AI will continue to drive innovation in this field. From new blockchain applications to market growth, there are many ways in which artificial intelligence could impact blockchain and cryptocurrencies.
Artificial Intelligence and Machine Learning
AI and machine learning have the capability to make fintech products more efficient and provide an improved user experience. Artificial intelligence models have already begun to infiltrate automation processes in fintech, and we think this is an area where the marriage of these technologies will continue to greatly benefit and drive customer service.
Interested in the specific applications of artificial intelligence in fintech products? Check out our blog: 10 ways to incorporate AI into your fintech
How AI benefits the fintech industry
Machine learning models have been utilised in the fintech industry for some time now and, while they have provided valuable data analysis and predictions, incorporating artificial intelligence into these models has made them more efficient and more accurate. That’s not to say that machine learning algorithms don’t still have their place in the fintech sector alongside AI. In fact, some fintechs have incorporated both technologies for their combined benefits. These fintechs make use of AI and machine learning in the following ways:
User Experience
Advanced sentiment analysis, AI-powered chatbots and virtual assistants are just some examples of how AI is enhancing the customer experience. AI and machine learning algorithms have the ability to provide personalised experiences by analysing historical data to make recommendations. AI-powered chatbots are also programmed to answer common questions immediately so that human support services can concern themselves with more complicated queries and tasks.
Automation
Analysing data and providing insights is a superpower of artificial intelligence tools. The fintech industry is benefiting from this superpower for all kinds of data analysis from credit scoring to user behaviour. This functionality not only saves fintech companies time and money but also minimises risk, as artificial intelligence algorithms have a higher accuracy score than their human counterparts.
Decision-Making
Having touched on the capabilities of AI and machine learning when it comes to data analysis, it’s no surprise that improved decision-making would be on our list of benefits. Artificial intelligence and machine learning algorithms process millions of data points to provide insights and make predictions. The patterns these models identify can then be used to empower decision-making.
How AI enables fintech integration with other industries
The integration of artificial intelligence and machine learning in fintech is not only benefiting the financial services industry but others as well. Embedded finance is a fast-growing trend that is enabling better financial services for several sectors so that consumers have a convenient way to manage their finances.
Embedded finance is the process of integrating financial services into business products and services with the use of Application Programming Software (API). It comprises four pillars: payments, banking, lending, and insurance. The great thing about embedded finance is that these businesses are not regulated in the same way as financial institutions, and businesses have the capability of keeping payments in their own ecosystem.
E-commerce retailers are one common use case of embedded finance. E-commerce stores integrate payment infrastructure within their applications, so users can action payments easily, without needing to be directed to a different platform. This integration ensures efficient service that improves the user experience. Another example is that of embedded credit whereby users can apply for loans and action repayments all within a mobile app.
How AI in the fintech industry is being regulated
The introduction of technological advancements is generally shrouded in controversy and concern. In the case of artificial intelligence, particularly so. Fintech regulations were initially created to instil balance and equal opportunity between financial technology and traditional financial institutions and to drive innovation for the financial sector. These same regulations are being used to monitor the use of AI in fintech, as well.
The three main regulators are Financial Conduct Authority (FCA), the Payment Services Directive (PSD2), and the Directive on Markets in Financial Instruments (MiFID II). Together, these regulations provide a controlled environment for fintechs to test their AI-powered apps, enforce rules for the use of AI in payment solutions and the financial market, and allow for the development and access to services and markets, respectively.
How AI in fintech continues to impact society
Fintech in general continues to have a significant impact on society and the way people move about their day. From managing their finances to paying for goods and services, and education on financial markets, fintech has made finances more convenient, more accessible, and more affordable to the global population. Incorporating AI into the fintech sector has further impacted society by providing more users with access to financial services, increasing competition within the financial services industry, and stimulating economies. Indirectly, AI in fintech has empowered users to make financial decisions, improved the quality of those services, and created jobs within the financial services industry.
On a grassroots level, AI-powered fintech has had a positive impact. Underserved communities have previously not had access to traditional banks within their immediate surrounds and thus faced the challenges of travel and time to manage their finances and action payments. Fintech products have resolved this issue as users living in these communities now have mobile app access to those very same services. Personalisation services also grant better services to underserved communities in the form of products such as microloans and low minimum balance savings accounts; tools which help empower and provide financial control.
Artificial intelligence is disrupting the fintech space and has become an ingrained part of fintech app development. Financial institutions will need to adapt and consider the benefits of utilising AI in their own products to remain relevant in a highly competitive market. Automation, machine learning, payment solutions and personalisation are just some of the trends to emerge from AI-powered solutions. Financial institutions that are most likely to succeed in this evolving ecosystem are most likely those who are adapting and taking advantage of artificial intelligence technologies. AI has transformed the fintech space in many ways and will continue to do so. Want to learn more about AI’s fintech capabilities? Connect with our team of developers.
Elevate your design with storytelling techniques. Learn how to use context, emotion, and visuals to captivate your audience and create a memorable brand experience.
First impressions matter. They always have. Whether face-to-face or virtual, a good first impression can be the difference between winning or losing business. But now? Instead of 5 minutes, you get 5 seconds. Max.
We’re living in an online world with masses and masses of media at our fingertips. Information is at an all-time high and concentration at an all-time low. There is so much stuff on the web. Users are either going to engage with what they care about, or scroll right past.
That’s why it’s important to create “thumb-stopping” content.
What’s that? The name says it all. It’s attention-grabbing content that causes the user to stop scrolling. The easiest way to get someone to focus on your content? Connect with them. Really connect with them.
Stories are a great way to do this. And I’m not referring to Instagram here. From the earliest recorded history, storytelling was a method used by cavemen to share, communicate and entertain members of their tribe. The better the story, the better the engagement. Huh, maybe I am referring to Instagram. Who doesn’t love a good narrative though?
Stories are still around. They just look different. We’ve swapped cave paintings and fairytales for books and movies. But it doesn’t end there. Stories are told through colours, shapes and layouts too. That’s right; messages can be communicated through visual elements. And they are, all the time. If a designer gets the intended message across effectively, then it’s a great story; no less than the one from a writer.
So, as a designer, how can we tell a story?
Focus on the user. Not the product.
Get to know your target audience. It’s important that you find out what it is they care about. That way, you’ll understand their circumstances and be able to create something that says, “This company? It’s tailor-made for people like you." Of course, without using those words.
Like Airbnb, for example. When Airbnb expanded to include the business traveller, they envisioned a suit, briefcase and busy work schedule. Were they right? Nope. The modern-day business traveller wears comfy clothes, travels with a laptop and makes time for play. Luckily, Airbnb figured this one out on their own. So, they built an experience for people who enjoyed travelling for work, wanted to embrace the local culture and got a kick out of exploring.
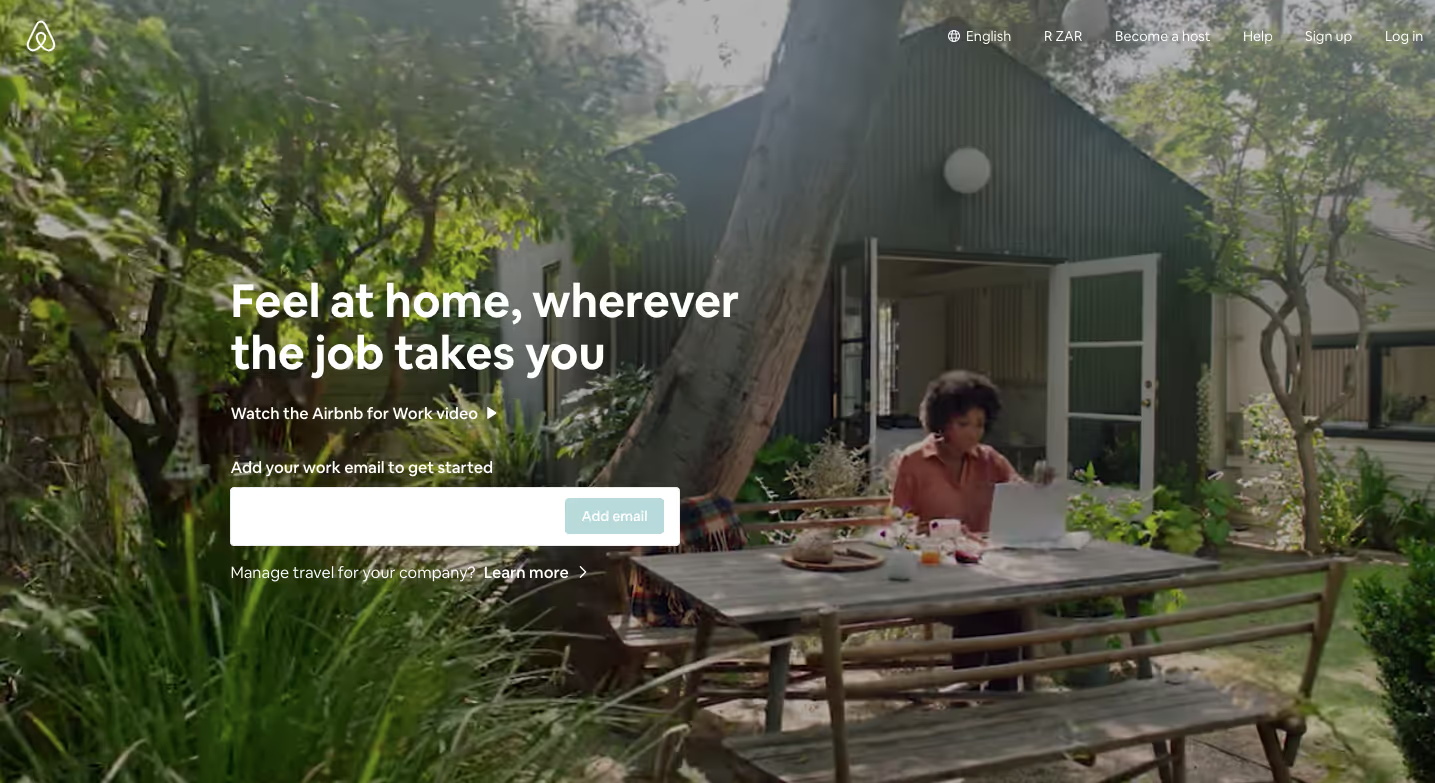
Context vs Content
We’ve all heard the saying. “If content is king, context is queen.” Context is what makes things valuable to the reader. Or, adversely, meaningless. If you understand the context of a user’s situation, you’ll be able to approach it with the right messaging. And if you can do that, then you’ll be a central element in their own story.
Tradition’s out. Emotion’s in.
Ever heard of a creative license? Well, it’s yours. Use it however you like. But if there’s one thing you aim to do, it should be to evoke emotion. Your choice of photography, typography, tone of voice and UI elements can say as much as a full story, if not more. And that’s what people will remember your brand by.
See this airline company? This is what we’re talking about. Their choice of information architecture, typography and imagery takes you on a flight over the Swiss alps. Just for a few seconds.

How about a brand mascot?
Hear me out. Loads of companies are using brand mascots. The Michelin Man, Rich Uncle Pennybags from Monopoly, or even Mr Muscle. Brands that give a face to their company seem to stay at the top of consumers’ minds. Is it because these mascots act as spokespersons and storytellers? Probably. And people love it!
Think of Headspace. Their funny-looking creatures guide you on a journey to mindfulness. They look pretty peaceful. So it must work, right? It’s random. But without them, it wouldn’t be Headspace.

Vision for a better future
“The perfect world? It doesn’t exist. Yet! But with our brand, you’ll be one step closer to it.” This is one of the most successful storytelling approaches. People are trying to do better. So encourage them, with your brand.
In this Ice And Sky homepage, the viewer is taken on a visual and educational journey. How? Through a variety of historical media and animations. It’s inviting. It’s righteous. And it even looks like a storybook.

Good design is like…
A well-written book. All the words have been carefully chosen to guide the reader on a journey from start to finish, ending with an overall message. It’s the same for designers. If the viewers get the message, it means it’s been effectively communicated. And that’s what makes a design a good one.
Are you considering outsourcing a development team but unsure if it's the right fit for your business? Here's everything you need to know to make informed, strategic decisions about outsourcing.
Balancing Innovation and Costs in Modern Business
In today’s fast-paced business landscape, particularly for startups, finding the right balance between innovation and budget management is a significant challenge. Companies often ask: How can we develop competitive products while keeping expenses in check?
Outsourcing a development team is one effective answer, though it offers more than cost savings. It provides flexibility and access to a global talent pool, enabling businesses to scale, diversify expertise, and stay agile.
The market for outsourcing continues to grow, driven by demand for flexible and skilled resources. According to a report by GlobeNewswire, the industry is on track to expand by $98 billion between 2020 and 2024. Many businesses, from small enterprises to large corporations, leverage outsourcing to expedite development while cutting overhead costs.
Understanding Outsourcing in Development
Outsourcing a development team involves engaging an external team of developers, designers, or project managers to execute specific software tasks or entire projects. Whether you need help with a short-term project or a dedicated team for ongoing development, outsourcing allows you to tap into expertise your internal team may lack, enabling high-quality outputs without increasing headcount permanently.
Benefits of Outsourcing Development Teams
Businesses choose to outsource for several strategic reasons, which include:
- Cost-Efficiency: Reduce expenses associated with full-time employees, such as benefits and office space.
- Global Expertise: Outsource to specific fields like mobile app development, front-end, or back-end expertise.
- Scalability: Adjust the size of your development team based on project scope and changing demands.
- Quicker Market Access: Outsourcing accelerates product delivery by providing immediate access to qualified teams.
Outsourcing Models: Choose the Best Fit for Your Needs
Before outsourcing, it’s essential to select the right model. Here are the main options, each with its benefits:
- Onshore Outsourcing: Collaborate with teams within your country for fewer time zone and language challenges, though it may cost more.
- Nearshore Outsourcing: Work with teams in nearby countries for moderate cost savings and easier communication.
- Offshore Outsourcing: Partner with teams in more distant countries to achieve significant cost reductions, though time zone and communication may require added coordination.
When Should You Consider Outsourcing Development?
Here are key signs that outsourcing may be the right choice for your business:
- Lack of In-House Expertise: Outsource specific technical skills your team lacks, such as mobile or specialised backend development.
- Budget Constraints: Save on salaries, benefits, and workspace costs.
- Project Deadlines: Scale resources with external support to meet tight launch schedules.
- Complex Project Needs: Outsource complex tasks across multiple areas like design, testing, and coding to ensure smooth project execution.
Real-World Success Stories in Outsourcing
Many industry leaders have successfully scaled their business with outsourced development teams. Examples include:
- Skype: Initially outsourced development to a skilled team in Estonia, enabling rapid product iteration and a path to global success.
- Basecamp: Utilised outsourcing for initial product development, gaining traction faster without sacrificing quality.
- GitHub: Outsourced early software development to scale its platform effectively, meeting demand during growth phases.
Key Steps to Outsource Development Effectively
To ensure successful outsourcing, take the following strategic steps:
- Define Your Outsourcing Model: Decide whether onshore, nearshore, or offshore best suits your project goals and budget.
- Select the Right Partner: Platforms like Clutch or Toptal allow you to review portfolios, client feedback, and specialisations to find a suitable partner.
- Set Clear Objectives: Define your project goals, establish communication protocols, and maintain regular check-ins to align efforts.
- Utilise Project Management Tools: Use tools like Trello, Jira, and Slack to keep both in-house and outsourced team members on track and aligned with project objectives.
Overcoming Outsourcing Challenges
While outsourcing offers numerous benefits, it also presents some challenges. Here’s how to address the most common ones:
- Quality Assurance: Set clear quality benchmarks and conduct regular reviews to ensure expectations are met.
- Communication Hurdles: Overcome time zone and language barriers with regular scheduled check-ins and collaboration tools.
- Security Risks: Protect sensitive information by ensuring your outsourcing partner follows best security practices and limits access.
Consider a Membership Plan for Dedicated Development Support
If you need consistent access to high-quality development resources, a membership plan like Coconut Lab’s Unlimited Membership Plan could be an ideal solution. Designed to give you access to a skilled team of over 20 professionals, this plan offers flexible, on-demand expertise without full-time costs.
Key Membership Plan Benefits:
- Cost-Effective Access to Expertise: Gain access to top-tier talent, including developers, designers, and marketing experts, all at a fraction of the cost of in-house teams.
- Scalability and Flexibility: Easily adjust resources based on current project needs, ensuring you only pay for what you use.
- Dedicated Account Management: A dedicated manager oversees project efficiency and aligns deliverables with business goals.
Conclusion: Is Outsourcing Right for Your Business?
Outsourcing development teams isn’t merely a cost-saving strategy; it’s a growth enabler that provides access to global expertise and allows businesses to stay flexible. Whether you’re a lean startup or a well-established company, outsourcing can offer the competitive edge needed to innovate, scale, and succeed.
Design an API that's built for the future. This guide provides actionable insights on creating scalable APIs that prioritize user experience, extensibility, and long-term success.
Bigger is not always better. Add more resources to a company, and its revenue may increase. That’s growth, and it’s good. But increase a company’s revenue without adding significantly more resources? That’s scale, and it’s even better.
We’ve heard the term “scalability” thrown around a lot in tech companies. It’s more than a 2020 buzzword. As applications grow exponentially larger and more complex, API providers need to adapt their interfaces. Why? Simple. To meet the increased user-focused demand. They need to do this while managing configuration though.
As an organisation scales, the design of their API should be equipped to handle the increased traffic and satisfy user’s requests. It’s important that the software is programmed to process one million requests with the same efficiency as one hundred requests. Its infrastructure should also be built with scalability in mind, allowing different software engineers and teams the ability to work alongside each other in a harmonious fashion. But, how?
It’s all in the design.
Any software developer knows how easily projects can devolve into unstructured, tangled webs. That’s why internal consistency is key; interfaces are being designed for other programmers to build on. Plus, programmers are usually pretty tech-savvy, so any flaws will be pointed out.
Predicting every future use and application of their service is impossible for API providers, especially once the company begins to scale. Designing an API that’s extensible will come in handy later. Why? Because it’s adaptable.
This will make it easy for multiple extensions and other services are able to tie into the system later on. And when exponential growth occurs, its functionality remains unrestricted. Less errors, more development, and easy maintenance. Great.
Don’t model.
The payments team should manage the payments API. Right? Wrong. The team with the right skill sets should. Don’t model your API based on your organizational design. Model your API based on an organizational structure that supports the business needs of the company, like longevity.
Developers may come and go, but demand is here to stay. So, design your API with the user in mind. Instead of asking questions like, “What does this service need to do?” Ask questions like, “How can users apply this API to do what they need?” And engineer accordingly. Future-proof? Check. User-focused? Check.
Sounds easy enough.
But how do you figure out the way users intend to apply your API? You ask them. A client advocate will serve as the point of contact for customers leveraging your API. Think “liaison.” They know the product, receive feedback, and work to better the consumer experience. End users are heard. Customers are happy. And all the different teams are able to work in close collaboration towards a clear goal. Finally. As companies scale, creating a space dedicated solely to the needs of clients is a no-brainer.
Versions?
What’s that? You’re building an API. If it’s going to scale, it needs to be designed for longevity. There should be no version one, but only one version. Extensions? Sure. Updates? Amazing. But fundamental changes are a no-go. Build your first version as if it’s your last. It’s going to stay (more or less) the same.
User journeys and workflows.
Users don’t want to go through several consecutive API calls to finish one task. Don’t make them. Nobody likes a complicated journey. Instead, focus on your user. What do their journeys look like when they’re accomplishing tasks? How can each one be made easier? In this case, less is more. Many companies launch new endpoints like it’s a race. Maybe it is. But slow and steady always wins. Remember; impact over activity.
Who goes where, and when?
Different components of the API need to be scaled differently. Why? Because each component has different business needs. Get ahead by determining which parts of the API would serve as horizontal components and which parts as vertical. Then, assign the appropriate teams to the different components. Easy.
The horizontal teams manage the core components of the API. This means that the different products offered by the API are dealt with rapidly by vertical teams, leaving the architectural and system level concerns for the horizontal teams to handle at their own pace. Delegating the workload this way allows for a customer-first approach to development. Each team is free to work independently on their product and really focus on delivering results.
A great problem to have.
It’s one thing to start a business and another thing to scale it. The fact that a company’s API needs scaling is a victory. Don’t celebrate too early though. Without careful consideration and a calculated manner, design flaws that could cause long-term problems may arise. Figure out the best way to pursue the opportunity, then go for it.
Confused about APIs and SDKs? This guide clarifies the differences between these crucial software components, explaining their functions and when to use each.
There is often confusion between what an SDK and an API are, and why an app would want to integrate either of these third-party softwares. Many people in the tech industry have a rather vague or incorrect idea regarding APIs and SDKs, and it is common for the 2 to get mixed together. One of the main reasons for this confusion is because SDKs usually contain APIs but, not vice versa. To understand the difference in-depth, let's start by defining the two terms and later jump into their differentiation.
What is an API?
API is an acronym for Application Programming Interface. An API is a software intermediary that allows two applications to communicate with each other.
Let’s say you make a request to an API for a list of products in JSON format. Typically, the server will check if you have the proper credentials, and if you do, then gives you the list of products you requested.
In layman's terms, an API is a messenger that delivers your request to the provider that you're requesting it from and then responds to you.
An API defines the correct way for a developer to request services from an application and expose data within different contexts and across multiple channels. Therefore, a good API makes it easier to develop a program by providing the building blocks.
Why are APIs important?
- APIs help developers to add new features to their apps easily. Rather than building something from scratch, a developer can access a useful API to save them development time and ensure that key functionality works effectively.
- By using APIs, developers help reduce fragmentation across the Internet and improve navigation through an interconnected ecosystem of sites, platforms and mobile apps.
- Finally, APIs can also help stitch the internet and mobile app economy together on behalf of users. They underpin essential online behaviours – such as share buttons or social login options. Even using mapping data in e-commerce apps requires an API, and as such, APIs have become essential to users.
API Example
Ever wondered how a travel booking website can aggregate thousands of flights and destinations and show the cheapest option? Usually, the answer is to use third-party APIs to collect flight and hotel availability from providers. Similarly, if you book through one of these services, they will use the API to confirm the itinerary with the provider from them.
APIs are great for travel services because they allow machines to easily and automatically exchange data and requests (in this case, travel availability and reservation requests). Without using the API , the staff who booked the service had to manually email the airline or hotel to learn about its availability. Then, after the provider receives the email, they must confirm with the traveller. By the time the travel agent sends an email to the provider again to confirm the trip, it may no longer be available!
What is an SDK?
A Software Development Kit (SDK) is typically a set of tools that can be used to create and develop Applications. In general, an SDK refers to a full-suite software module that includes one or more libraries or APIs, processes, programming tools, sample code, documentation as well as guides that developers can use and integrate into their apps.
Although SDKs are commonly used for mobile applications, they can also be used on websites and other digital platforms too.
A common analogy that can better explain SDKs is to compare them with a model car kit. The kit contains the necessary items such as model parts, instructions, glue, and other tools needed to put them together.
Why are SDKs important?
Without an SDK, a lot of this pre-built functionality would have to be made from scratch. SDKs let you create new tools efficiently and make the process easier for anyone integrating them as everything is pre-built.
SDK Example
SDKs can be used in cases as simple as the implementation of one or more application programming interfaces (APIs) in the form of some libraries to interface to a particular programming language or to include advanced hardware that can communicate with an appropriate embedded system. SDKs are widely associated with mobile native apps, it is possible to make use of them in connection with websites and other digital platforms. A mobile SDK is used for building a mobile application for iOS and Android devices.
For example, in the majority of mobile apps, a user will need to login and most apps use oAuth logins from the likes Facebook, Twitter and Google. All three companies provide their own SDKs for mobile applications, that allows the developer to easily make the requests necessary to initiate the functionality within, in this instance to authenticate the user.
The Difference between APIs and SDKs?
An application programming interface (API) and software development kit (SDK) both play an important role in the software development process. As the name implies, an API acts as an interface between different applications or platforms and helps various software programmes interact. They usually contain specifications that can be used to interface between different software programmes. On the other hand, the SDK consists of development tools and pre-written code that developers can use to build their applications. The SDK usually reduces the workload and time required for developers to write their unique code to build software applications.
APIs may contain routines, data structures, protocols, and object class specifications to facilitate interaction between different programmes. To facilitate interaction with embedded systems, the SDK usually consists of files or APIs in the form of complex hardware. The SDK plays a vital role in eliminating work duplication and saving developers the time to create new software applications. An API usually includes a set of rules and specifications, and software programmes will follow these rules and specifications to facilitate easy interaction.
The API does not have any written sample code but includes a detailed description of function calls and function prototypes' behaviour. The SDK consists of sample programmes, technical descriptions, utility programmes, and debugging tools for programmers to integrate into the development of applications, thereby saving a lot of time and effort.
When to use SDK and when to use an API?
Developers always use the SDK when building applications. Besides, there are SDKs specifically designed for specific platforms, for example, an Android SDK would be specific to android applications. The API is used to communicate with external applications or platforms through various methods and protocols.
In short, when developing any software application, engineers will use the SDK. When you need to communicate with other third-party service providers (application/platform), you will use the API integration. Which may perhaps be part of the SDK.
Summary
To summarise, APIs act as an interface for different applications to communicate with one another, while an SDK provides a set of tools and utilities needed to develop software programmes. API only contains specifications and descriptions about functions, while SDK contains API, sample code, technical documents, tools, and utilities. The API describes the parameter types and return value types to be provided to the function, and the SDK contains libraries that can be used to develop software applications.
Learn the difference between APIs and webhooks! This guide explains how these technologies allow applications to communicate and share data, with real-world examples and use cases.
The pace at which we are able to communicate has increased 10 fold in the last two and half decades. Being able to communicate with people in different parts of the world in real-time really is something a fair few of us take for granted.
Technology has further advanced this communication by making it possible for different applications to communicate and share data amongst each other. The communication between apps has been made possible through APIs and webhooks. If you are a developer or a tech enthusiast, you have probably heard these terms multiple times, and in this case, this article probably isn’t for you.
Product and development teams alike invest heaps of time in keeping up with the latest technologies available, we are hit with “new and shiny” tools and concepts on what seems to be a daily basis, and it’s not slowing down. Deciding which to use and which not to can be tough, one thing you can be sure of though is that APIs and webhooks are here to stay.
To remove any confusion between the two and help you have a better understanding, let's break it down and look at the different technologies behind the communication of separate systems, and why they're crucial to today's world.
What is an API?
API is an acronym for Application Programming Interface. An API is a software intermediary that allows two applications to communicate with each other.
Let’s say you make a request to an API for a list of products in JSON format. Typically, the server will check if you have the proper credentials, and if you do, then gives you the list of products you requested.
In layman's terms, an API is a messenger that delivers your request to the provider that you're requesting it from and then responds to you.
An API defines the correct way for a developer to request services from an application and expose data within different contexts and across multiple channels. Therefore, a good API makes it easier to develop a program by providing the building blocks.
For instance, Facebook has an API that websites can use to get user login information as long as the user is willing to share their Facebook information with the websites. This kind of arrangement makes it easy for users to sign up on different websites on the internet without having to rewrite their login information.
Why are APIs important?
- APIs help developers to add new features to their apps easily. Rather than building something from scratch, a developer can access a useful API to save them development time and ensure that key functionality works effectively.
- By using APIs, developers help reduce fragmentation across the Internet and improve navigation through an interconnected ecosystem of sites, platforms and mobile apps.
- Finally, APIs can also help stitch the internet and mobile app economy together on behalf of users. They underpin essential online behaviours – such as share buttons or social login options. Even using mapping data in e-commerce apps requires an API, and as such, APIs have become essential to users.
Some examples of APIs include:
- Facebook Ad insights API - which helps marketers track the performance of their Ads right from their websites. This API saves time for the marketers because they don't have to always log in to their Facebook to get this data.
- Google Maps API helps other applications get access of location data that is available on Google Maps.
- Dropbox API for interacting with files stored on Dropbox. Other websites can use this API to help their users save and get files from Dropbox with ease.
What is a Webhook?
Just like an API, a webhook is also a piece of software that enables applications to share data. What makes these two different is when they send data. With an API, data has to be requested by the client whereas with a webhook, the data is automatically sent when a certain set of conditions are met.
For example, a webhook can be used to automatically send a text message to a user when they share a phone number to a website. So, with a webhook data is shared in real-time when a certain event occurs without any request being made by the application the data is being sent to.
In simple terms, a webhook broadcasts an action was taken on a server.
Why are Webhooks important?
● Webhooks are best used when an application needs to get real-time information from another website without having to make the request manually. For instance, if you need your site to constantly display the changing value of a cryptocurrency like Bitcoin, such data needs to be updated in real-time without making any requests.
● Webhooks can also be used in situations where there is no existing API to get the data that you frequently need on your website. Or even in situations when the existing API is poorly designed and may not be able to serve the purpose. The only downside with webhooks is when the source of data is offline or has a technical fault.
Common examples of Webhooks
● PayPal webhook that sends information to your accounting applications whenever one of your clients pays you.
● Shopify webhooks that provide data to apps that want to get real-time information from Shopify. Some of the information that apps may need from Shopify may include; sales, orders, etc.
● Facebook webhooks that provide other apps real-time HTTP notifications about any new events happening on the Facebook account associated with that app
Final thoughts
Both APIs and webhooks are vital for app developers, more so if the app is going to rely on data coming from multiple sources in order to operate. Without APIs and webhooks, the functionalities of most web applications on the internet would be incredibly limited. Just imagine a world without the Google Maps API. It would be extremely hard for apps to get location data.
The decision between using an API or a webhook should largely depend on whether the information needed is to be provided in real-time or on request. If the required set of data has to be sent in real-time, a webhook could potentially be a better option. But suppose the information is needed only when the user requests it. In such a case, an API would be a better alternative.
Short on time and resources but need to nail your product's UX? Learn how lean UX research can help startups validate ideas, identify user pain points, and build successful products – even on a tight budget.
It’s a common scenario we’ve seen played out amongst startups: time and resources are few, and often key stakeholders or higher-ups are adding pressure to get going on developing the product. In some cases, startups buckle and dive straight into product development, while in others, startups prioritise the value that user research brings to the user experience. Almost always, the products that launch to market and succeed are those that prioritised user research and UX. In The ROI of User Experience, Dr. Susan Weinschenk showed that, “The cost of fixing an error after development is 100 times that of fixing it before development,” and the Nielsen Norman Group proved that you could uncover up to 85% of usability problems if you test with only 5 users. Startups simply cannot ignore UX research as part of the product design process.
When timelines are short and funding limited, its easy to overlook user research in favour of dedicating those few resources to building out the product but, without user research, there’s no guarantee that users will adopt your product and that it will be successful. Lean UX research, as an agile and cost-effective option for startups, is the solution.
What is lean UX?
UX research, overall, refers to the process where product designers conduct in-depth user research in an attempt to outline user pain points and then determine the best product solutions to solve those pain points. UX research is an integral part of the user experience journey.
Lean UX may be likened to the agile methodology in that it focuses on fast-paced cycles and ongoing improvements throughout the development process, while that user research is being uncovered.
Now that we’ve outlined lean UX research a bit better, we need to jump into the various components that make up UX research that you’ll require to build out an effective UX research plan for your startup.
The building blocks of an effective lean UX research plan
It’s a common misconception that planning is a waste of resources and time. Two well-respected world leaders, Benjamin Franklin and Winston Churchill, echoed that failing to plan means that you are preparing to fail, and you’ve undoubtedly heard that sentiment shared by many other leaders. Taking time to create a plan ensures that you and your team remain focussed to the task at hand, which is conducting user research. So, what should that plan look like?
- Outlining questions: As you’ve gathered by now, there are multiple ways to conduct user research but not all of them will be worth your while. This is entirely dependent on the questions you want answered. Be strategic, thoughtful, and purpose-driven when outlining your questions so you can eliminate any research methods that won’t result in the insight you need.
- Defining your why: It’s easy to get mixed up with market research and design research. Remember that you’re looking to define specific answers that will ultimately assist you in creating a better product design for improved user experience. Focus on these only.
- Identifying research techniques: Different research methods will provide different insights into user behaviour. Take the time to highlight which methods will most likely grant you the information you’re after and build a strategy around those techniques. Lean UX research prioritises timesaving so this is an invaluable part of your plan.
- Identifying timeline resources:Using the strategy you’ve already outlined, create a timeline of your user research project. Remember to allocate team members as resources, leeway for instances where it might take more or less time to conduct the research and indicate your budget, as well as the services and software you may need to incorporate.
Start with data that’s already at your fingertips
User research is a time-consuming exercise, and if you want the data analysis to be an accurate representation of your target audience (eg. data that is valuable and useful), then it should be prioritised. The problem though, is that in-depth user research is expensive. It’s for this reason that we recommend gathering data and insights from resources that might already be available to you and then build on the process from there.
Google Analytics is the perfect place to start. This tool provides key insights into the user traffic and demographics on your website. Understanding how users interact with your website can not only help you begin the process of collecting quantitative research but assist you in forming questions for the qualitative research you aim to conduct, as well. Building profiles of your current users is also made easier as you can quickly extract information such as: interests, age, location, and devices used to build your target audience.
Another great tool to utilise is your network! While it’s important not to make any assumptions or rely too heavily on opinions that may be bias (such as opinions offered by your nuclear family or close friends), there’s no reason why you cannot conduct user research with those you already know. Bring a topic of conversation to the table in an informal setting, ask your online network to complete a survey or start a conversation on your LinkedIn profile; these are all ways to enlist the help of your network. Just consider any informal conversations, questions or chats to be preliminary.
Once you’ve exhausted the research options already available to you, you may want to expand into some other user research techniques that are commonly used by product designers when time and funding are limited.
The different types of UX research
There are numerous, additional ways to conduct data analysis, some of which are also employed by user researchers, but the focus of UX research is to determine user behaviour. How do they think? What are their needs? What motivates them to purchase a product? UX research answers these, and other questions, through observation, analysis, and feedback techniques.
The two main types of user research are qualitative and quantitative. There are many different types of qualitative and quantitative research techniques but, for the purpose of your startup, we recommend focussing on the following techniques:
Card sorting
Card sorting is an effective tool for better understanding the inner workings of your users’ minds. It’s also a fairly easy way to collect data, with minimal input required from your product designer. Simply structure an Excel spreadsheet with terms that the user is required to categorise, either by categories you have created or by creating their own. The product designer simply emails the user a copy and collects the completed forms to review.
Free surveys
Google Forms is a free, and incredibly easy, tool to make use of when you’re looking for a better understanding of your users. Ideally, your first few questions should specify demographics or other categorising data (so you can later sort the surveys) and the remainder should then be product-specific. These surveys can be sent out via any social media channel of your choosing. Bear in mind that you’ll likely receive some opinions from non-users, so those initial questions within the survey template should be used to distinguish between information that is useful to the product development process, and that which needs to be removed from the pool.
One-on-one interviews
One-on-one interviews can be time-consuming, but it isn’t necessary to dedicate all your time to this technique, only some of it. Interviews grant insight into how users navigate your product, and how they feel about it. We recommend a guerrilla approach to one-on-one interviews. Instead of setting up standardised testing in a formal environment, head out to a public space and ask a few people to answer some questions. This is a great way to get unbiased, honest feedback; just remember to try and compensate participants for their time!
Usability Tests
We mentioned at the start of this article that you could uncover up to 85% of usability problems if you test with only 5 users. Usability tests give high-value insight to how users perceive and engage with your product in the early stages, so even if you cannot dedicate a lot of time to walking through tests with individual users, you should spend time doing at least 5 usability tests. Invite a few users to participate and sit in on the walkthrough as they experience your product; it costs nothing but some time and ensures valuable insight into the functionalities and features of your product.
Heuristic Evaluations
Heuristic evaluations are the usability tests of software; they assist in identifying any usability problems so that your development team can make corrections or fixes early on. While this technique is broadly considered as simple, judging the product interface against the compliance criteria laid out by the Nielsen Norman Group offers valuable insight that can be used to enhance your product and/or gain an advantage over your competitors.
Concept Testing
There are various methods of conducting concept testing. It isn’t important how you do it, just that you make sure that you do incorporate it into your research process. The purpose of concept testing is to determine whether the idea behind your product will meet the needs of your target audience, and testing the value proposition of the product can be achieved by proving the concept one-on-one, or through large group interviews. By way of example, the product design teams at Blott Studio like to iterate on each other’s concepts to determine the best possible prototype.
Incorporating the above combination of qualitative and quantitative research techniques is not only the most cost-effective way to conduct lean UX research for your startup, but also caters for more limited resources, while still ensuring that due consideration has been given to the research process.
How to share your findings with your team and implement effective UX design
The key to quality user research is summarising it in an organised way so that your team can easily interpret the information and draw key findings, assumptions, and validations from your report. Unorganised, raw data is not usable nor actionable.
In the case of providing insights to your UX product design team, you’ll specifically want to provide your findings in a way that is easy to interpret so your team can extract the relevant information. We recommend using a tool such as Excel spreadsheets or Google Docs. The idea is to tabulate all your research and aim to colour-code and organise the information for your UX product design team. This ensures that, at a glance, they can determine what pain points your research has highlighted and work to solve those pain points.
Once you’ve shared your findings with your team, those insights can be interpreted and used to improve on your product design for a more effective, and quality, user experience.
To summarise
As a startup with restricted resources, its easy to get caught up in the excitement of creating something new. But, getting started on the product design without prioritising user research (regardless of how limited resources may be) means dismissing the user that will translate to a poor user experience and, ultimately, an abandoned product. Implement this easy process to create an effective lean UX research plan by giving thought to the research you need, reviewing the tools you already have access to, dedicating time to a few additional cost-effective research methods, and then compiling your research into a thorough report your product team can utilise.
Lean UX research incorporates the best components of effective user research methodology with an agile approach so you, as a startup, can maximise on available resources and gain thorough insights that convert into a user-centric product with the highest probability for success.
Discover how Machine Learning can revolutionize your Fintech. Improve customer service, security, and efficiency with AI-powered solutions.
The banking industry has undoubtedly gone through a revolutionary process over the course of the past few years, with a significant rise in digital banking solutions since COVID-19. On the back of this revolution, many financial service providers have sought out tools and techniques to provide an improved user experience through their Fintech offerings, not unlike Machine Learning. Machine Learning has made Fintechs more efficient by improving internal processes and procedures, offering a better understanding of customers by analysing their behaviour, and reducing operational costs – to name just a few.
It's no surprise then that so many Fintechs employ Machine Learning techniques to improve their products and gain a greater market share. With all the excitement of recent Artificial Intelligence advancements, we wanted to take a step back and share how Machine Learning still deserves your consideration for improved efficiency, so let’s dive right in!
Machine Learning vs Artificial Intelligence
The first step in determining the right Machine Learning opportunities for your Fintech is to identify how Machine Learning differs from Artificial Intelligence. Consider that Artificial Intelligence is an over-arching technology, under which Machine Learning (and Deep Learning) can be found; Machine Learning is thus a small part of Artificial Intelligence.
To delve into the specifics, Machine Learning relies on specific pre-programming that determines which outputs it should compute, based on previously inputted historical data. Its strength lies in making predictions about this historical data, though it cannot provide feedback outside of its’ specific programming. It incorporates significant amounts of structured and semi-structured data to be as accurate and effective as possible, however, without inputting additional data points, it will not provide outputs outside of its scope.
On the other hand, Artificial Intelligence refers to a computer-based system that relies on algorithms to learn or process information in a similar way to human intelligence. Where Machine Learning can provide information based on the set number of formulae it has been given, Artificial Intelligence is able to make its own assumptions and draw conclusions. To find out more about Artificial Intelligence specifically, read our blog: What is Artificial Intelligence (AI)?
In many instances, Artificial Intelligence applications incorporate Machine Learning programmes to enhance the AI’s capabilities. Understanding this correlation provides better clarity into just how beneficial Machine Learning can be for your Fintech. Here are some ways you could make your Fintech more efficient, through Machine Learning:
Improved Customer Service
Excellent customer service is an expectation of customer relations, regardless of industry, though the uptick in digital banking solutions has seen an even greater demand for improved customer service experiences in Fintech. Machine Learning can assist you with this goal in two ways:
- Chatbots: In a world of instant gratification, responding to customers’ queries and complaints (or compliments) is a high priority. Chatbots provide a painless, swift solution to responding to customers instantaneously. Chatbots also not only provide feedback to customers when they need it, but they also gather valuable insights for Fintechs to analyse and improve their offerings.
- Personalisation: Machine Learning algorithms can also be implemented to collect customer data, analyse that data, and then tweak the user experience for a more personalised interaction. This offers more tailored solutions to different users, promoting brand loyalty and continued product use.
Protection Against Fraud
Fraud is commonly associated with the banking industry, and now that so much of the industry has gone digital, threats are even more prominent. Hackers are amongst the biggest threats to digital products, as they look to exploit weaknesses. Machine Learning cannot necessarily eradicate these threats, but it can certainly assist Fintech’s security measures against them.
- Data analytics: Analysing user information goes further than just assessing behaviours for improved services, it also assists in fraud detection. Machine Learning algorithms can collect data points such as location, the device used and more, to assist in these efforts and isolate any concerns.
- Attack detection: With Machine Learning’s ability to process large quantities of data, it’s no surprise that its algorithms are fully capable of detecting fraud attempts more accurately than humans. Not only this, but they can also alert to potential threats, as well.
- Regulatory compliance support: Another great benefit of Machine Learning, thanks to its capabilities mentioned above, is the timeliness it lends Fintech owners when it comes to keeping policies up to date, which is valuable for maintaining regulatory compliance.
Minimised Loan Approval Risk
Whether peer-to-peer or traditional institutions, lending is an area of the banking industry that has often been shrouded in risk. Machine Learning algorithms can mitigate risks associated with loans:
- Credit-risk models: Algorithms analyse borrower data against other lenders to determine if that borrower would be able to pay back the loan. As previously mentioned, this ML would make use of historical data and data points related to repayments to effectively assess the success rate of loan repayments. Borrowers who do not automatically meet the requirements are referred to customer service advisors.
Credit-risk models greatly minimise the time and resources needed to determine loan safety, though some argue that historical data may be outdated in this instance.
Refined Forecasting
While traders and investors are highly skilled in their craft and able to deduce effectively, to some degree, the ever-moving needle of stock market forecasts, Machine Learning algorithms are unparalleled.
- Stock market forecasting metrics: Machine Learning algorithms are capable of processing vast amounts of historical data while simultaneously monitoring data in real-time. This combination makes their computer processing capability highly accurate and effective with live updates that can be pushed to traders and investors for improved strategy.
Effective Financial Planning Assistance
Effective financial planning assistance is a sought-after financial service, particularly in the field of investing. Machine Learning provides Fintechs with a competitive advantage using robo-advisors.
- Robo-advisors: robo-advisors are ML-driven solutions that analyse consumer data found in surveys (or through automated algorithms) to assess their financial status and make informed investment decisions based thereon, that are in the consumer’s best interests.
Robo-advisors are still developing, and many have argued on the ethics and accuracy behind survey-focussed robo-advisors, though newer models have proven to be more accurate as they assess, not only income but investment habits and level of risk aversion, too.
The Right ML Solution for Your Fintech
The key thing to consider when determining the right Machine Learning tool for your Fintech is not to incorporate multiple solutions all at once, but to rather decide on specific models or algorithms that will further support your unique selling point. If your USP is risk aversion, you’ll want to consider a Machine Learning model that detects fraud. If your core values are set on user experience, you may want to enable an algorithm that personalises their experience or incorporate a chatbot for fast, effective communication.
While Artificial Intelligence continues to make waves across the Fintech sphere, within the creative industry, and the general tech space, Machine Learning still has its place and is highly beneficial for Fintechs looking to make cost- and resource-savings across a range of procedures and functions, that will ultimately result in improved user experience. If you’d like to find out more about Blott Studio’s Machine Learning products, schedule a chat with our experts!
UX researchers: Avoid these 15 cognitive biases in your research process! Confirmation bias, anchoring bias, and more explained. Improve your UX research quality today.
Cognitive biases impact our day-to-day on a personal level, but within our work environments, as well. They effect our judgement of others and can impact perceptions. This is detrimental to UX design and UX research that needs to be valuable and accurate to deliver on successful user-centric projects. Understanding cognitive biases and how to avoid them throughout your UX research process is imperative if you’re aiming to deliver a high-quality finished product to your clients.
In this article, I’ll highlight 15 cognitive biases UX researchers regularly come across. Read on for 15 types of cognitive bias to avoid in UX research:
Confirmation Bias
Confirmation bias refers to the tendency UX researchers can have to interpret data so that it specifically supports their pre-existing beliefs, effectively ignoring other valuable insights. This is also the most common type of bias in research. The problem this presents is that the final product is not designed with the end user in mind. Researchers need to be careful not to discount data that doesn’t support their assumptions, but to review all data equally.
False-Consensus Bias
False-consensus bias has to do with a researcher viewing their own opinions and behaviours as normal, or common-place, and the opinions and behaviours of others as abnormal. This is similar to confirmation bias in the sense that researchers make assumptions about end users and do not conduct thorough research to support their final design. In some cases, UX researchers with false-consensus bias may not even conduct research as they assume their end users share their outlooks. It’s important for UX researchers to follow due process and conduct thorough research irrespective of their own opinions and behaviours.
Recency Bias
Recency bias is when UX researchers place more value on events that have occurred in recent times, over those that are historic. Because it’s easier to remember our most recent experiences these UX researchers will place more value on their most recent encounters and rely more heavily on them when making design decisions. The easiest way to overcome this type of bias is to ensure that detailed notes are taken of every interview or interaction, for easy reference later on in the research process.
Primacy Bias
Primacy bias effectively translates to “first impressions count”. In some cases, UX researchers tend to remember the first, or most memorable, impressions in the interview stage, and disregard all other encounters. As with recency bias, it is important to keep details notes of every interview so that the data can be thoroughly reviewed, and primacy bias can be avoided.
Anchoring Bias
Referred to as “anchors”, anchoring bias is a cognitive bias wherein individuals tend to rely on their first impressions and make comparisons with new information to that original experience. For example, when conducting research, the UX designer comes across app subscription A at a high price point. So, when the next app subscription, subscription B, is brought up at a lower price point, the UX designer will “anchor” subscription A as the relevant source of information and determine that subscription B is cheap and possibly lower quality. UX researchers can avoid this type of cognitive bias by referencing multiple sources when making key decisions.
Peak-End Bias
Peak-end bias references the tendency UX researchers have to value highly emotional moments, or “peaks”, as well as the last moments, or “end”, of any interaction or experience. To this end, researchers who experience a positive “peak” and a positive “end” will view the overall experience as positive (and the opposite is true if the “peak” and “end” were deemed negative). While it is difficult to reframe intense emotional moments, thorough note-taking can assist when revisiting these interactions.
Implicit Bias
Also known as unconscious bias, implicit bias is our tendency to have a collection of attitudes or preconceived stereotypes which we associate with people unintentionally. In UX research, this can affect research, as UX researchers may conduct interviews within limited parameters, based on demographics or geographics (as an example). It’s important to determine parameters prior to setting out to collect research, as UX researchers can take the time to determine inclusive parameters.
Hindsight Bias
Having hindsight bias refers to some UX researchers’ tendency to overestimate just how predictable events that have happened in the past were. This further influences these UX researchers into believing that they can accurately depict future outcomes, based on this same notion. UX researchers can avoid this cognitive bias by framing interview questions that bring to light more of the individuals past behaviour (that is objective) or avoid framing interview questions that put individuals in the hindsight mindset.
Social Desirability Bias
Social desirability bias links to our innate need as humans to feel accepted within our community. Because of this, social desirability as a cognitive bias refers to the tendency interviewees have in answering their questions in a way that makes them fit in or be looked upon favourably. This results in skewed data that can mislead the design team. It is therefore imperative to communicate the confidentiality of interviewees’ answers and to request honesty throughout the interview process, or else conduct observational research instead.
Serial Position Bias
Serial position bias refers to UX researchers’ ability to recall the beginning or end of a list or sequence more accurately than that of the middle. For example, in a list of features a designer is more likely to remember those listed closer to the top or the bottom and forget, or struggle to remember, those listed in the middle. One way to overcome serial position bias is to reference key information at the beginning and the end of the interview, or the user interface experience.
Clustering Illusion Bias
Clustering illusion bias refers to the tendency UX researchers can have in analysing data and isolating false patterns that aren’t there. In some instances, random data may present in coincidental groups, such as having occurred in a short time frame, and UX researchers will see this information as trend clusters. One way to avoid clustering illusions is to ensure your interview questions are not leading. Another is to incorporate quantitative data sources into your UX research.
Framing Bias
Framing bias has to do with the tendency people may have to interpret information in a certain way, dependent on how that information is displayed (and not based on the facts presented). UX researchers may reach different conclusions, and make certain judgements, if that same information is presented to them in two different ways. Avoid this cognitive bias by reframing your interview questions to be neutral and open-ended.
Sunk-Cost Bias
Sunk-cost bias refers to the tendency of continuing with a task or project when time, effort, and other resources (such as funding) have been invested, irrespective of whether the cost of that task or project outweighs the benefits gained. By way of example, a UX product design team may utilise resources to build out a feature prototype only to discover that that feature does not provide value to the users. Instead of abandoning the feature, the product design team pushes forward with it. While these situations are never great to realise, the important thing is to not waste any further resources on the item.
Transparency Bias
Transparency bias is the tendency to overestimate how well people understand other people’s internal thoughts and feelings, as well as how well other people understand their own internal thoughts and feelings. The trouble with the illusion of transparency is that it can lead to miscommunication. In the instance of conducting interviews, participants may overestimate how much interviewers are able to glean from their body language and thus do not feel the need to clarify their answers. UX researchers need to incorporate affirmative feedback and take time to clarify any points throughout the interview process for in-depth insights.
Fundamental Attribution Bias
Fundamental attribution bias is when an individual tends to attribute another individual’s decision-making and actions to their character, while they attribute their own behaviours to external factors that they deem are out of their control. Not knowing an individual’s situation and how that may impact their behaviour is what leads us to make judgements of that individual. UX researchers can reframe their own judgements in these scenarios by considering their own actions whenever they have been in a similar situation to better understand and appreciate their interviewee’s response to any given question.
Understanding these 15 types of cognitive biases avoid in UX research will help you to identify and avoid them to ensure your UX research remains unbiased and of value. Preparation is always key. At the end of the day, the most important thing is to deliver a high-performing product to your clients, and cognitive biases are just one element of UX research that could impact on delivering a quality result.
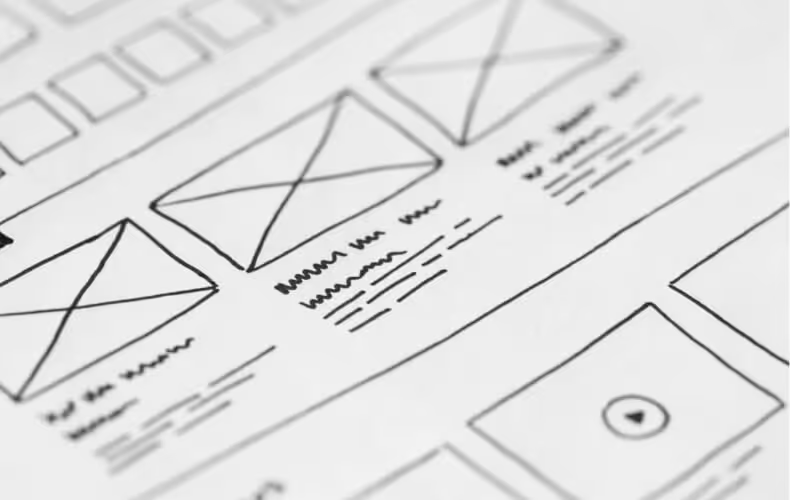
15 Types of Cognitive Bias to Avoid in UX Research
UX researchers: Avoid these 15 cognitive biases in your research process! Confirmation bias, anchoring bias, and more explained. Improve your UX research quality today.
Browse all
Maximising Your R&D Tax Credits in the UK: Why a Software Development Agency is Your Best Ally
Discover how partnering with a software development agency can help maximise your R&D tax credits in the UK. Learn about upcoming legislative changes and tips for optimising your claim.
Cybersecurity 101: Protecting Your Digital Ecosystem in the Age of DORA
Learn the basics of cybersecurity and why they are critical in today's digital age. Explore how mastering the fundamentals supports compliance with DORA and strengthens your organisation's resilience against cyber threats.
The Ultimate Guide to Fintech Security: Prioritising What Matters Most
Discover the ultimate guide to fintech security. Explore key strategies to protect data, prevent fraud, and comply with regulations, ranked in order of importance to help your fintech thrive securely.
That “Aha” Moment
What’s that? Some call it the “eureka” moment. Some, the “wow” moment. But it’s all in the name; the “aha” moment is when a user thinks, “Aha! I get it.”
21 Questions: Usability Style
As much as we’d love to think that our users are a lot like us, they rarely are. That’s why it’s important that we understand who they are, what they need, and how they go about getting it. Our products are designed for them.
Get Your (tech) Priorities Straight
Startups fail from time to time. Not because of a lack of good ideas, but because the wrong idea’s been chosen.
Product Feedback: What's The Best Way To Get It?
Why are people using one feature three times more than another? Why are they falling off the wagon on the last step? Why are customers using your product less frequently than before?
Start Strong With A Discovery Session
Brainstorming session, discovery group, team meeting; whatever. Every project should start with a group discussion- client included.
Let's Talk About Agile Development
The dictionary (well, Google) describes the term “Agile” as “able to move quickly and easily.” That’s true, even in the context of tech. But it’s also more than that.